IFGNN: An Individual Fairness Awareness Model for Missing Sensitive Information Graphs
DATABASES THEORY AND APPLICATIONS, ADC 2023(2024)
摘要
Graph neural networks (GNNs) provide an approach for analyzing complicated graph data for node, edge, and graph-level prediction tasks. However, due to societal discrimination in real-world applications, the labels in datasets may have certain biases. This bias is magnified as GNNs iteratively obtain information from neighbourhoods through message passing and aggregation, generating unfair embeddings that implicitly affect the prediction results. In real-world datasets, missing sensitive attributes is common due to incomplete data collection and privacy concerns. However, research on the fairness of GNNs in incomplete graph data is limited and mainly focuses on group fairness. Addressing individual unfairness in GNNs when the sensitive attributes are missing remains unexplored. To solve this novel problem, we introduce a model named IFGNN, which leverages a GNN-based encoder and a decoder to generate node embeddings. Additionally, IFGNN adopts the Lipschitz condition to ensure individual fairness. Through comprehensive experiments on four real-world datasets compared with baseline models in node classification tasks, the results demonstrate that IFGNN can achieve individual fairness while maintaining high prediction accuracy.
更多查看译文
关键词
Individual fairness,Sensitive attribute,GNN
AI 理解论文
溯源树
样例
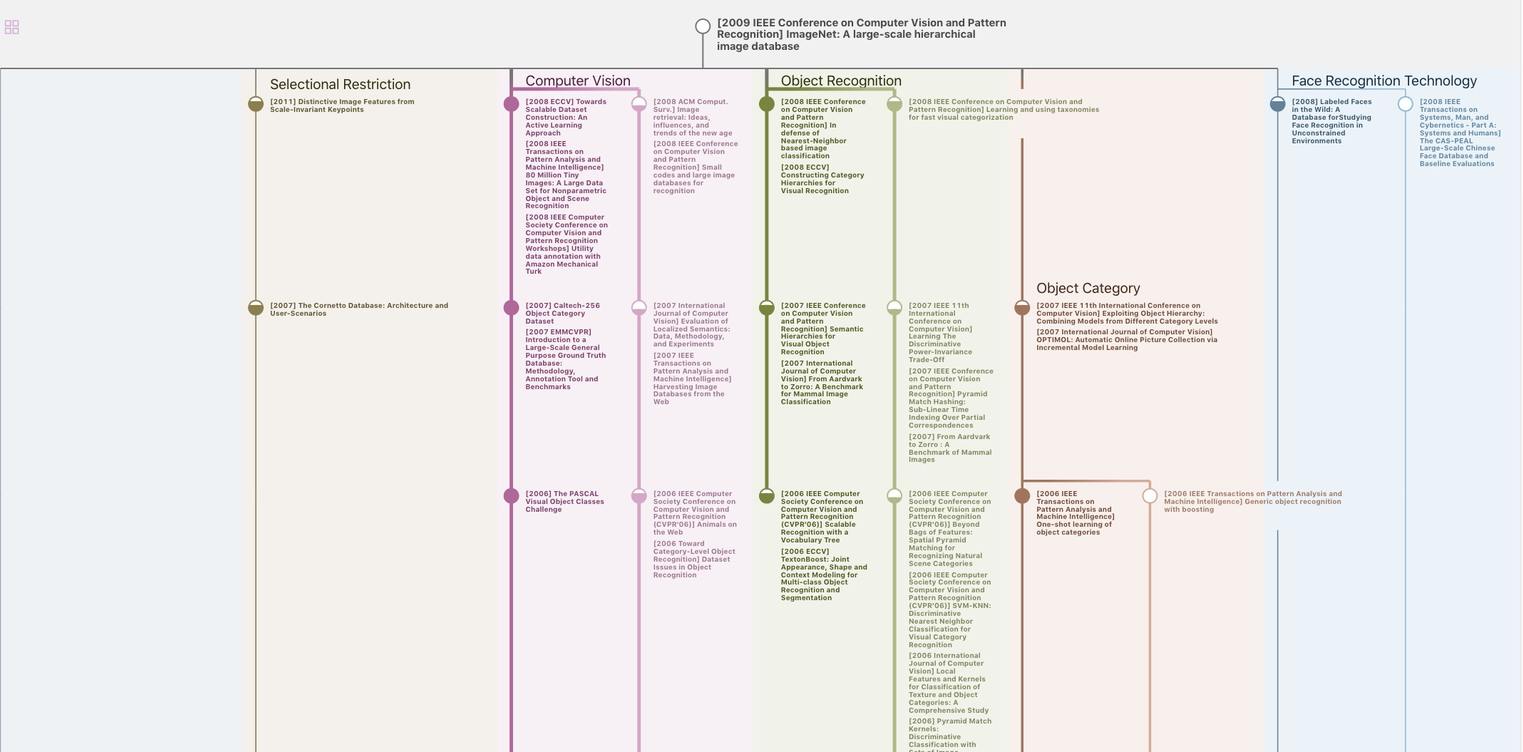
生成溯源树,研究论文发展脉络
Chat Paper
正在生成论文摘要