Healthcare Sustainability: Hospitalization Rate Forecasting with Transfer Learning and Location-Aware News Analysis
SUSTAINABILITY(2023)
摘要
Monitoring and forecasting hospitalization rates are of essential significance to public health systems in understanding and managing overall healthcare deliveries and strategizing long-term sustainability. Early-stage prediction of hospitalization rates is crucial to meet the medical needs of numerous patients during emerging epidemic diseases such as COVID-19. Nevertheless, this is a challenging task due to insufficient data and experience. In addition, relevant existing work neglects or fails to exploit the extensive contribution of external factors such as news, policies, and geolocations. In this paper, we demonstrate the significant relationship between hospitalization rates and COVID-19 infection cases. We then adapt a transfer learning architecture with dynamic location-aware sentiment and semantic analysis (TLSS) to a new application scenario: hospitalization rate prediction during COVID-19. This architecture learns and transfers general transmission patterns of existing epidemic diseases to predict hospitalization rates during COVID-19. We combine the learned knowledge with time series features and news sentiment and semantic features in a dynamic propagation process. We conduct extensive experiments to compare the proposed approach with several state-of-the-art machine learning methods with different lead times of ground truth. Our results show that TLSS exhibits outstanding predictive performance for hospitalization rates. Thus, it provides advanced artificial intelligence (AI) techniques for supporting decision-making in healthcare sustainability.
更多查看译文
关键词
sustainability,hospitalization,healthcare,COVID-19,time series forecasting,transfer learning,sentiment,semantic analysis
AI 理解论文
溯源树
样例
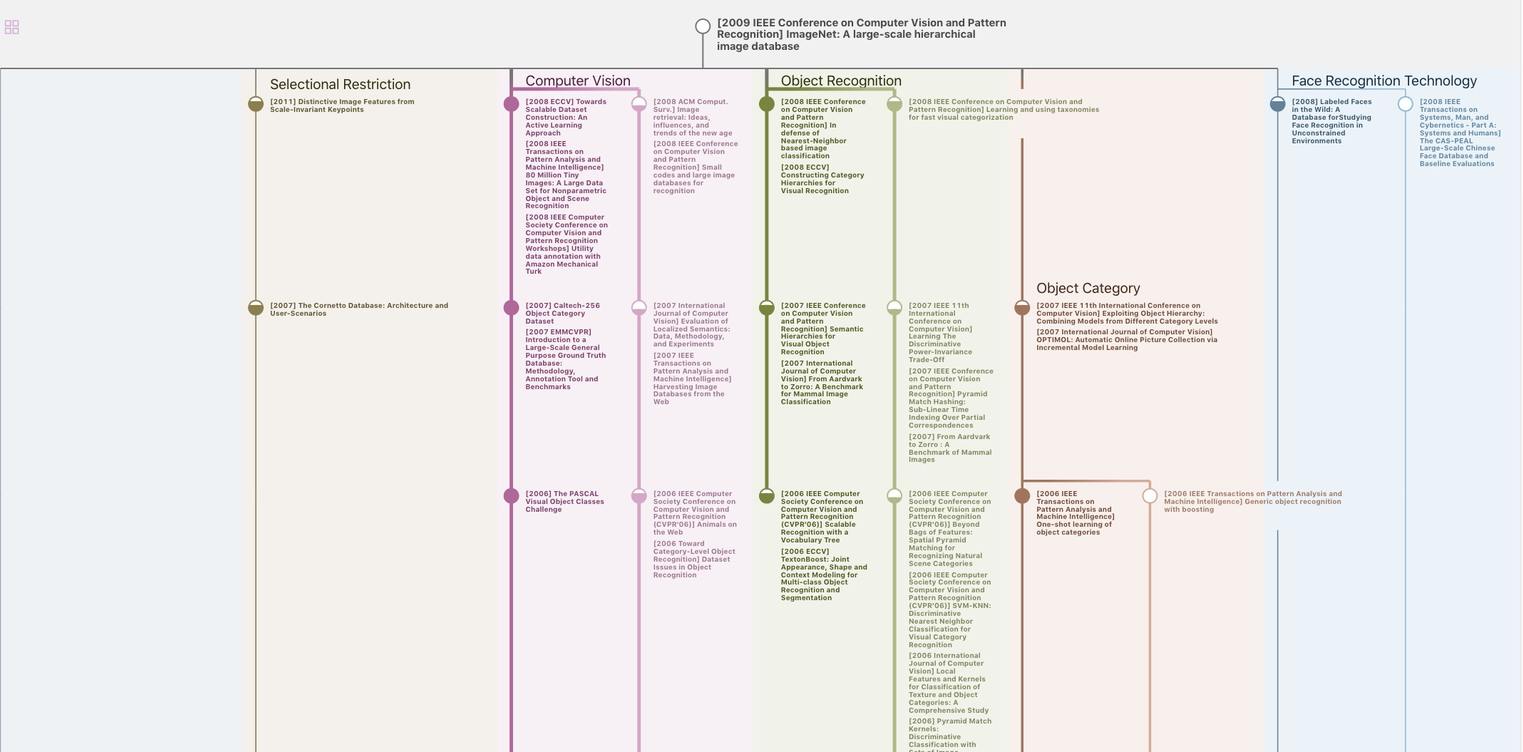
生成溯源树,研究论文发展脉络
Chat Paper
正在生成论文摘要