An Error-Based Measure for Concept Drift Detection and Characterization.
Learning and Intelligent Optimization: 17th International Conference, LION 17, Nice, France, June 4–8, 2023, Revised Selected Papers(2023)
摘要
Continual learning is an increasingly studied field, aiming at regulating catastrophic forgetting for online machine learning tasks. In this article, we propose a prediction error measure for continual learning, to detect concept drift induced from learned data input before the learning step. In addition, we check this measure’s ability for characterization of the drift. For these purposes, we propose an algorithm to compute the proposed measure on a data stream while also estimating concept drift. Then, we calculate the correlation coefficients between this estimate and our measurement, using time series analysis. To validate our proposal, we base our experiments on simulated streams of metadata collected from an industrial dataset corresponding to real conversation data. The results show that the proposed measure constitutes a reliable criterion for concept drift detection. They also show that a characterization of the drift relative to components of the stream is possible thanks to the proposed measure.
更多查看译文
关键词
concept drift detection,measure,error-based
AI 理解论文
溯源树
样例
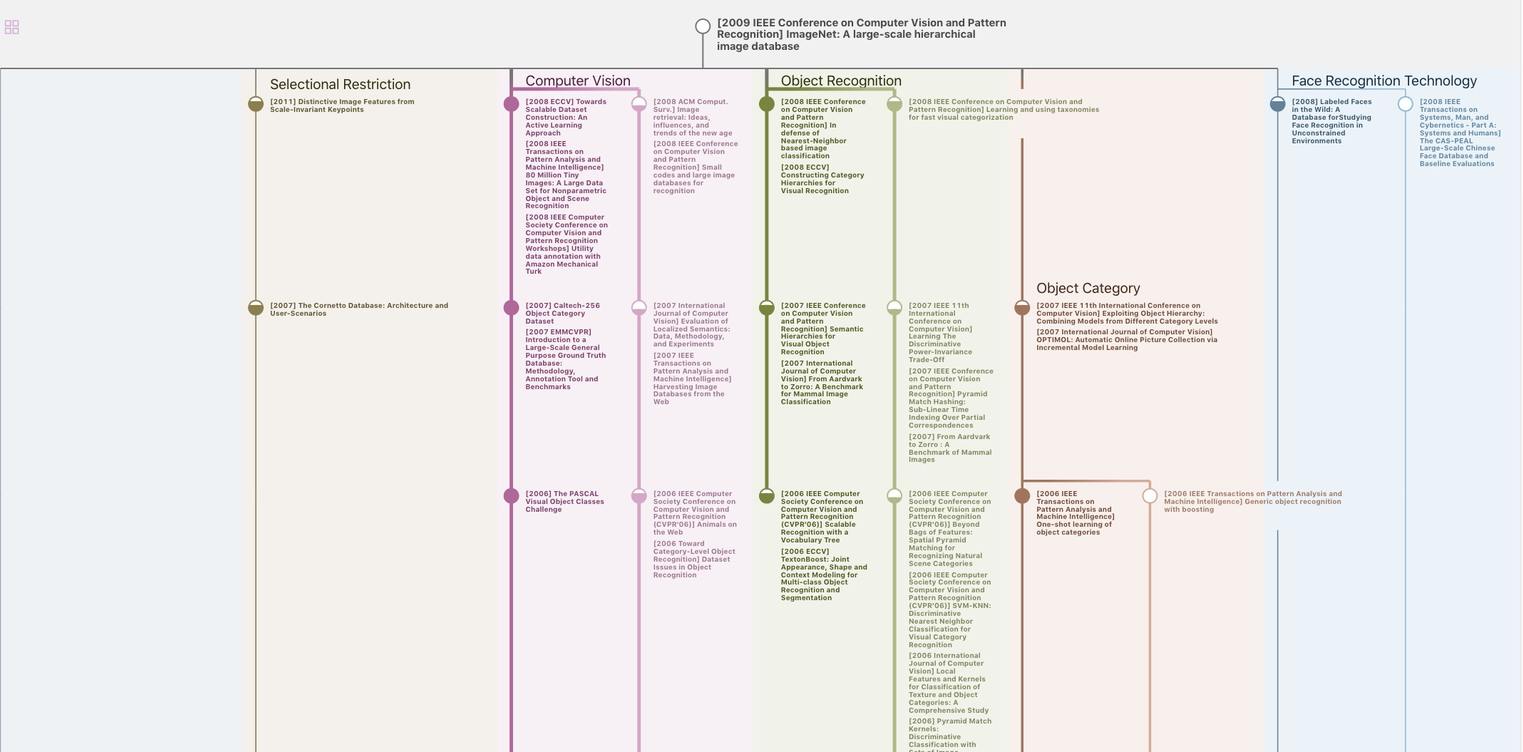
生成溯源树,研究论文发展脉络
Chat Paper
正在生成论文摘要