ARdetector: android ransomware detection framework
The Journal of Supercomputing(2024)
摘要
Ransomware has affected a broad range of public and private-sector organizations, and the impacts include direct and indirect financial loss (e.g., opportunity costs), reputational damage, legal implications, and physical consequences (e.g., fatalities). However, it has been challenging to accurately detect ransomware. For example, ransomware’s behavioral characteristics differ from many other malicious applications, and it can be laborious to obtain representative features that can be used for machine learning training. In addition, the issue of the imbalanced dataset of minority and majority classes complicates efforts for machine learning models to learn the internal patterns of minority classes. In an effort for tackling such difficulties, we suggest an architecture regarding Android ransomware detection (hereafter referred to as ARdetector), which allows one to analyze the relationship between behav oral characteristics and other candidate features associated with ransomware to select more representative features. In addition, we design a deep neural network based on focal loss, which lowers the loss generated by the majority class. In our evaluations, we use two real-world datasets of different class proportions, and the findings show that the accuracy of the ARdetector on both datasets is over 99.00% when the imbalanced ratio is 6. Specifically, via the BMR dataset, the AUC as a synthetical categorizing assessment indicator reaches as high as 0.9625 at that ratio of 15.
更多查看译文
关键词
Android mobile,Convolutional neural networks (CNN),Focal loss,Imbalanced,Ransomware
AI 理解论文
溯源树
样例
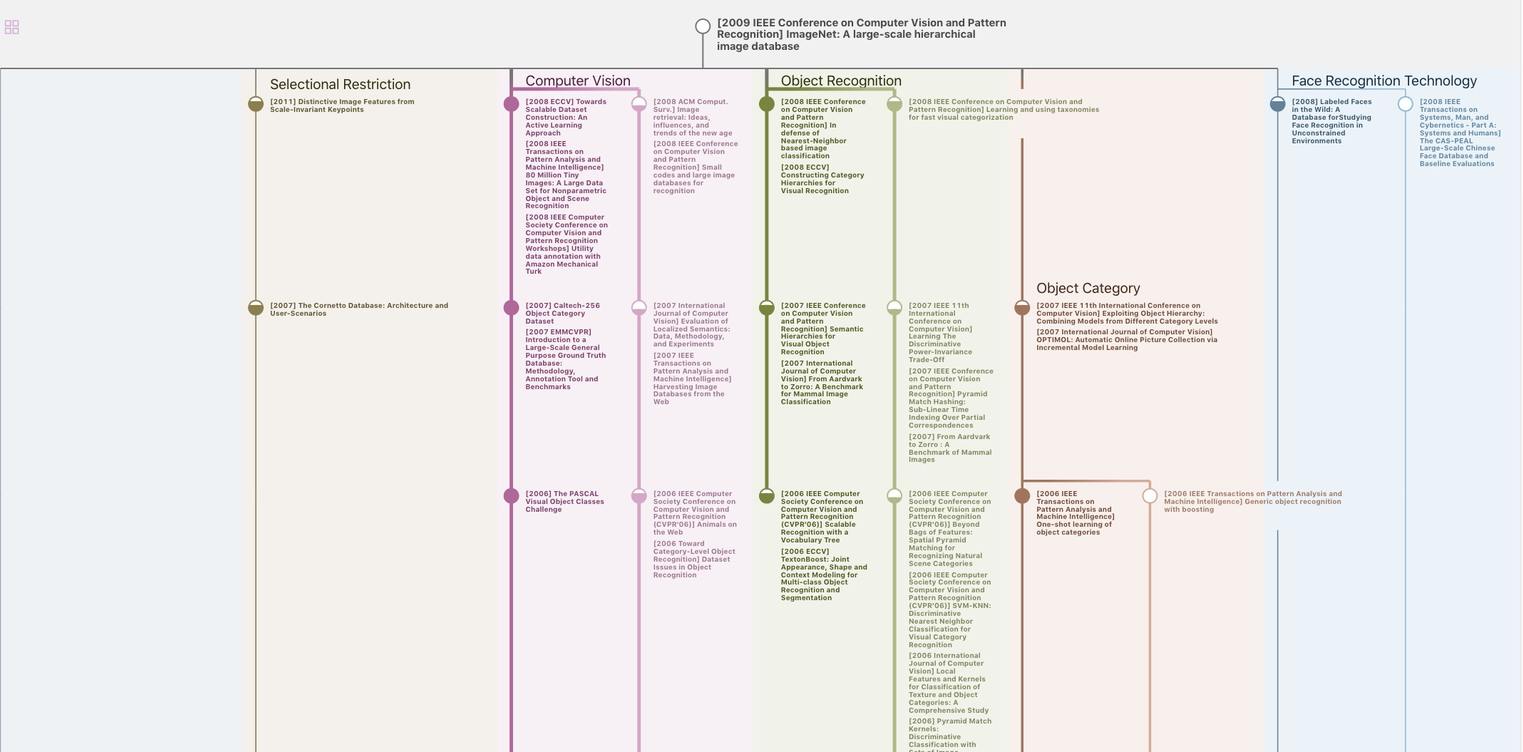
生成溯源树,研究论文发展脉络
Chat Paper
正在生成论文摘要