Submeter-level land cover mapping of Japan
INTERNATIONAL JOURNAL OF APPLIED EARTH OBSERVATION AND GEOINFORMATION(2024)
摘要
Deep learning has shown promising performance in submeter-level mapping tasks; however, its annotation cost remains a challenge, especially when applied on a large scale. In this paper, we introduce the first submeterlevel land cover mapping of Japan, employing eight classes. We present a human-in-the-loop framework that achieves national-scale mapping with a small amount of additional labeled data together with OpenEarthMap, a recently introduced benchmark dataset for global submeter-level land cover mapping. Using aerial imagery provided by the Geospatial Information Authority of Japan, we create land cover classification maps for the entire country of Japan and evaluate their accuracy. By adding a small amount of labeled data to areas where a U -Net model trained on OpenEarthMap clearly failed and retraining the model, an overall accuracy of 80% was achieved, which is a nearly 16 percentage point improvement after retraining. Our framework, with its low-cost and high-accuracy mapping results, demonstrates the potential to contribute to the automatic updating of land cover maps using submeter-level optical remote sensing data. The mapping results will be made publicly available.
更多查看译文
关键词
Land cover mapping,Very high resolution,Deep learning
AI 理解论文
溯源树
样例
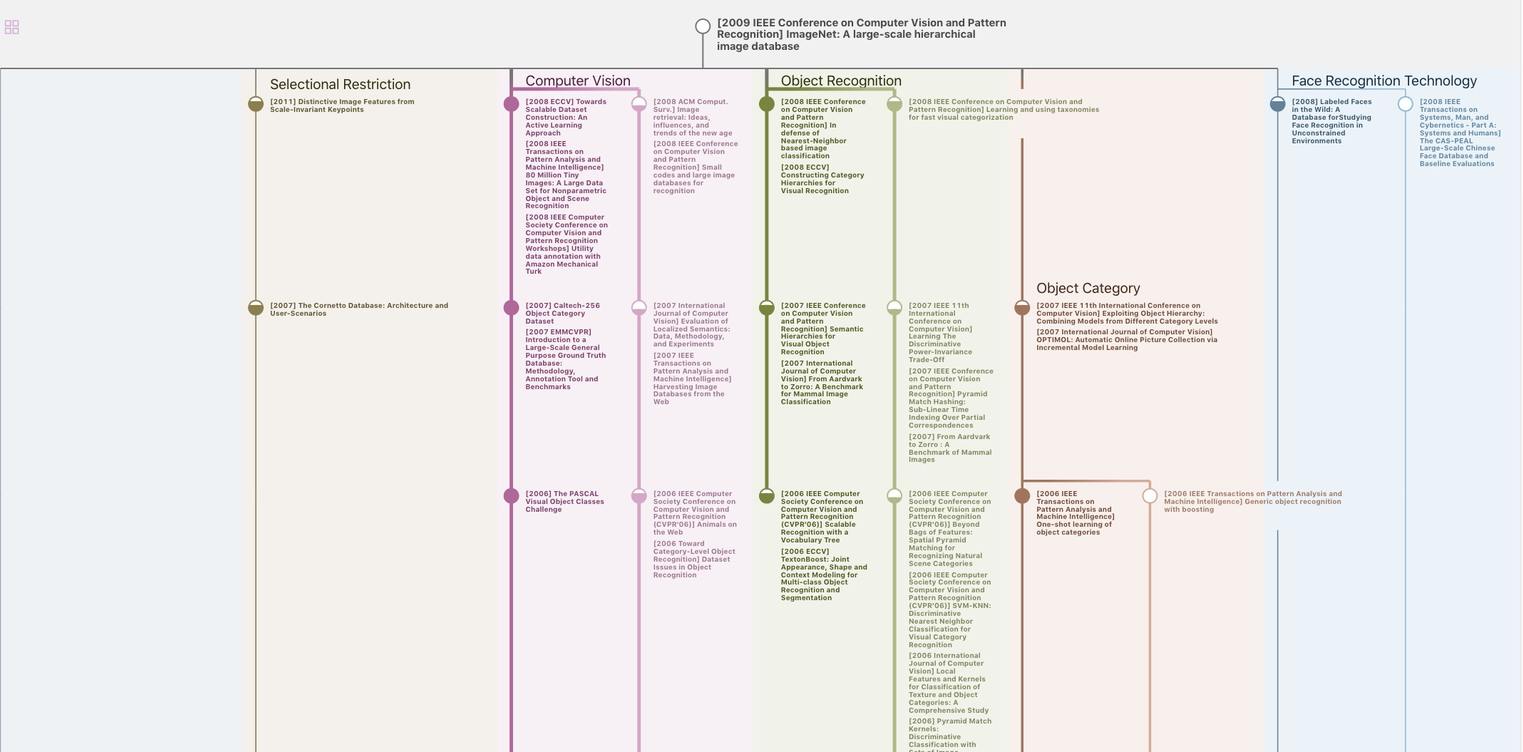
生成溯源树,研究论文发展脉络
Chat Paper
正在生成论文摘要