Reinforcement learning for scaffold-free construction of spanning structures
8TH ACM SYMPOSIUM ON COMPUTATIONAL FABRICATION, SCF 2023(2023)
摘要
In construction robotics, a conventional design-to-fabrication workflow starts with designing a structure, followed by task and robotic motion planning, and ultimately, fabrication. However, this approach can prove unsuccessful, as we may only discover the infeasibility of a design at the final stages of the process. This can result in rework and a considerable waste of time and resources. To overcome this challenge, we propose a design method based on reinforcement learning (RL) where the agent makes decisions at every step of the sequential assembly of the structure while considering assembly's stability. In this way, we take the construction constraints into consideration at the design stage. The research particularly focuses on the design of spanning structures that multiple robot arms can construct without the need for scaffolding. A series of experiments were conducted using both a centralized and a decentralized learning setup. Our results show that while the decentralized setup was successful in constructing smaller structures, only the centralized setup allowed active collaboration between robot arms, resulting in structures with larger spans. To validate our approach, we fabricated two of the designed structures with two collaborating robot arms, which confirmed the feasibility of these designs. In summary, the proposed method opens exciting possibilities for generating innovative designs that push the boundaries of architectural creativity while simultaneously fulfilling fabrication-related constraints.
更多查看译文
关键词
multi-agent reinforcement learning,structured networks,centralized training,soft actor critic,robotic fabrication
AI 理解论文
溯源树
样例
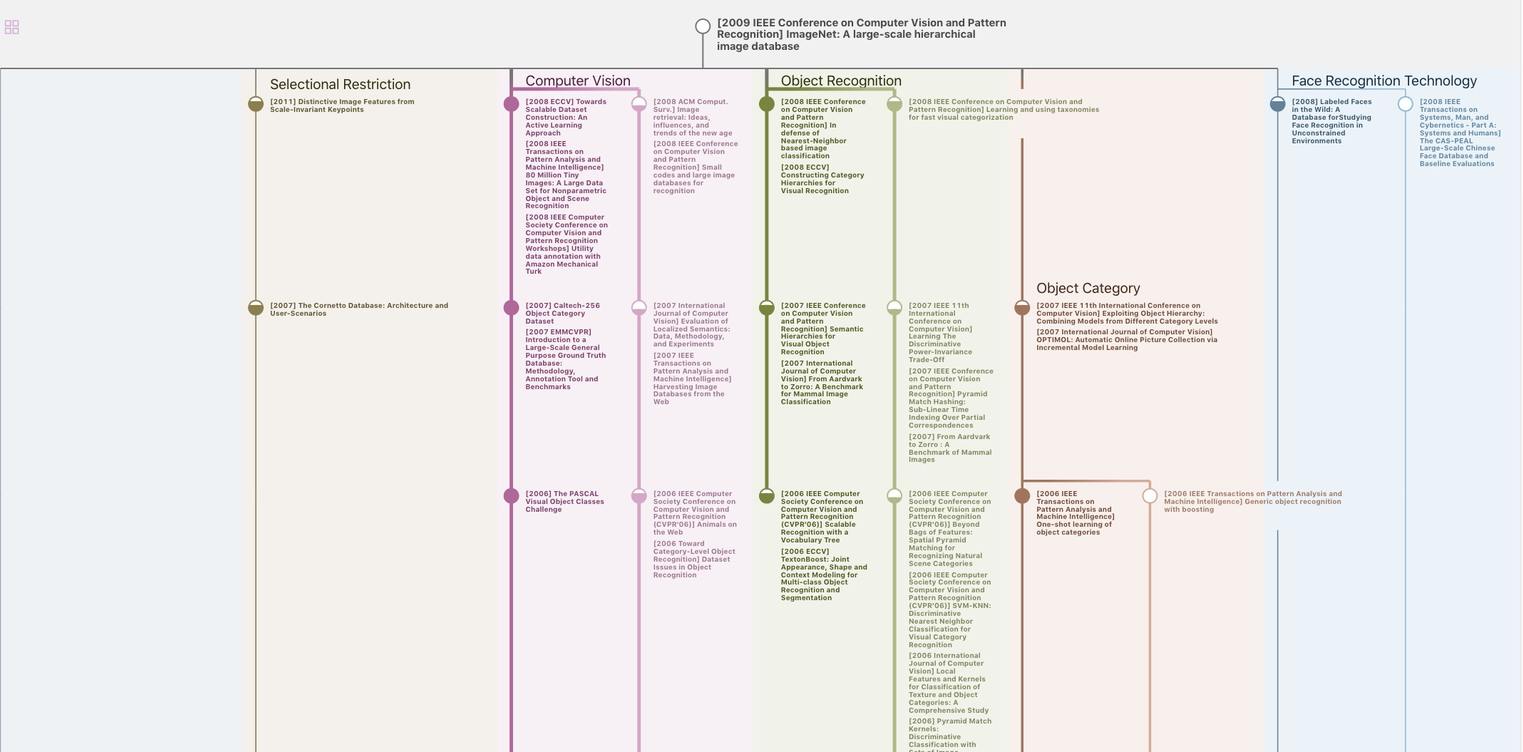
生成溯源树,研究论文发展脉络
Chat Paper
正在生成论文摘要