Advancing a Model of Students' Intentional Persistence in Machine Learning and Artificial Intelligence.
CoRR(2023)
摘要
Machine Learning (ML) and Artificial Intelligence (AI) are powering the
applications we use, the decisions we make, and the decisions made about us. We
have seen numerous examples of non-equitable outcomes, from facial recognition
algorithms to recidivism algorithms, when they are designed without diversity
in mind. Thus, we must take action to promote diversity among those in this
field. A critical step in this work is understanding why some students who
choose to study ML/AI later leave the field. While the persistence of diverse
populations has been studied in engineering, there is a lack of research
investigating factors that influence persistence in ML/AI. In this work, we
present the advancement of a model of intentional persistence in ML/AI by
surveying students in ML/AI courses. We examine persistence across demographic
groups, such as gender, international student status, student loan status, and
visible minority status. We investigate independent variables that distinguish
ML/AI from other STEM fields, such as the varying emphasis on non-technical
skills, the ambiguous ethical implications of the work, and the highly
competitive and lucrative nature of the field. Our findings suggest that
short-term intentional persistence is associated with academic enrollment
factors such as major and level of study. Long-term intentional persistence is
correlated with measures of professional role confidence. Unique to our study,
we show that wanting your work to have a positive social benefit is a negative
predictor of long-term intentional persistence, and women generally care more
about this. We provide recommendations to educators to meaningfully discuss
ML/AI ethics in classes and encourage the development of interpersonal skills
to help increase diversity in the field.
更多查看译文
AI 理解论文
溯源树
样例
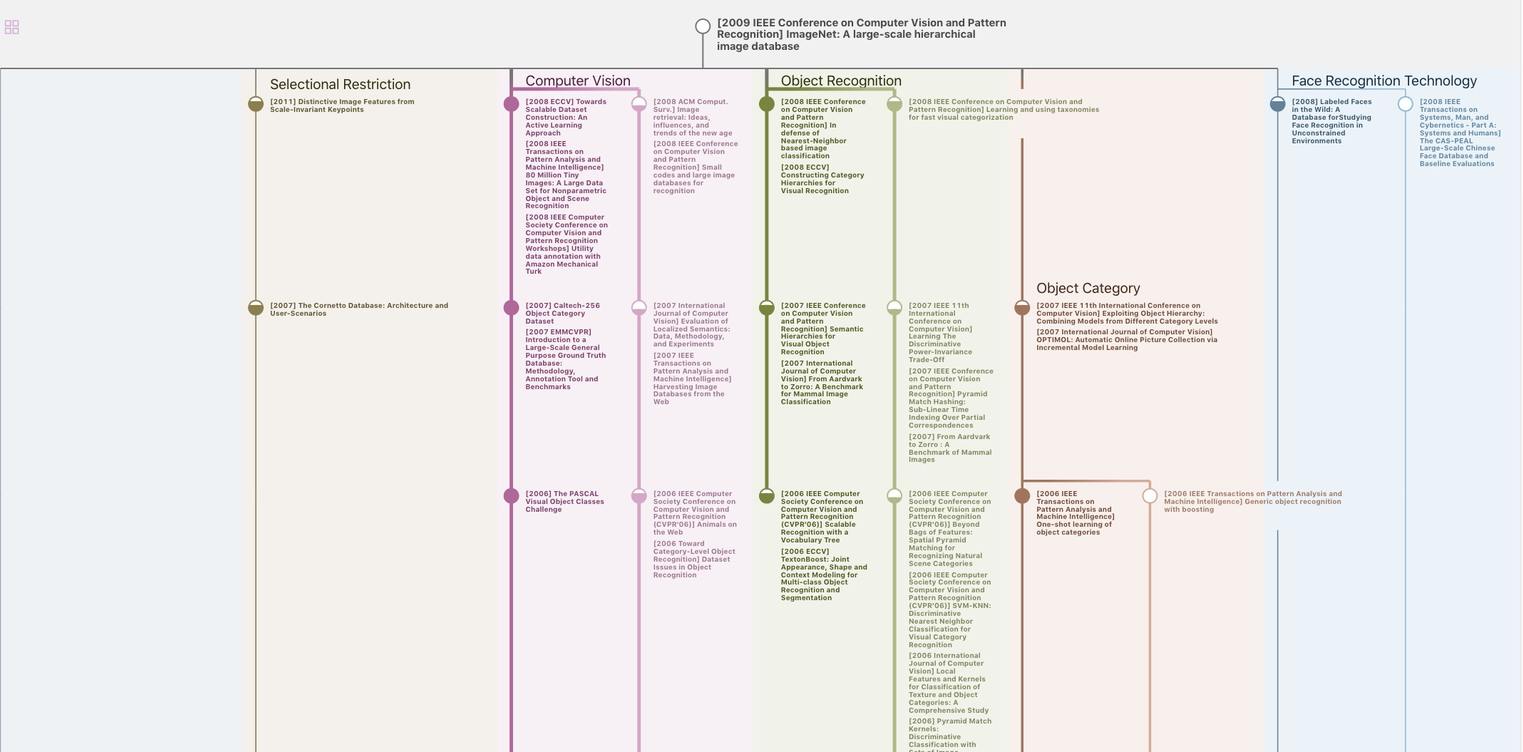
生成溯源树,研究论文发展脉络
Chat Paper
正在生成论文摘要