CMCI: A Robust Multimodal Fusion Method for Spiking Neural Networks
NEURAL INFORMATION PROCESSING, ICONIP 2023, PT III(2024)
摘要
Human understand the external world through a variety of perceptual processes such as sight, sound, touch and smell. Simulating such biological multi-sensory fusion decisions using a computational model is important for both computer and neuroscience research. Spiking Neural Networks (SNNs) mimic the neural dynamics of the brain, which are expected to reveal the biological multimodal perception mechanism. However, existing works of multimodal SNNs are still limited, and most of them only focus on audiovisual fusion and lack systematic comparison of the performance and robustness of the models. In this paper, we propose a novel fusion module called Cross-modality Current Integration (CMCI) for multimodal SNNs and systematically compare it with other fusion methods on visual, auditory and olfactory fusion recognition tasks. Besides, a regularization technique called Modality-wise Dropout (Mod-Drop) is introduced to further improve the robustness of multimodal SNNs in missing modalities. Experimental results show that our method exhibits superiority in both modality-complete and missing conditions without any additional networks or parameters.
更多查看译文
关键词
spiking neural networks,multimodal learning,missing modality
AI 理解论文
溯源树
样例
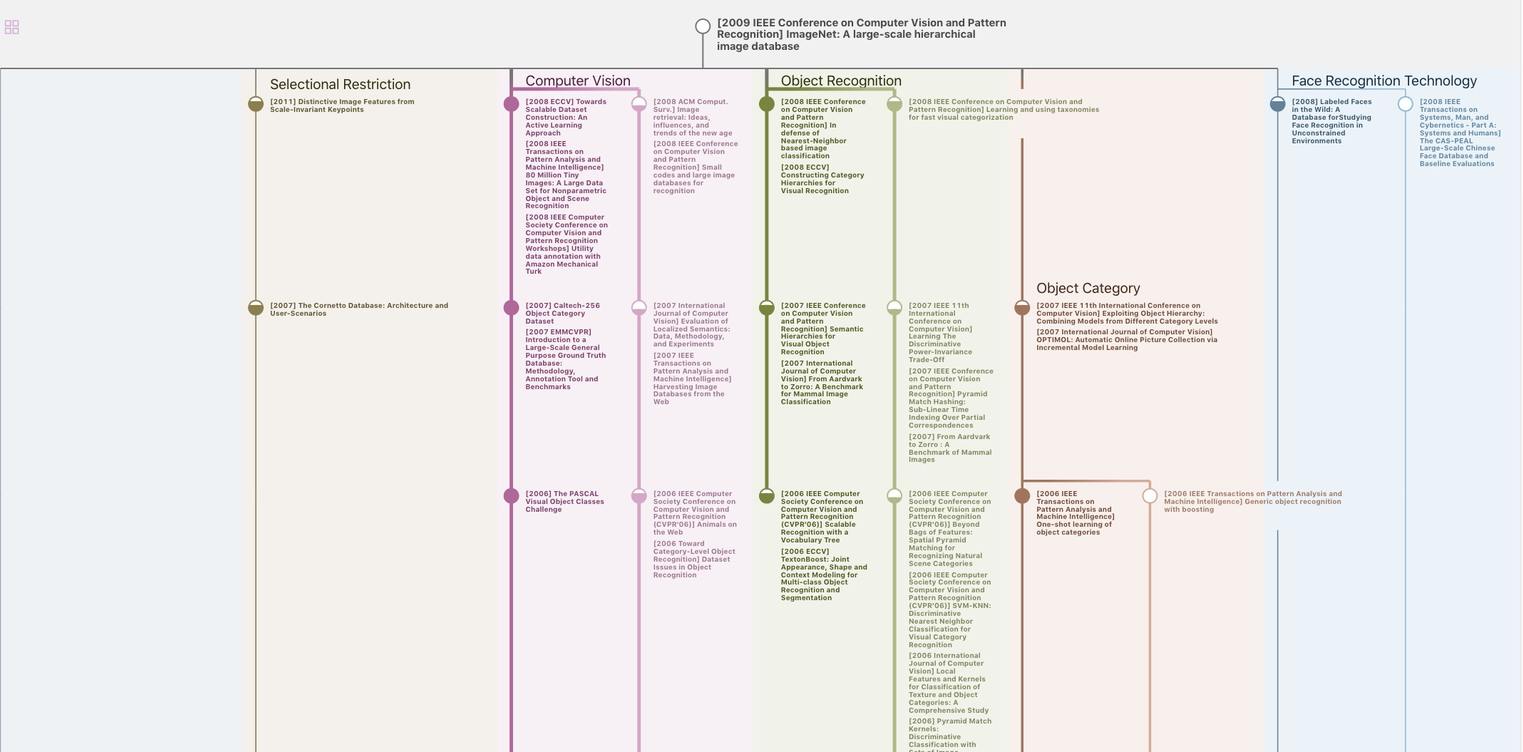
生成溯源树,研究论文发展脉络
Chat Paper
正在生成论文摘要