Online Confirmation-Augmented Probabilistic Topic Modeling in Cyber-Physical Social Infrastructure Systems
PROCEEDINGS OF THE 10TH ACM INTERNATIONAL CONFERENCE ON SYSTEMS FOR ENERGY-EFFICIENT BUILDINGS, CITIES, AND TRANSPORTATION, BUILDSYS 2023(2023)
摘要
Online probabilistic topic models serve as essential analytical tools within Cyber-Physical Social Infrastructure Systems (CPSIS), enabling the analysis of real-time data streams. These models empower operators and decision-makers with actionable insights, anomaly detection, predictions, optimized resource allocation, user engagement, and social feedback, all critical for responding to evolving CPSIS conditions. While these models use inferred topic-assignment distributions to create lower-dimensional representations, applying them to online user-generated streams, like social media and community apps, has historically posed challenges due to sparse relevant content, leading to suboptimal performance. Our study proposes a novel and expanded version of topic models that integrates the variational lower bound with a linear reward function, supervised by a label associated with the confidence of relevant content presence. We introduce a learning algorithm designed for these augmented topic models. Our empirical experiments, conducted on real-world datasets, provide compelling evidence that our approach uniquely enhances the potential of any topic model in CPSIS for downstream tasks in information management. These enhancements encompass improved topic interpretability, enhanced data labeling precision, and the refinement of similarity metrics, reinforcing the effectiveness of our online confirmation-augmented probabilistic topic modeling approach in processing and analyzing CPSIS real-time data streams.
更多查看译文
关键词
information augmentation,online machine learning,topic models,variational bayes
AI 理解论文
溯源树
样例
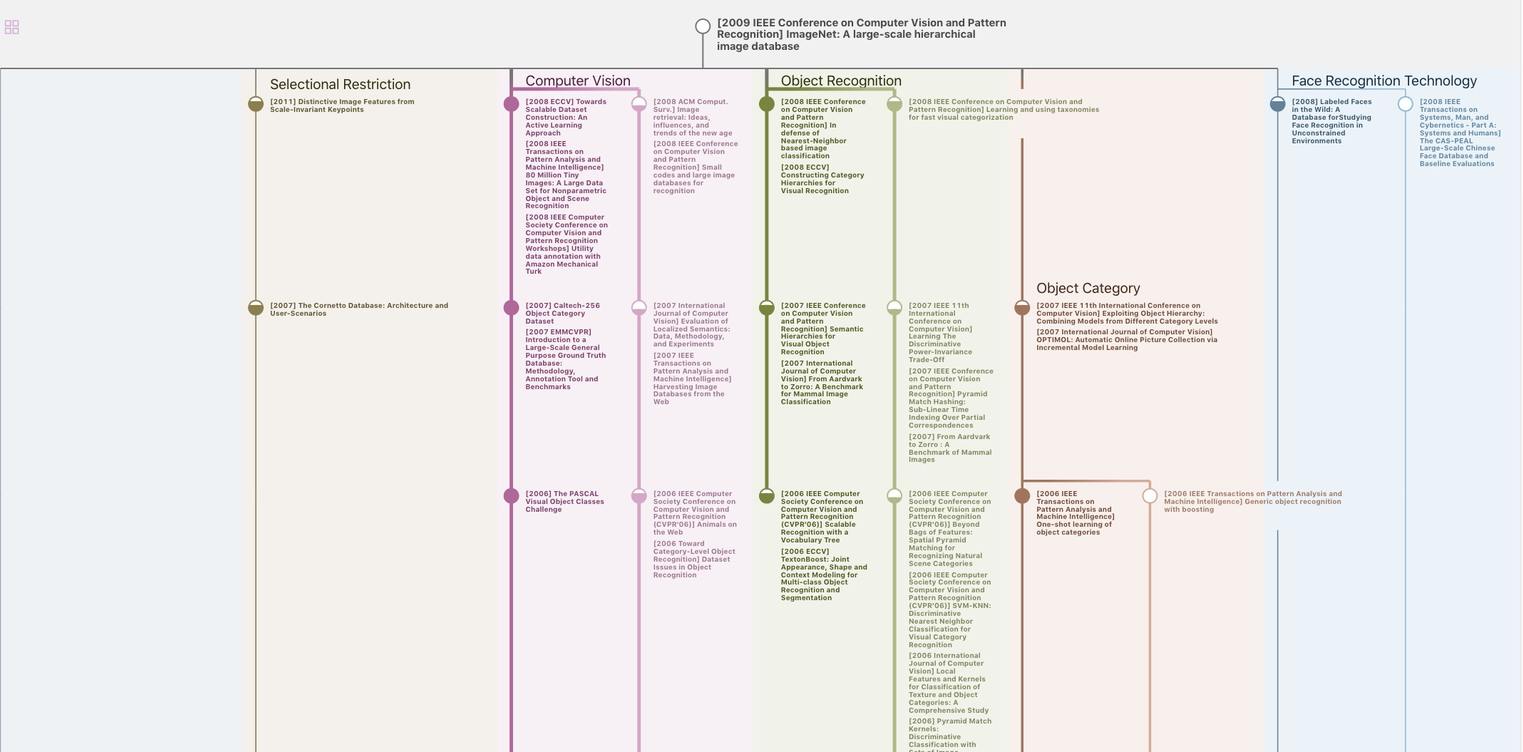
生成溯源树,研究论文发展脉络
Chat Paper
正在生成论文摘要