Hierarchical Attention based Feature Learning for Interpretable Social Event Prediction
CVIPPR '23: Proceedings of the 2023 Asia Conference on Computer Vision, Image Processing and Pattern Recognition(2023)
摘要
abstract. Major social events, e.g., civil unrests, generally impact both social stability and civil life. Therefore, anticipating the occurrence of concerned social events in advance is of great significance to decision makers. By mining previous indicators of the event type of interest from open-source data, we can make inference on whether a particular one of that type will occur sometime in the future. In recent years, this kind of data-driven approaches have been proposed to predict social events. However, there are still some challenges remaining to be addressed: (I) Modeling previous feature for a particular event based on limited and obtainable data source. (II) Mining temporal dependences between complicated information in different periods. (III) Explaining prediction results from a reasonable perspective. To cope with these research issues, we proposed a hierarchical attention-based feature learning framework for interpretable social event prediction. We model the evolution processes prior to the onset of an event of interest using a sequence of temporal event graphs. Then, we employ the GNN (Graph Neural Network) approach for graph mining and the attention mechanism on multi-level data for feature learning. For model explanation, an importance evaluation indicator is proposed to identify influential factors of distinct feature levels leading to the event occurrence from the past. Additionally, we conduct experiments on four real-world datasets to verify the proposed method. The results indicate that it outperforms other baseline models on protest prediction tasks.
更多查看译文
AI 理解论文
溯源树
样例
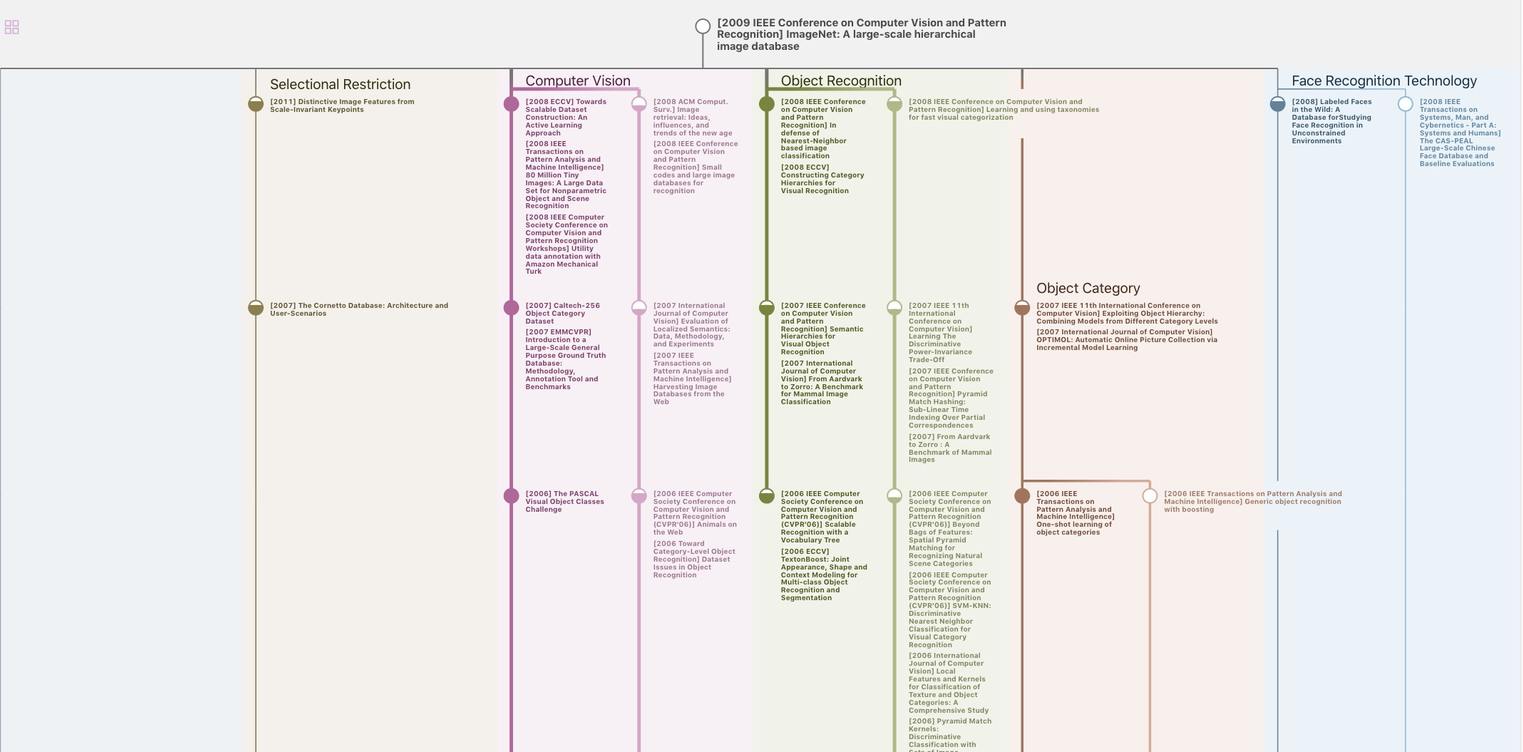
生成溯源树,研究论文发展脉络
Chat Paper
正在生成论文摘要