Ensemble transport smoothing. Part II: Nonlinear updates
Journal of Computational Physics: X(2023)
摘要
Smoothing is a specialized form of Bayesian inference for state-space models that characterizes the posterior distribution of a collection of states given an associated sequence of observations. Ramgraber et al. [38] proposes a general framework for transport-based ensemble smoothing, which includes linear Kalman-type smoothers as special cases. Here, we build on this foundation to realize and demonstrate nonlinear backward ensemble transport smoothers. We discuss parameterization and regularization of the associated transport maps, and then examine the performance of these smoothers for nonlinear and chaotic dynamical systems that exhibit non-Gaussian behavior. In these settings, our nonlinear transport smoothers yield lower estimation error than conventional linear smoothers and state-of-the-art iterative ensemble Kalman smoothers, for comparable numbers of model evaluations.
更多查看译文
关键词
Data assimilation,Smoothing,Ensemble methods,Triangular transport
AI 理解论文
溯源树
样例
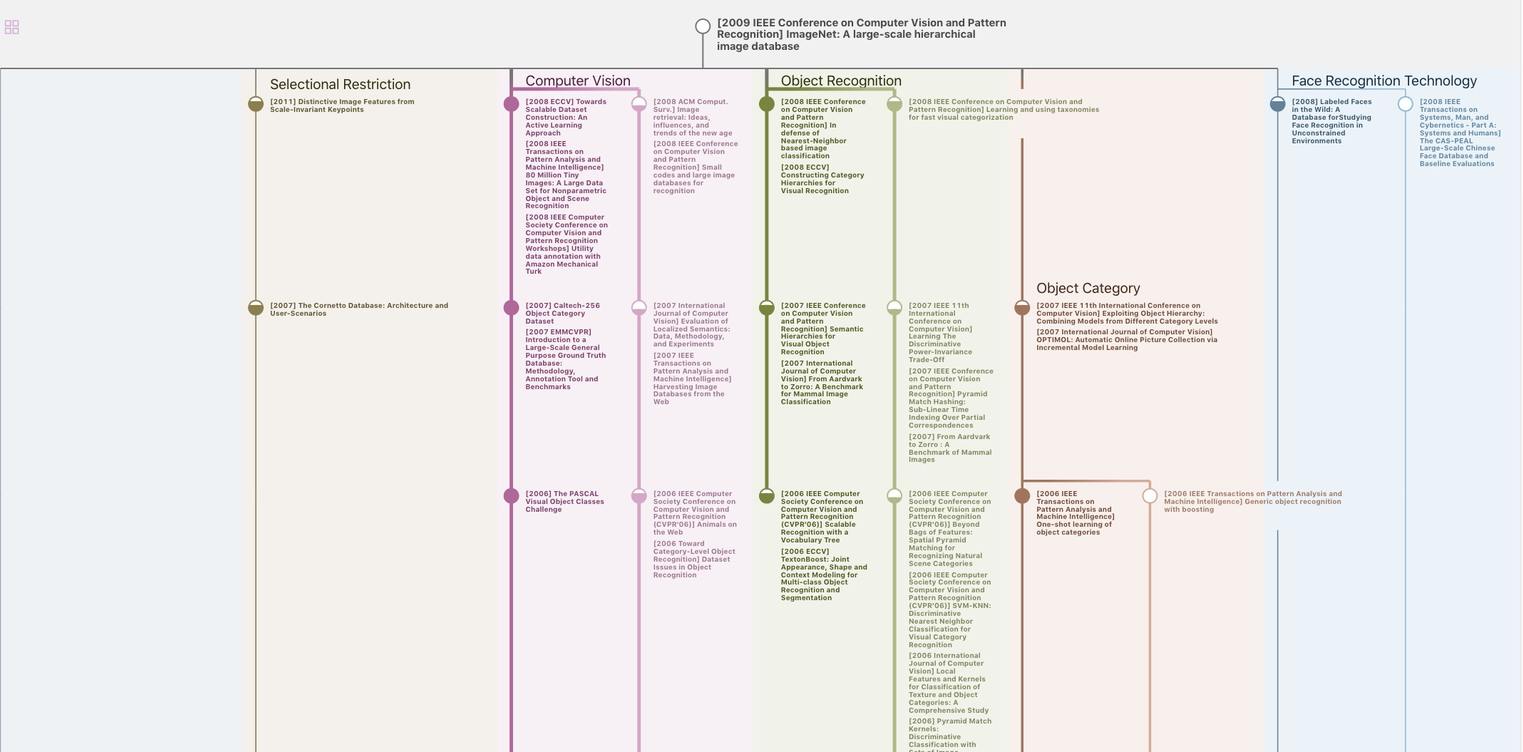
生成溯源树,研究论文发展脉络
Chat Paper
正在生成论文摘要