Interpretable Image Recognition in Hyperbolic Space
2023 ASIA PACIFIC SIGNAL AND INFORMATION PROCESSING ASSOCIATION ANNUAL SUMMIT AND CONFERENCE, APSIPA ASC(2023)
摘要
The lack of human-level interpretability in the decision-making process of computer vision is one of the obstacles to further its application and performance improvement. This issue is investigated by eXplainable Artificial Intelligence (XAI), and a range of interpretable XAI networks has been proposed to mitigate the black box issue. Most of them attempt to implement case-based reasoning in image recognition that is similar to humans. This cognitive process includes dissecting the image and identifying prototypical parts that distinct one class from another. However, only a few works take into consideration the hierarchical principle of this process and visual data. In contrast to previously introduced XAI approaches that utilize Euclidean space to design a network, hyperbolic space with its ability to embed hierarchical structures is exploited in this work. The proposed prototype-based hyperbolic network, namely ProtoHyp, first learns prototypes that play key roles in identifying classes. Then, using the similarity between them and an image, the human-understandable classification of an image is conducted in hyperbolic space. Experimental results on two image recognition datasets, Birds CUB-200-2011 and Stanford Dogs, have demonstrated the improvement of state-of-the-art interpretable image recognition in terms of both accuracy and interpretability.
更多查看译文
AI 理解论文
溯源树
样例
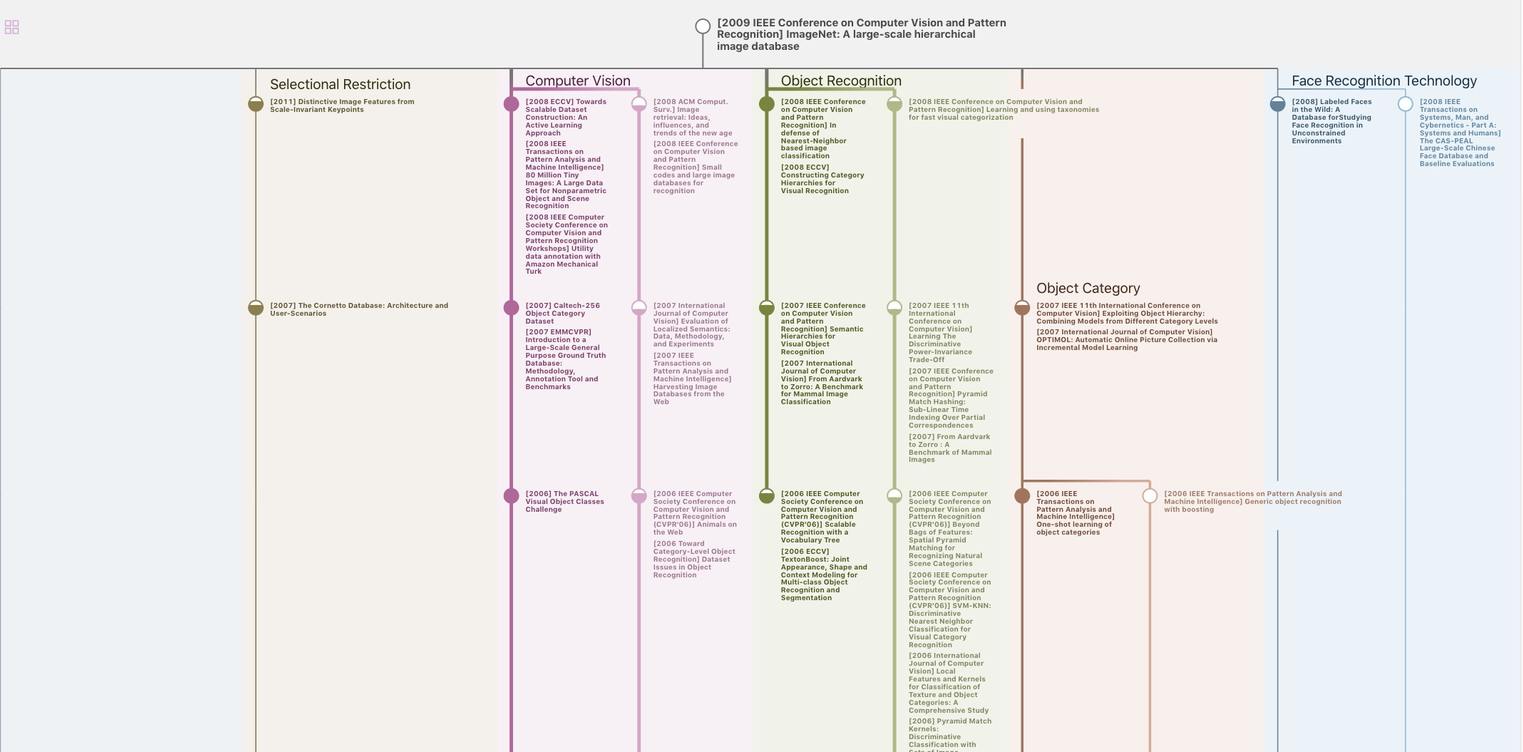
生成溯源树,研究论文发展脉络
Chat Paper
正在生成论文摘要