From Optimization to Control: Quasi Policy Iteration.
CoRR(2023)
摘要
Recent control algorithms for Markov decision processes (MDPs) have been
designed using an implicit analogy with well-established optimization
algorithms. In this paper, we make this analogy explicit across four problem
classes with a unified solution characterization. This novel framework, in
turn, allows for a systematic transformation of algorithms from one domain to
the other. In particular, we identify equivalent optimization and control
algorithms that have already been pointed out in the existing literature, but
mostly in a scattered way. With this unifying framework in mind, we then
exploit two linear structural constraints specific to MDPs for approximating
the Hessian in a second-order-type algorithm from optimization, namely,
Anderson mixing. This leads to a novel first-order control algorithm that
modifies the standard value iteration (VI) algorithm by incorporating two new
directions and adaptive step sizes. While the proposed algorithm, coined as
quasi-policy iteration, has the same computational complexity as VI, it
interestingly exhibits an empirical convergence behavior similar to policy
iteration with a very low sensitivity to the discount factor.
更多查看译文
AI 理解论文
溯源树
样例
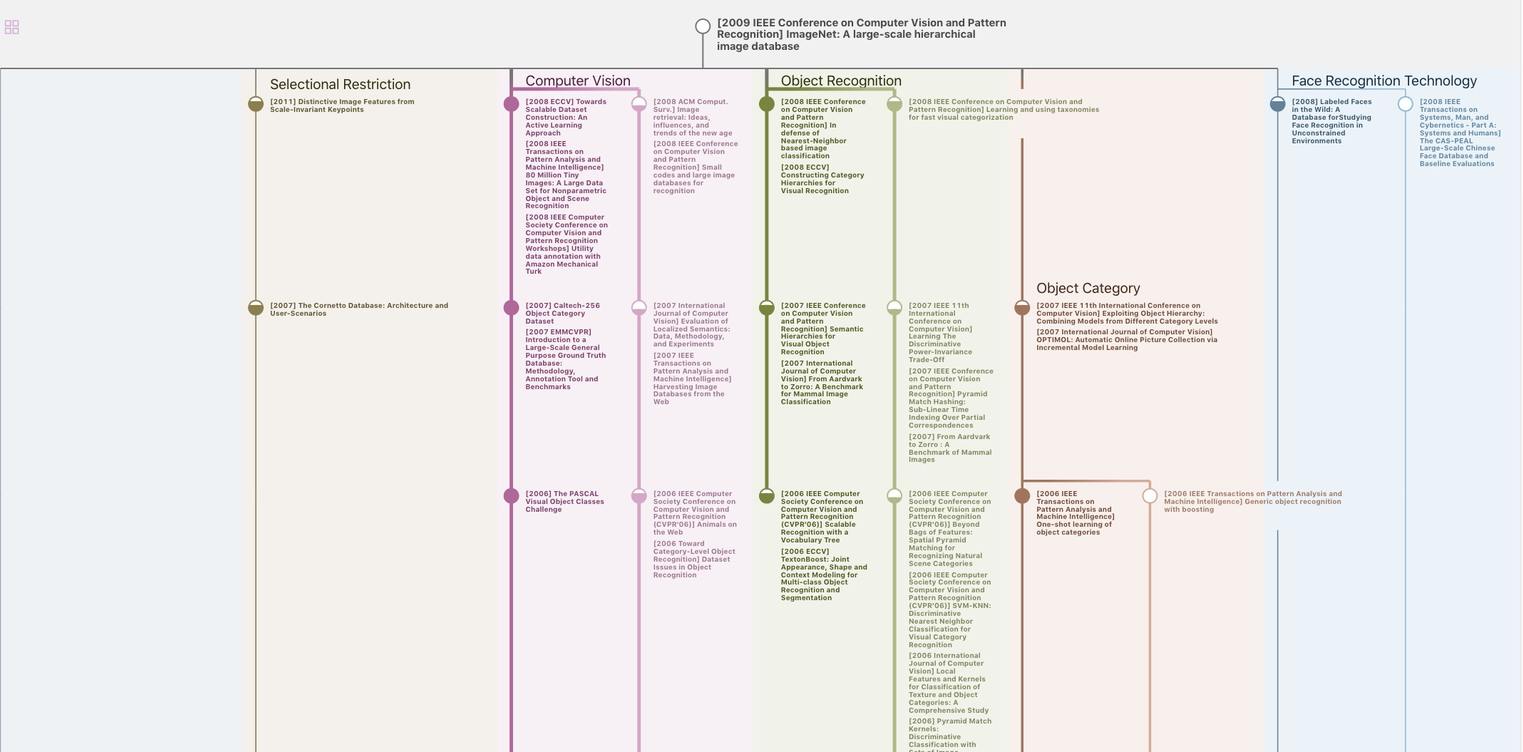
生成溯源树,研究论文发展脉络
Chat Paper
正在生成论文摘要