Unmasking and Improving Data Credibility: A Study with Datasets for Training Harmless Language Models
ICLR 2024(2023)
摘要
Language models have shown promise in various tasks but can be affected by
undesired data during training, fine-tuning, or alignment. For example, if some
unsafe conversations are wrongly annotated as safe ones, the model fine-tuned
on these samples may be harmful. Therefore, the correctness of annotations,
i.e., the credibility of the dataset, is important. This study focuses on the
credibility of real-world datasets, including the popular benchmarks Jigsaw
Civil Comments, Anthropic Harmless & Red Team, PKU BeaverTails & SafeRLHF, that
can be used for training a harmless language model. Given the cost and
difficulty of cleaning these datasets by humans, we introduce a systematic
framework for evaluating the credibility of datasets, identifying label errors,
and evaluating the influence of noisy labels in the curated language data,
specifically focusing on unsafe comments and conversation classification. With
the framework, we find and fix an average of 6.16% label errors in 11 datasets
constructed from the above benchmarks. The data credibility and downstream
learning performance can be remarkably improved by directly fixing label
errors, indicating the significance of cleaning existing real-world datasets.
Open-source: https://github.com/Docta-ai/docta.
更多查看译文
关键词
Label errors,dataset cleaning,AI safety,toxicity,harmless,language models
AI 理解论文
溯源树
样例
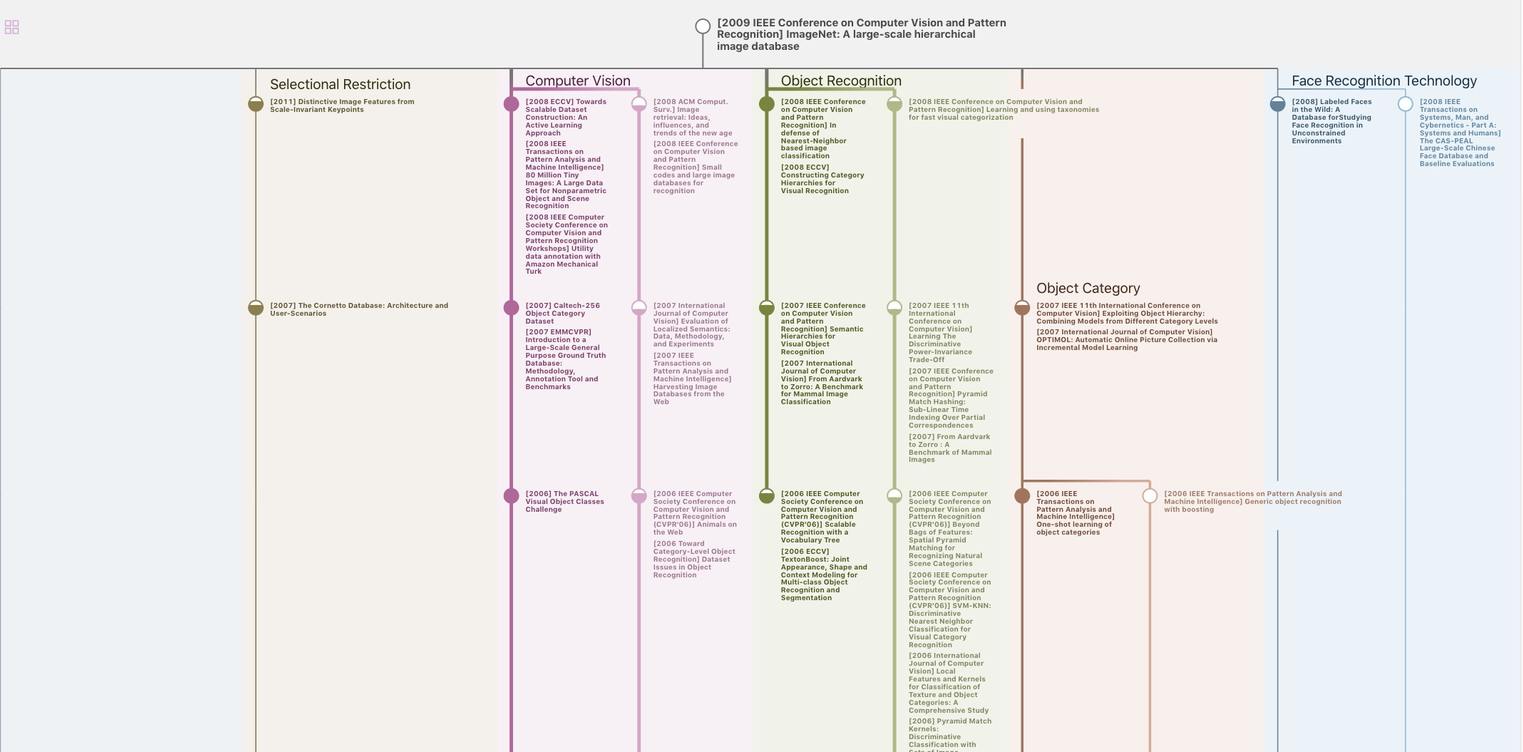
生成溯源树,研究论文发展脉络
Chat Paper
正在生成论文摘要