Synthetic Data Augmentation for ASR with Domain Filtering
2023 ASIA PACIFIC SIGNAL AND INFORMATION PROCESSING ASSOCIATION ANNUAL SUMMIT AND CONFERENCE, APSIPA ASC(2023)
摘要
Recent studies have shown that synthetic speech can effectively serve as training data for automatic speech recognition models. Text data for synthetic speech is mostly obtained from in-domain text or generated text using augmentation. However, obtaining large amounts of in-domain text data with diverse lexical contexts is difficult, especially in low-resource scenarios. This paper proposes using text from a large generic-domain source and applying a domain filtering method to choose the relevant text data. This method involves two filtering steps: 1) selecting text based on its semantic similarity to the available in-domain text and 2) diversifying the vocabulary of the selected text using a greedy-search algorithm. Experimental results show that our proposed method outperforms the conventional text augmentation approach, with the relative reduction of word-error-rate ranging from 6% to 25% on the LibriSpeech dataset and 15% on a low-resource Vietnamese dataset.
更多查看译文
关键词
speech recognition,domain filtering,semantic similarity maximization,vocabulary coverage maximization
AI 理解论文
溯源树
样例
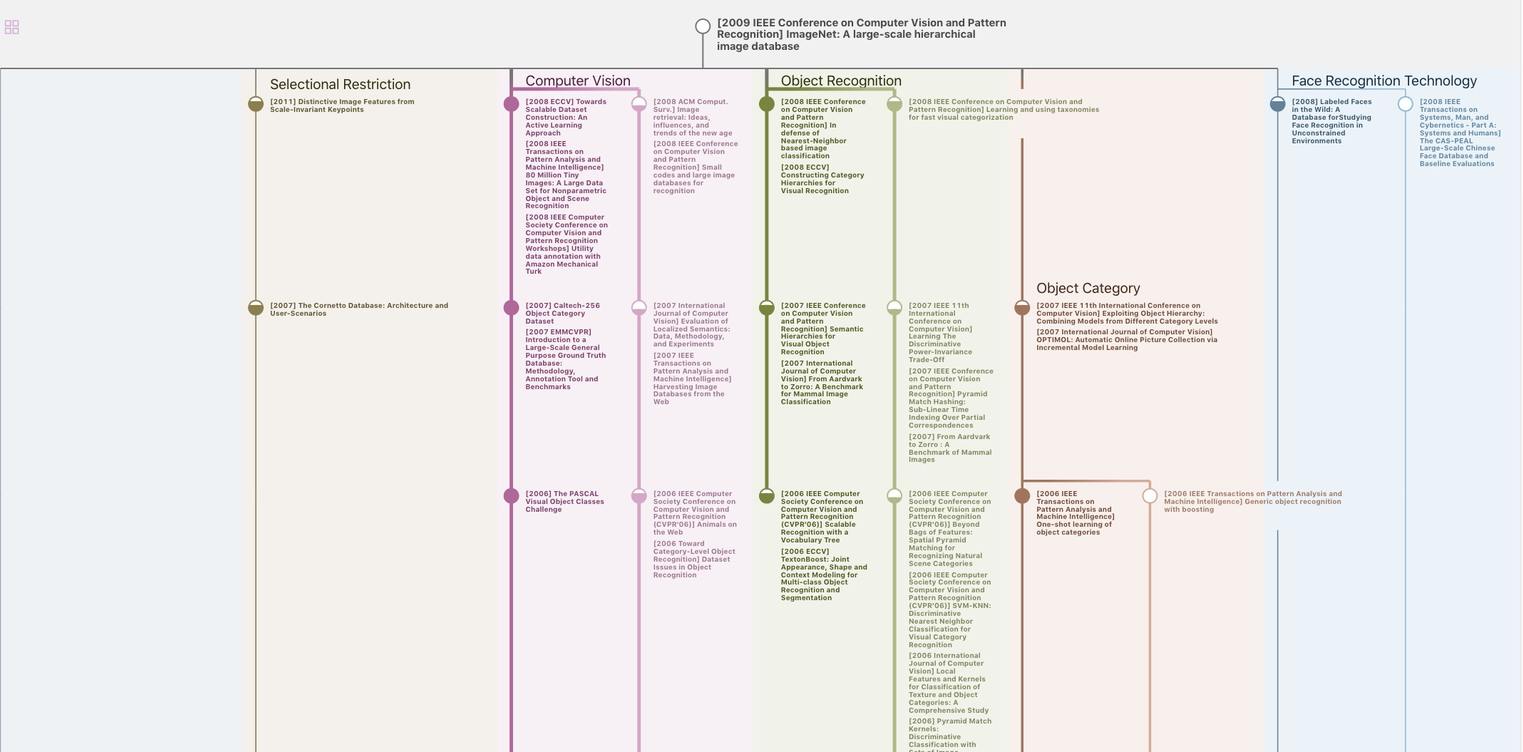
生成溯源树,研究论文发展脉络
Chat Paper
正在生成论文摘要