Poster: Detecting Adversarial Examples Hidden under Watermark Perturbation via Usable Information Theory
PROCEEDINGS OF THE 2023 ACM SIGSAC CONFERENCE ON COMPUTER AND COMMUNICATIONS SECURITY, CCS 2023(2023)
摘要
Image watermark is a technique widely used for copyright protection. Recent studies show that the image watermark can be added to the clear image as a kind of noise to realize fooling deep learning models. However, previous adversarial example (AE) detection schemes tend to be ineffective since the watermark logo differs from typical noise perturbations. In this poster, we propose Themis, a novel AE detection method against watermark perturbation. Different from prior methods, Themis neither modifies the protected classifier nor requires knowledge of the process for generating AEs. Specifically, Themis leverages usable information theory to calculate the pointwise score, thereby discovering those instances that may be watermark AEs. The empirical evaluations involving 5 different logo watermark perturbations demonstrate the proposed scheme can efficiently detect AEs, and significantly (over 15% accuracy) outperforms five state-of-the-art (SOTA) detection methods. The visualization results display our detection metric is more distinguishable between AEs and non-AEs. Meanwhile, Themis realizes a larger Area Under Curve (AUC) in a threshold-resilient manner, while only introducing similar to 0.04s overhead.
更多查看译文
关键词
Watermark Adversarial Examples,Usable Information Theory
AI 理解论文
溯源树
样例
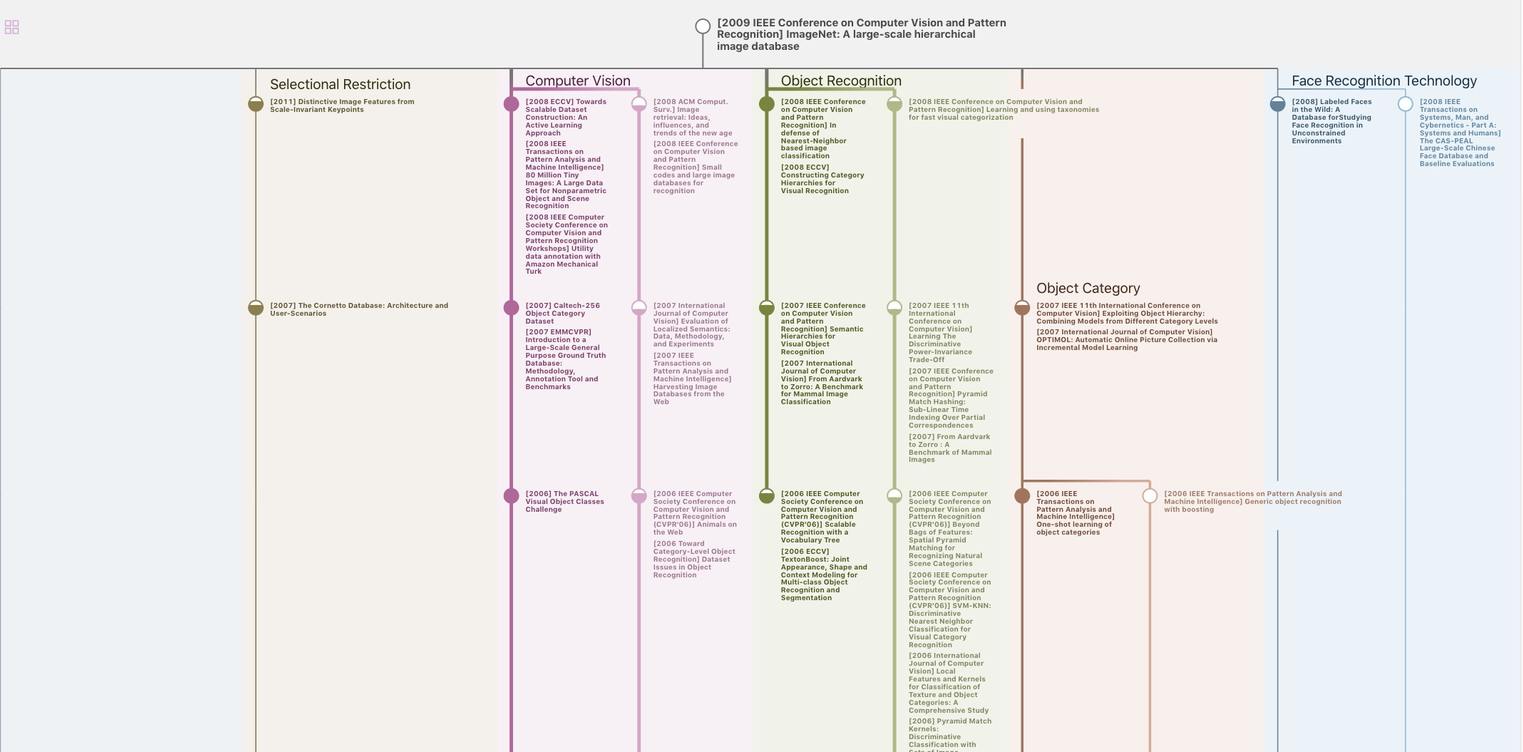
生成溯源树,研究论文发展脉络
Chat Paper
正在生成论文摘要