Toward an Efficient in-Network Caching Using Federated Learning
2023 5th International Conference on Pattern Analysis and Intelligent Systems (PAIS)(2023)
摘要
In today’s digital age, the Internet has experienced remarkable growth, accompanied by an exponential increase in both the diversity of available content and the number of users. Consequently, the demand for server resources and the volume of server requests have surged significantly. This places a significant strain on servers, diminishing their ability to handle user demands effectively. To alleviate this issue, caching is employed to store frequently requested content in memory that is closer to users. However, determining which content should be cached poses a challenge. Efficient cache management plays a vital role in enhancing data access speed and overall efficiency. This challenge has been extensively studied and applied in the context of federated learning engineering, where effective cache management techniques are crucial for optimizing the performance of distributed machine learning models. By addressing cache management challenges, researchers aim to improve scalability, efficiency, and overall system performance, ultimately enhancing the effectiveness of federated learning methodologies. In this paper, we conducted a study on enhancing network caching efficiency by implementing federated learning. Our study involved the creation of different users, each of whom was assigned different databases with the same purpose (e.g., movies). The main aim is to identify the most popular content using artificial neural networks and cache them for each user, thus improving delivery services within the network by bringing these contents closer to the respective users.
更多查看译文
关键词
Federated learning,cache management,cache decision,Caching in network,Placement strategies,Replacement strategies,Machine learning
AI 理解论文
溯源树
样例
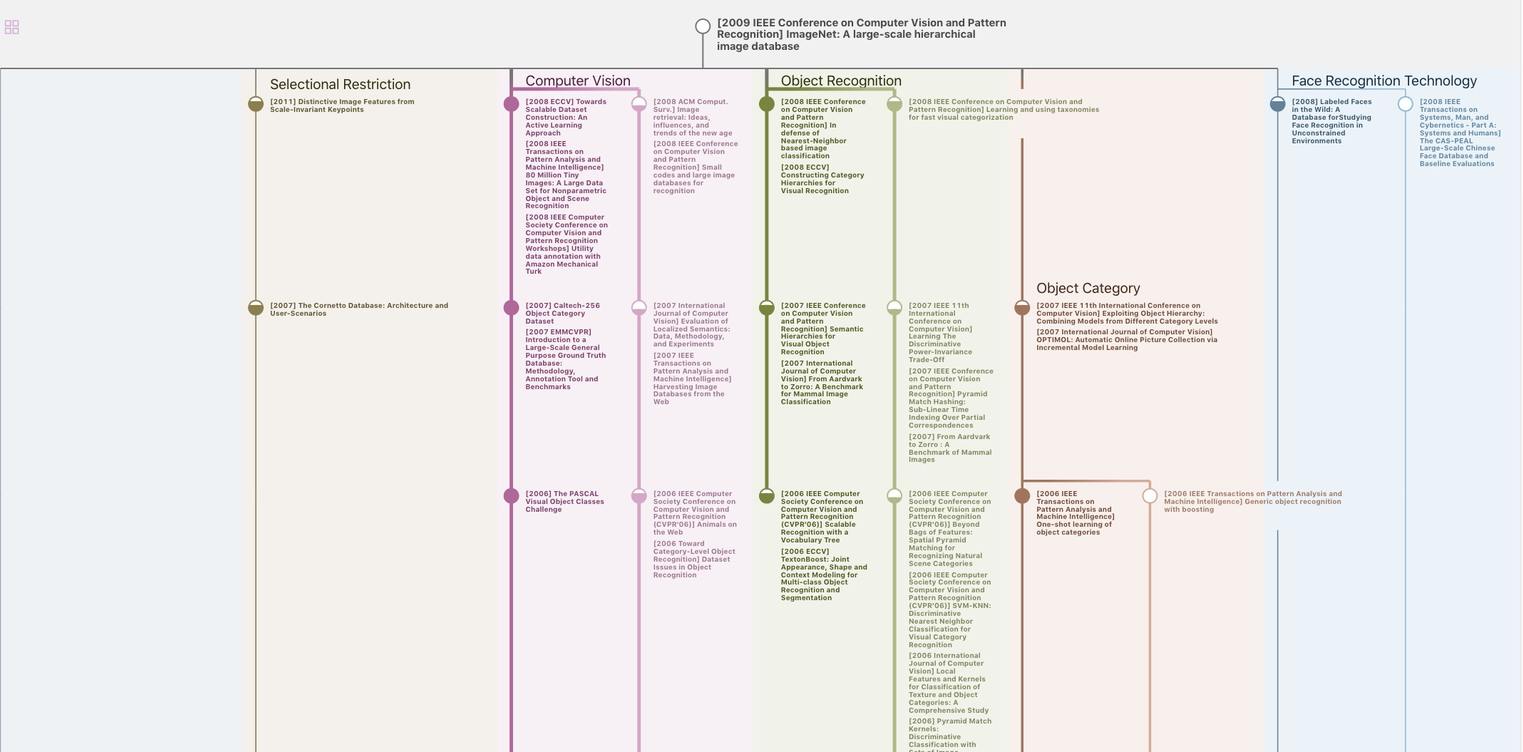
生成溯源树,研究论文发展脉络
Chat Paper
正在生成论文摘要