On Removing Potential Redundant Constraints For Svor Learning
APPLIED SOFT COMPUTING(2021)
摘要
As an extension of support vector machine in ordinal regression problem, support vector ordinal regression (SVOR) finds (r - 1) parallel hyperplanes by solving a quadratic programming which has n(r - 1) constraints. Here, n and r represent the number of training sample and ranks, respectively. Therefore, it would costs much more time to train SVOR than SVC or SVR. Fortunately, the solution of SVOR is only decided by minor constraints which are associated with non-zero Lagrange multipliers. Other constraints with zero Lagrange multipliers have no influence on the solution. Because a training sample is associated with (r - 1) constraints, retaining potential support vector may still induce that many redundant constraints are reserved. In this paper, we try to remove these potential redundant constraints for SVOR learning. For the jth parallel hyperplane, the potential constraints with non-zero Lagrange multipliers are associated with the samples near the jth parallel hyperplane. These samples can be identified by a chain near the jth parallel hyperplane. Then, other constraints for the jth parallel hyperplane can be discarded before learning. The number of the constraints can be reduced to less than 17 percent of the original in our experiments. Additionally, it only executes once to find potential critical constraints. Obviously, it is easy to tune parameters after removing potential redundant constraints. The experimental results on several datasets demonstrate that SVOR becomes much faster after removing potential redundant constraints and the performance does not degrade seriously. (C) 2020 Elsevier B.V. All rights reserved.
更多查看译文
关键词
Support vector ordinal regression, Redundant constraint, Extended nearest neighbor chain
AI 理解论文
溯源树
样例
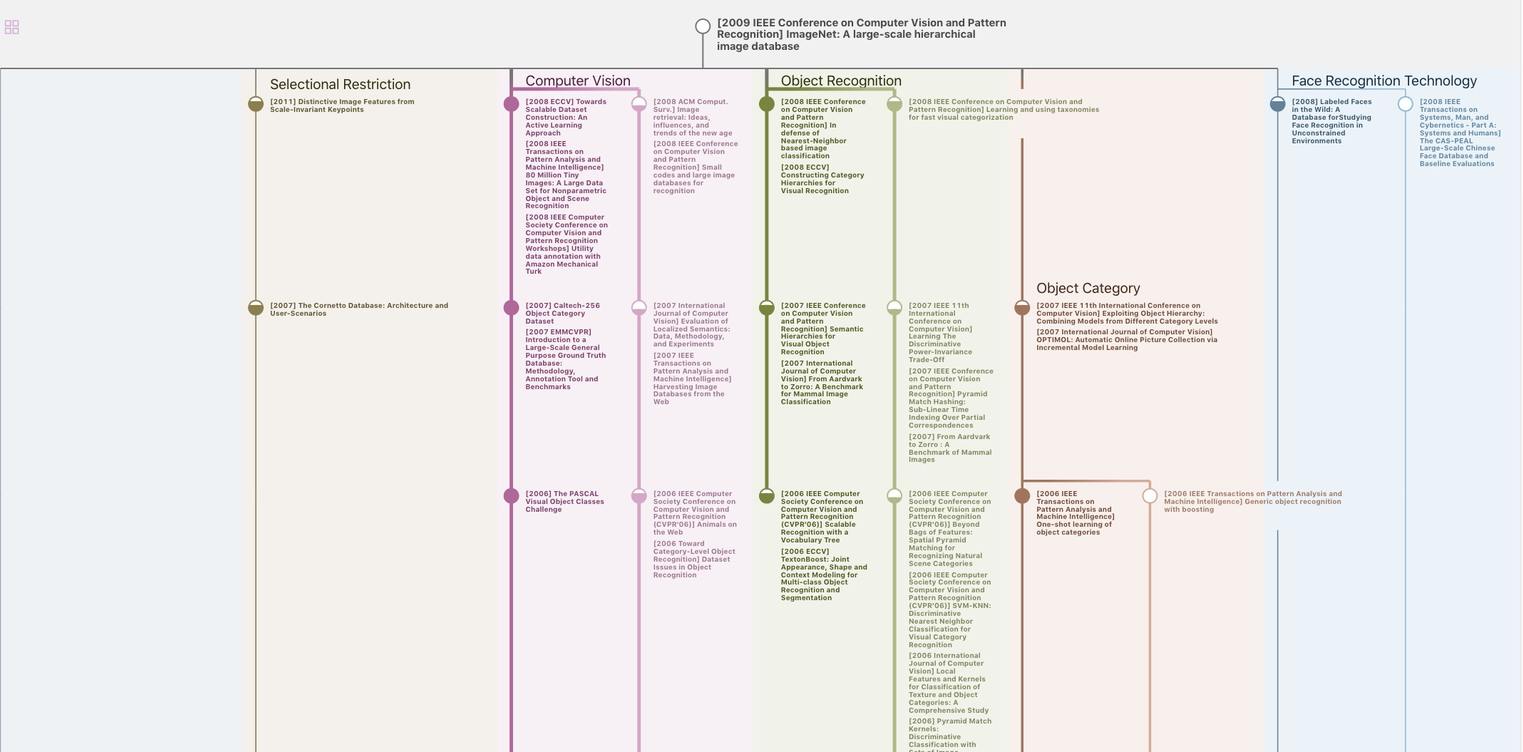
生成溯源树,研究论文发展脉络
Chat Paper
正在生成论文摘要