Spatial Commonsense Reasoning for Machine Reading Comprehension.
Advanced Data Mining and Applications: 19th International Conference, ADMA 2023, Shenyang, China, August 21–23, 2023, Proceedings, Part II(2023)
摘要
This paper studies the problem of spatial commonsense reasoning for the machine reading comprehension task. Spatial commonsense is the human-shared but latent knowledge of object shape, size, distance, and position. Reasoning this abstract knowledge can facilitate machines better perceive their surroundings, which is crucial for general intelligence. However, this valuable topic is challenging and has been less studied. To bridge this research gap, we focus on this topic and propose a new method to realize spatial reasoning. Given a text, we first build a potential reasoning graph based on its parsing tree. To better support spatial reasoning, we retrieve the related commonsense entities and relations from external knowledge sources, including the pre-trained language model (LM) and knowledge graph (KG). LM covers all kinds of factual knowledge and KG has abundant commonsense relations. We then propose a new fusion method called LEGRN (LM Edge-GNN Reasoner Networks) to fuse the text and graph. LEGRN adopts layer-based attention to integrate the LM text encoder and KG graph encoder, which can capture correlations between LM text context and KG graph structure. Considering that spatial relations involve a variety of attributes, we propose an attribute-aware inferential network to deduce the correct answers. To evaluate our approach, we construct a new large-scale dataset named CRCSpatial, consisting of 40k spatial reasoning questions. Experiment results illustrated the effectiveness of our approach.
更多查看译文
关键词
spatial commonsense reasoning,reading comprehension
AI 理解论文
溯源树
样例
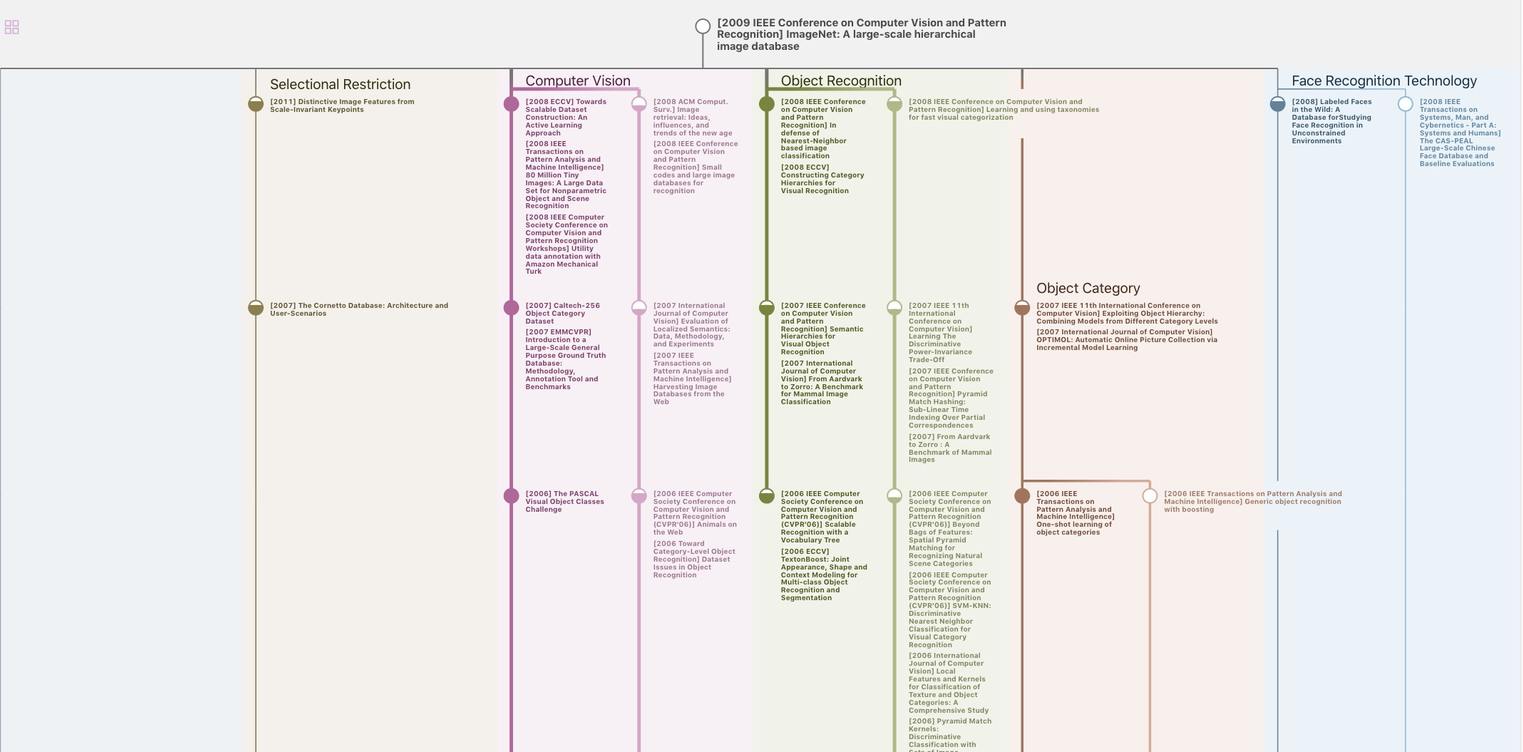
生成溯源树,研究论文发展脉络
Chat Paper
正在生成论文摘要