Multi-level Transformer for Cancer Outcome Prediction in Large-Scale Claims Data.
Advanced Data Mining and Applications: 19th International Conference, ADMA 2023, Shenyang, China, August 21–23, 2023, Proceedings, Part III(2023)
摘要
Predicting outcomes for cancer patients initiating chemotherapy is essential for care planning and offers potential to support clinical and health policy decision-making. Existing models leveraging deep learning with longitudinal healthcare data have demonstrated the benefits of Transformer-based approaches to learning temporal relationships among medical codes (e.g., diagnoses, medications, procedures). Recent applications have also recognised the benefit of including patient information such as demographics to improve predictions. However, much of the existing work has focused on Electronic Health Record (EHR) data, and applications to administrative claims data, which has a differing temporal structure to EHR, are limited. Furthermore, it is still unclear how to best encode medical data from both EHR and claims data and model it collectively in Transformer models. Motivated by the above, this work proposes a Multi-Level Transformer specifically designed for claims data (Claims-MLT) to enhance cancer outcome prediction. The model uses a dual-level structure to learn effective patient representations by considering the low-level claims item relationships and sequential patterns in patient claim histories. We also integrate patient demographic and clinical features to provide additional information to the model. We evaluate our approach on two tasks from a real-world cancer dataset containing breast and colorectal cancer patients, and demonstrate the proposed model outperforms comparative baselines.
更多查看译文
关键词
cancer outcome prediction,outcome prediction,multi-level,large-scale
AI 理解论文
溯源树
样例
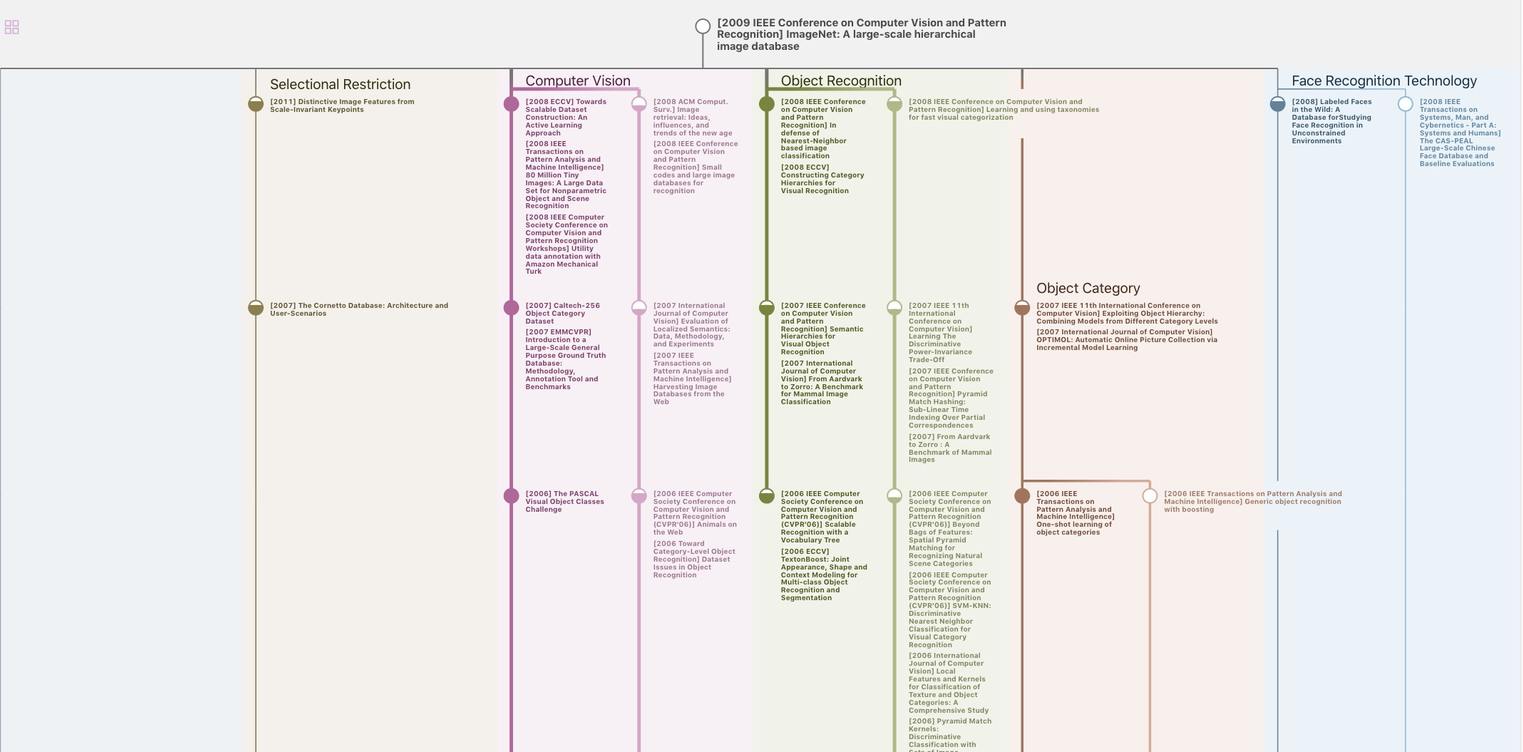
生成溯源树,研究论文发展脉络
Chat Paper
正在生成论文摘要