Correlational analyses of biomarkers that are harmonized through a bridging study due to measurement errors
STATISTICAL METHODS IN MEDICAL RESEARCH(2024)
摘要
Evaluating correlations between disease biomarkers and clinical outcomes is crucial in biomedical research. During the early stages of many chronic diseases, changes in biomarkers and clinical outcomes are often subtle. A major challenge to detecting subtle correlations is that studies with large sample sizes are usually needed to achieve sufficient statistical power. This challenge is even greater when biofluid and imaging biomarker data are used because the required procedures are burdensome, perceived as invasive, and/or expensive, limiting sample sizes in individual studies. Combining data across multiple studies may increase statistical power, but biomarker data may be generated using different assay platforms, scanner types, or processing protocols, which may affect measured biomarker values. Therefore, harmonizing biomarker data is essential to combining data across studies. Bridging studies involve re-processing of a subset of samples or imaging scans to evaluate how biomarker values vary by studies. This presents an analytic challenge on how to best harmonize biomarker data across studies to allow unbiased and optimal estimates of their correlations with standardized clinical outcomes. We conceptualize that a latent biomarker underlies the observed biomarkers across studies, and propose a novel approach that integrates the data in the bridging study with the study-specific biomarker data for estimating the biological correlations between biomarkers and clinical outcomes. Through extensive simulations, we compare our method to several alternative methods/algorithms often used to estimate the correlations. Finally, we demonstrate the application of this methodology to a real-world multi-center Alzheimer's disease biomarker study to correlate cerebrospinal fluid biomarker concentrations with cognitive outcomes.
更多查看译文
关键词
Alzheimer's disease,bridging cohort,correlation,Fisher transformation,harmonization,intra-class correlation,latent variable
AI 理解论文
溯源树
样例
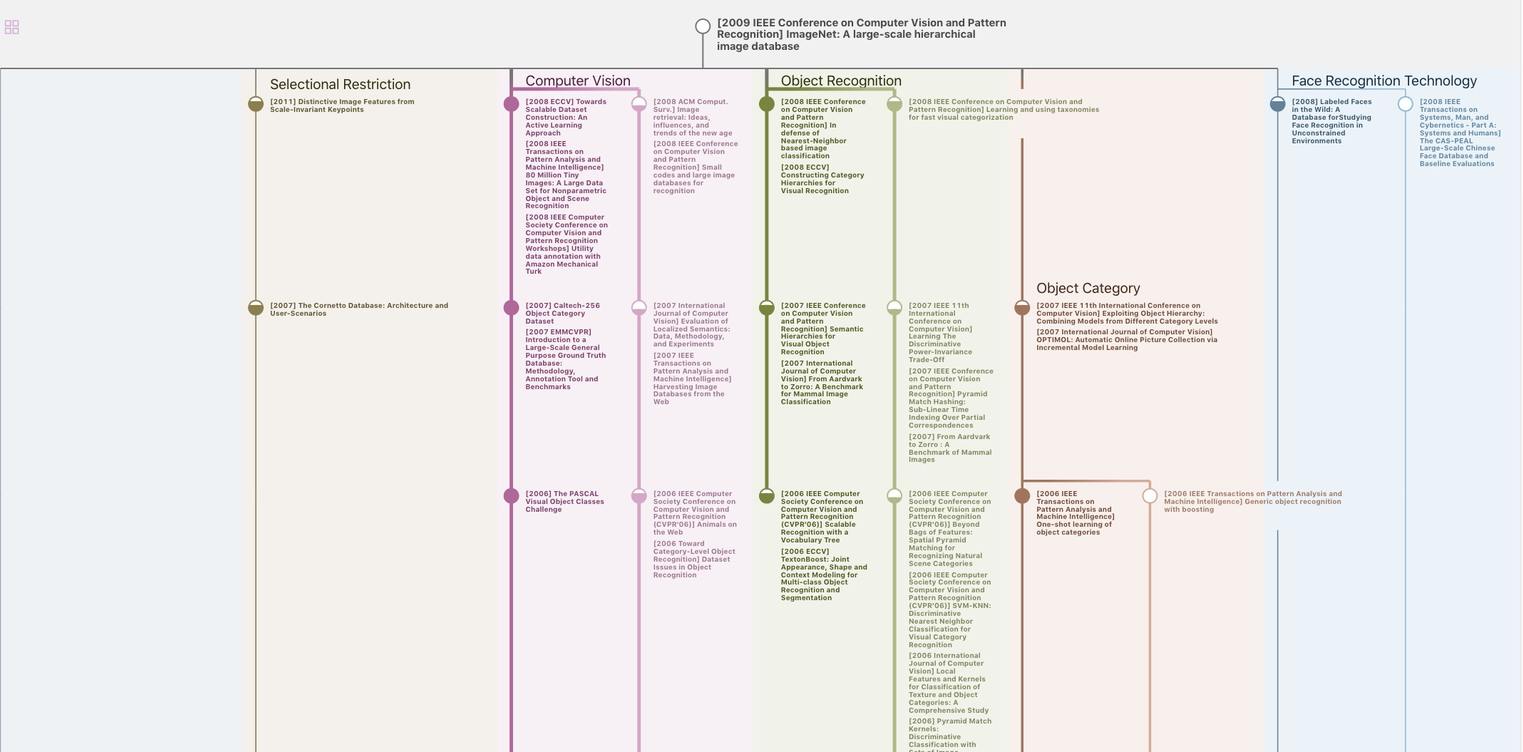
生成溯源树,研究论文发展脉络
Chat Paper
正在生成论文摘要