Local-Fusion Diffusion Model for Enhancing Few-Shot Image Generation.
Image and Graphics: 12th International Conference, ICIG 2023, Nanjing, China, September 22–24, 2023, Proceedings, Part I(2023)
摘要
In recent research, few-shot generation models have attracted increasing interest in computer vision. They aim at generating more data of a given domain, with only a few available training examples. Although many methods have been introduced to handle few-shot generation tasks, most of them are usually unstable during the training process and can only generate cookie-cutter images. To alleviate these issues, we propose a novel few-shot generation method based on the classifier-free conditional diffusion model. Specifically, we train an autoencoder on seen categories and then use patch discriminator adversarial training to achieve better reconstruction quality. Subsequently, for the k -shot task, we extract k image features and calculate the conditional information to guide the training generation of the diffusion model. To avoid the singularness of conditional information caused by the prototype model, we use the latest Feature Fusion module (LFM) to learn various features. We conduct extensive experiments on three well-known datasets and the experimental results clearly demonstrate the effectiveness of our proposed method for few-shot image generation.
更多查看译文
关键词
few-shot few-shot,diffusion,local-fusion
AI 理解论文
溯源树
样例
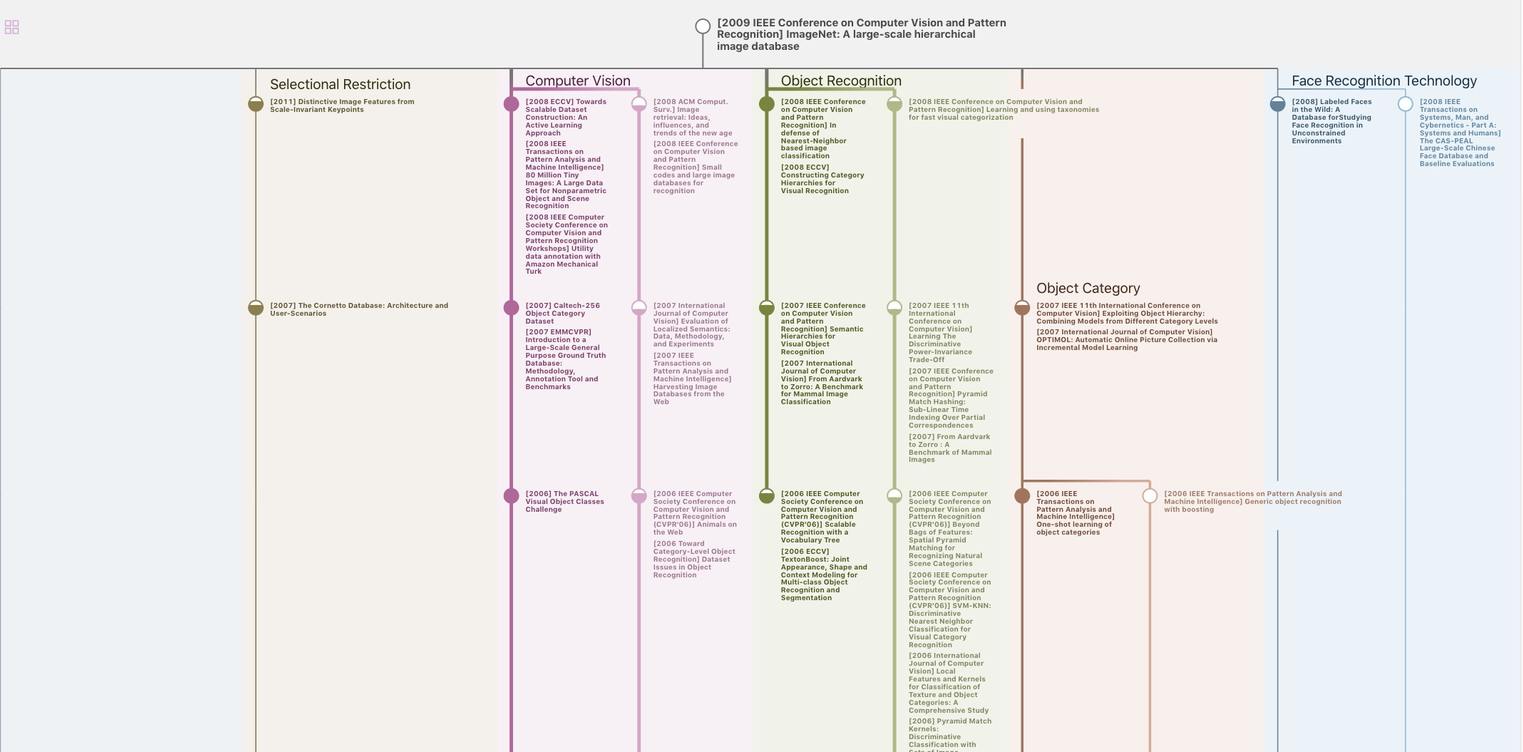
生成溯源树,研究论文发展脉络
Chat Paper
正在生成论文摘要