SS-Net: 3D Spatial-Spectral Network for Cerebrovascular Segmentation in TOF-MRA
ARTIFICIAL NEURAL NETWORKS AND MACHINE LEARNING, ICANN 2023, PT III(2023)
摘要
The extraction of cerebrovascular structure plays a pivotal role in the diagnosis and analysis of various cerebrovascular diseases. However, cerebrovascular segmentation from time-of-flight magnetic resonance angiography (TOF-MRA) volumes remains a challenging task due to the complex topology, slender contour, and noisy background. This paper proposes a 3D SS-Net that combines the spatial and spectral domain features to accurately segment the cerebral vasculature. The SS-Net is based on an end-to-end autoencoder architecture, which incorporates both spatial and a spectral encoders. The spectral encoder branch applies 3D fast Fourier convolution (FFC) to extract global features and frequency domain features in the shallow layers of the network. Furthermore, we introduce cerebrovascular edge supervised information, which enables the network to model the high-frequency variations and distribution patterns of cerebrovascular edges more effectively. Experimental results show that the SS-Net delivers outstanding performance, achieving the DSC of 71.14% on a publicly available dataset and outperforming other 3D deep-learning-based approaches. Code: github.com/y8421036/SS-Net.
更多查看译文
关键词
Cerebrovascular segmentation,Fast Fourier Convolution,3D U-Net,Edge supervision,TOF-MRA
AI 理解论文
溯源树
样例
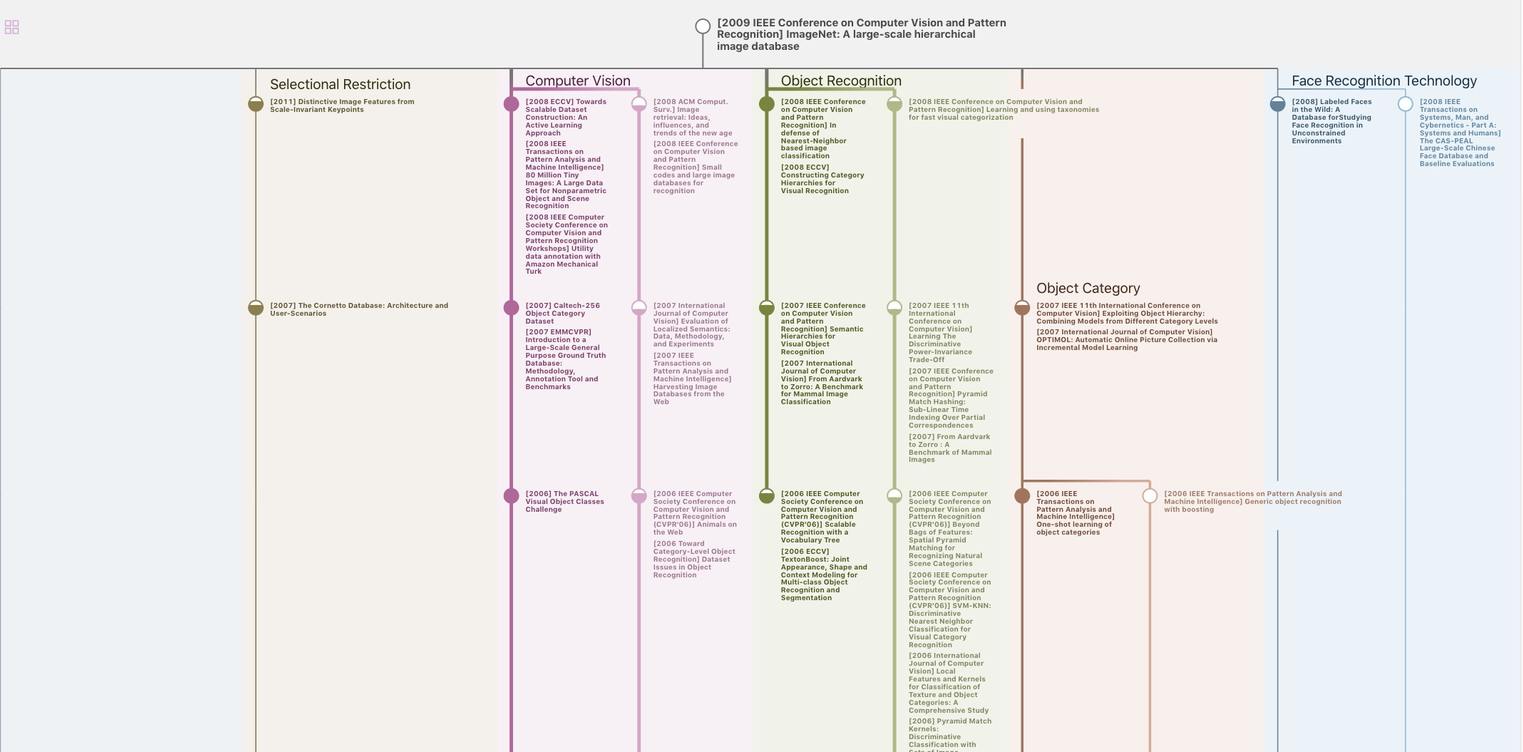
生成溯源树,研究论文发展脉络
Chat Paper
正在生成论文摘要