Robust Neural Network Approach to System Identification in the High-Noise Regime.
Learning and Intelligent Optimization: 17th International Conference, LION 17, Nice, France, June 4–8, 2023, Revised Selected Papers(2023)
摘要
We present a new algorithm for learning unknown governing equations from trajectory data, using a family of neural networks. Given samples of solutions x ( t ) to an unknown dynamical system x ˙ ( t ) = f ( t , x ( t ) ) , we approximate the function f using a family of neural networks. We express the equation in integral form and use Euler method to predict the solution at every successive time step using at each iteration a different neural network as a prior for f . This procedure yields M-1 time-independent networks, where M is the number of time steps at which x ( t ) is observed. Finally, we obtain a single function f ( t , x ( t )) by neural network interpolation. Unlike our earlier work, where we numerically computed the derivatives of data, and used them as target in a Lipschitz regularized neural network to approximate f , our new method avoids numerical differentiations, which are unstable in presence of noise. We test the new algorithm on multiple examples in a high-noise setting. We empirically show that generalization and recovery of the governing equation improve by adding a Lipschitz regularization term in our loss function and that this method improves our previous one especially in the high-noise regime, when numerical differentiation provides low quality target data. Finally, we compare our results with other state of the art methods for system identification.
更多查看译文
关键词
system identification,robust,neural network,high-noise
AI 理解论文
溯源树
样例
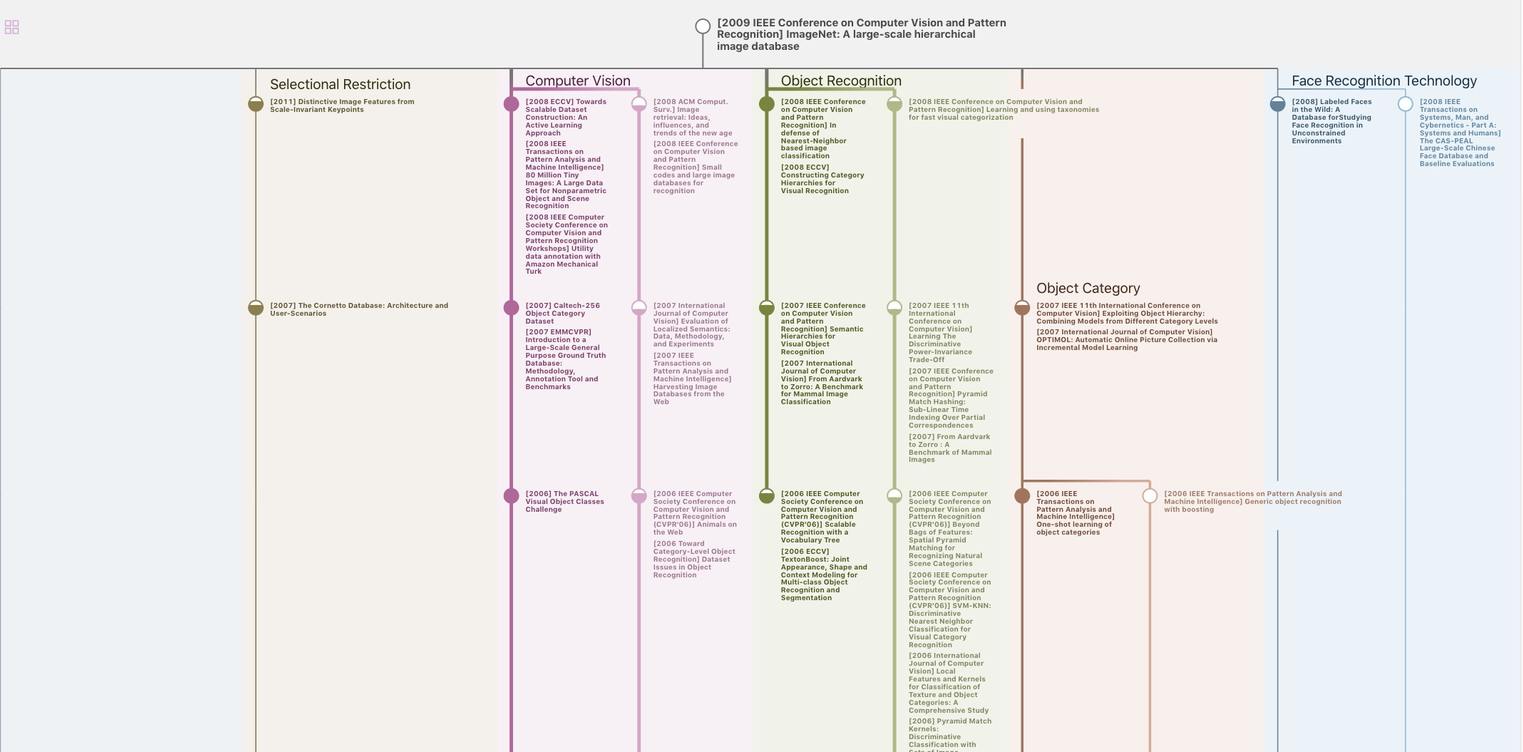
生成溯源树,研究论文发展脉络
Chat Paper
正在生成论文摘要