A dual progressive strategy for long-tailed visual recognition
Machine Vision and Applications(2023)
摘要
Unlike the roughly balanced dataset used in the experiments, the long-tail phenomenon in the dataset is more common when applied in practice. Most previous work has typically used re-sampling, re-weighting, and ensemble learning to mitigate the long-tail problem. The first two are the most commonly used (as are we) due to their better generality. Differently, assigning weights to classes directly using the inverse of the sample size to solve such problems may not be a good strategy, which often sacrifices the performance of the head classes. We propose a new approach to cost allocation, which consists of two parts: the first part is trained in an unweighted manner to ensure that the network is adequately fitted to the head data. The second part then dynamically assigns weights based on the relative difficulty of the class levels.In addition, we propose a novel, practical Grabcut-based data augmentation approach to increase the diversity and differentiation of the mid-tail class data. Extensive experiments on public and self-constructed long-tailed datasets demonstrate the effectiveness of our approach and achieve excellent performance.
更多查看译文
关键词
Long-tailed recognition,Imbalanced learning,Image classification,Deep learning
AI 理解论文
溯源树
样例
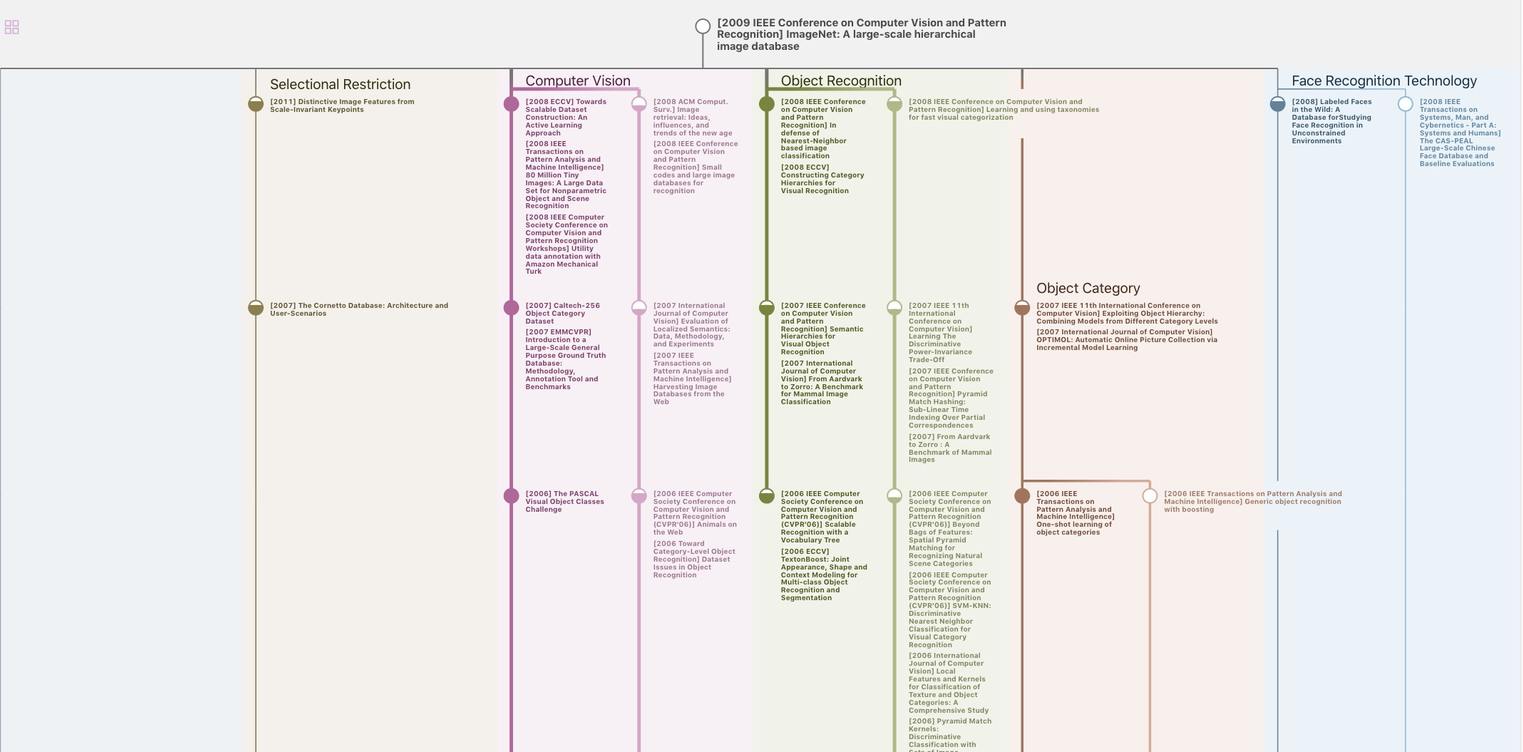
生成溯源树,研究论文发展脉络
Chat Paper
正在生成论文摘要