Breaking the Anonymity of Ethereum Mixing Services Using Graph Feature Learning
IEEE TRANSACTIONS ON INFORMATION FORENSICS AND SECURITY(2024)
摘要
With the property of helping users further enhance the anonymity of transactions, mixing services in blockchain have gained wide popularity in recent years. However, the strong untraceability offered by mixing services has led to the abuse of them by criminals for money laundering and committing fraud. These illegal actions pose significant threats to the blockchain ecosystem and financial order. In this paper, we focus on the problem of correlating the addresses of mixing transactions in Tornado Cash, a widely-used mixing service on Ethereum. We propose a graph neural network framework named MixBroker, which aims to break the anonymity of Tornado Cash by correlate mixing addresses from the perspective of node-pair link prediction. Specifically, we construct a Mixing Interaction Graph (MIG) using raw Ethereum mixing transaction data that can be used for subsequent analysis. To better represent the properties of mixing account nodes, we extract features from account nodes in the MIG from multiple perspectives. Furthermore, we design a GNN-based link prediction mechanism to serve as the backbone of MixBroker. This mechanism captures the interconnected nature of nodes within the MIG and calculates the probability of correlation between account nodes through node embeddings. In addition, to solve the problem of lacking ground-truth, we collect a large number of real mixing transactions of Ethereum in Tornado Cash and construct a ground-truth dataset by combining the principles of Ethereum Name Service (ENS). We conduct extensive experiments on the datasets, and the results demonstrate that MixBroker has a superior performance over other state-of-the-art methods on the address correlation problem in Ethereum mixing transactions.
更多查看译文
关键词
Tornadoes,Correlation,Feature extraction,Receivers,Blockchains,Topology,Representation learning,Blockchain,ethereum,mixing services,deep learning,graph neural networks
AI 理解论文
溯源树
样例
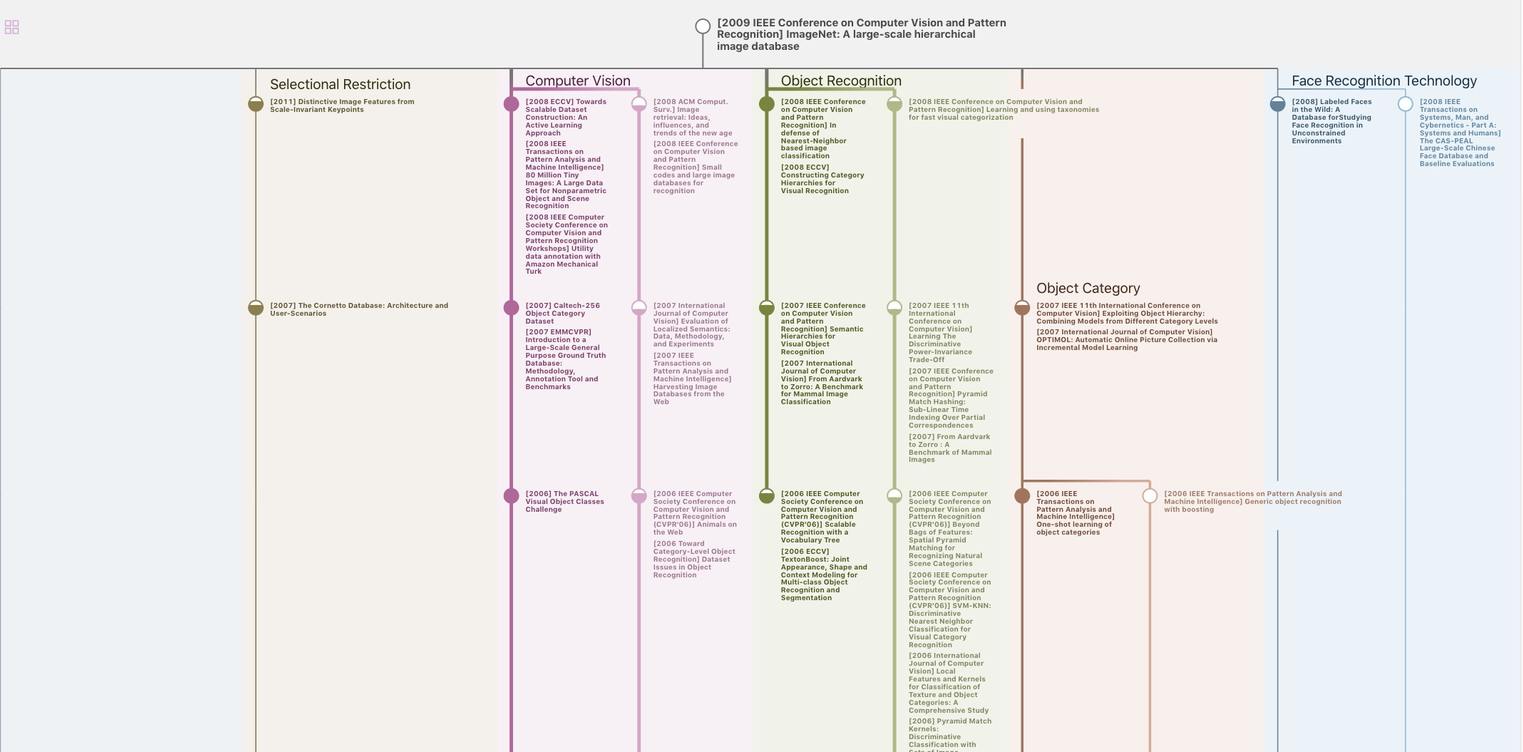
生成溯源树,研究论文发展脉络
Chat Paper
正在生成论文摘要