On the Communication Complexity of Decentralized Bilevel Optimization
CoRR(2023)
摘要
Decentralized bilevel optimization has been actively studied in the past few
years since it has widespread applications in machine learning. However,
existing algorithms suffer from large communication complexity caused by the
estimation of stochastic hypergradient, limiting their application to
real-world tasks. To address this issue, we develop a novel decentralized
stochastic bilevel gradient descent algorithm under the heterogeneous setting,
which enjoys a small communication cost in each round and small communication
rounds. As such, it can achieve a much better communication complexity than
existing algorithms. Moreover, we extend our algorithm to the more challenging
decentralized multi-level optimization. To the best of our knowledge, this is
the first time achieving these theoretical results under the heterogeneous
setting. At last, the experimental results confirm the efficacy of our
algorithm.
更多查看译文
AI 理解论文
溯源树
样例
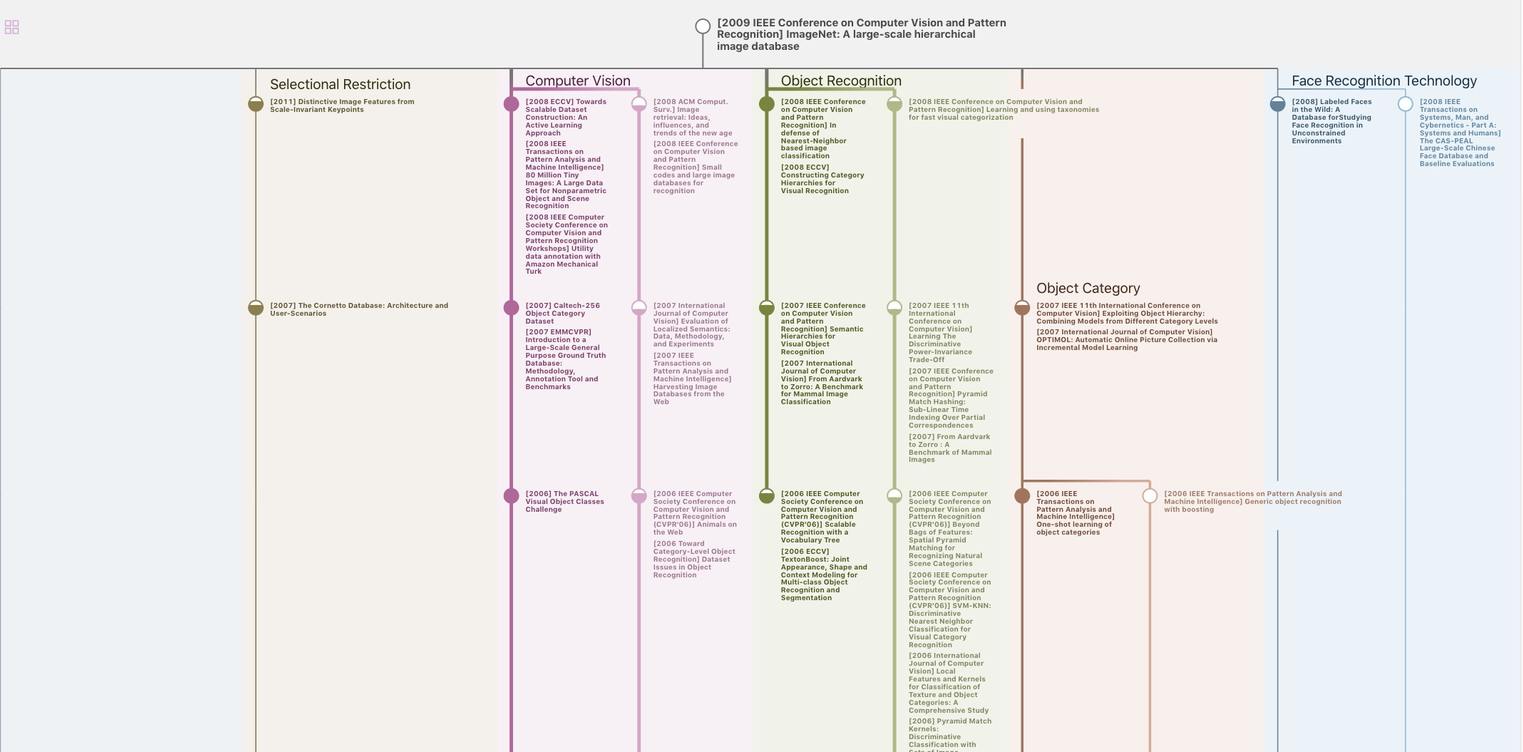
生成溯源树,研究论文发展脉络
Chat Paper
正在生成论文摘要