Diversified Contrastive Learning For Few-Shot Classification
ARTIFICIAL NEURAL NETWORKS AND MACHINE LEARNING, ICANN 2023, PT I(2023)
摘要
We argue that the current few-shot learning, which only uses contrastive learning as an auxiliary task, cannot fully realize the potential of contrastive learning. In this paper, we take a deeper exploration of how to combine contrastive learning and few-shot classification better. We use a two-stage training paradigm called pre-training and meta-training, respectively. During the pre-training phase, we differ from previous work that only extracted global features of images for contrastive learning. We extract the global features of the image and local features for contrastive learning, where the local feature contrastive loss is called the maximum matching local contrastive loss. To better integrate contrastive learning with few-shot learning, we propose a prototype contrastive module in the meta-training stage. During the meta-training phase, we record the feature vector representations of all base class prototypes and conduct class-level contrastive learning between K-way class prototypes obtained from the current task and all base class prototypes. Meanwhile, we dynamically update all stored base class prototypes as the training progresses. We validate our model on mimiImagenet and tired-Imagenet datasets. Our experimental results show meaningful improvements in few-shot classification and therefore demonstrate the usefulness of our model.
更多查看译文
关键词
Deep learning,Few-shot learning,Meta-learning,Contrastive learning
AI 理解论文
溯源树
样例
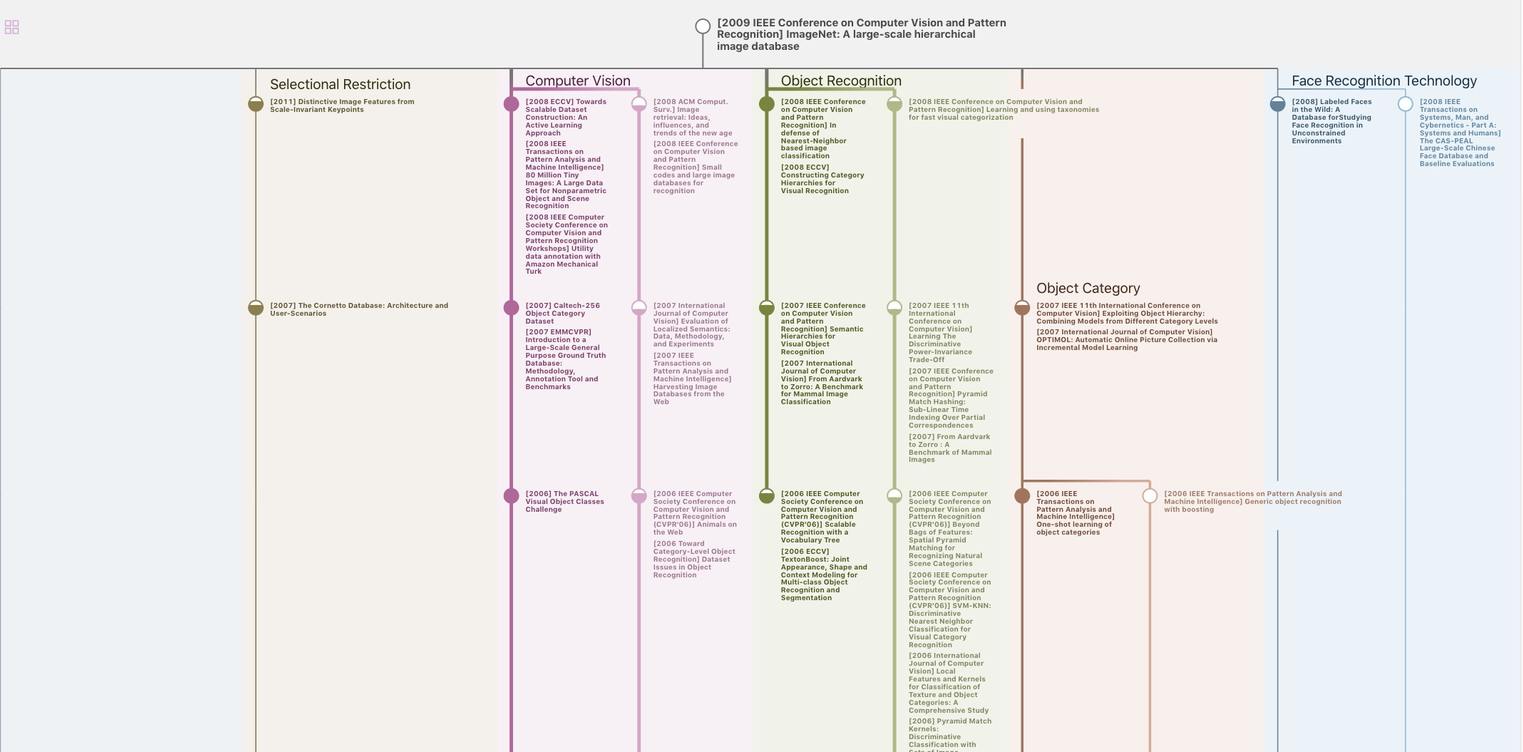
生成溯源树,研究论文发展脉络
Chat Paper
正在生成论文摘要