Edge-Guided Interpretable Neural Network for Image Compressive Sensing Reconstruction.
Image and Graphics : 12th International Conference, ICIG 2023, Nanjing, China, September 22–24, 2023, Proceedings, Part III(2023)
摘要
In recent years, interpretable Compressive Sensing (CS) technology has received more and more attention. Although these CS reconstruction methods can obtain good interpretability, they ignore the importance of edge information, which greatly limits the representation ability of their reconstruction models. Therefore, this paper reasonably embeds the boundary consistency constraint to the CS optimization model and proposes a CS reconstruction model with boundary constraint. This model can be expanded into Edge-Guided Interpretable Neural Network (EGINNet) for image CS reconstruction. In particular, in order to solve the problem of information loss in the iterative process, we introduce a multiple-memory enhancement mechanism to explore the feature dependency of EGINNet across different iterative stages. At the same time, we design a parallel-cross fusion module, which can selectively fuse boundary features and image features. Qualitative and quantitative experimental results show that compared with the state-of-the-art explainable CS reconstruction methods, the proposed EGINNet has better reconstruction performance while maintaining the network interpretability, especially on the restoration of boundary information.
更多查看译文
关键词
image compressive sensing reconstruction,interpretable neural network,neural network,edge-guided
AI 理解论文
溯源树
样例
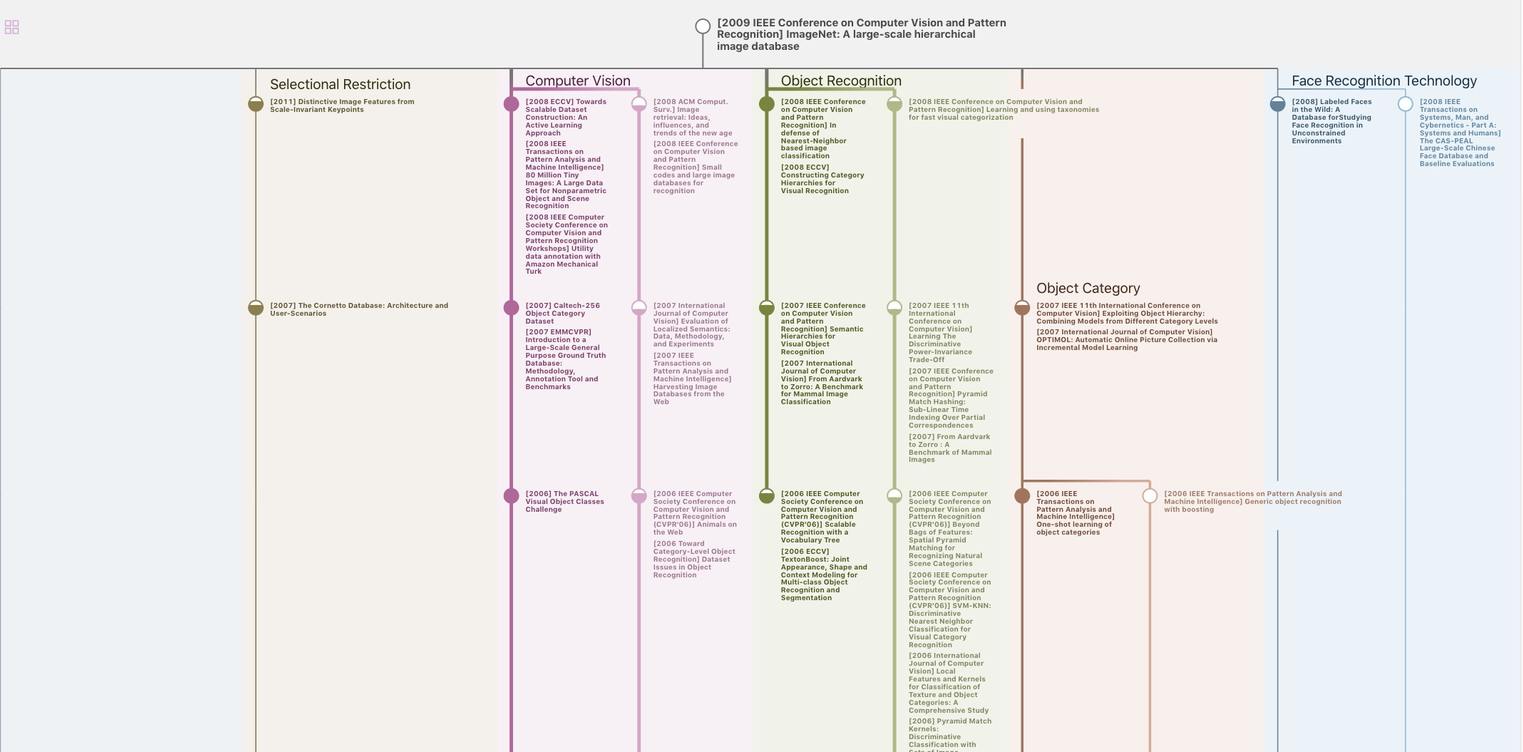
生成溯源树,研究论文发展脉络
Chat Paper
正在生成论文摘要