Classification of Radio Galaxies with trainable COSFIRE filters
CoRR(2023)
摘要
Radio galaxies exhibit a rich diversity of characteristics and emit radio emissions through a variety of radiation mechanisms, making their classification into distinct types based on morphology a complex challenge. To address this challenge effectively, we introduce an innovative approach for radio galaxy classification using COSFIRE filters. These filters possess the ability to adapt to both the shape and orientation of prototype patterns within images. The COSFIRE approach is explainable, learning-free, rotation-tolerant, efficient, and does not require a huge training set. To assess the efficacy of our method, we conducted experiments on a benchmark radio galaxy data set comprising of 1180 training samples and 404 test samples. Notably, our approach achieved an average accuracy rate of 93.36\%. This achievement outperforms contemporary deep learning models, and it is the best result ever achieved on this data set. Additionally, COSFIRE filters offer better computational performance, $\sim$20$\times$ fewer operations than the DenseNet-based competing method (when comparing at the same accuracy). Our findings underscore the effectiveness of the COSFIRE filter-based approach in addressing the complexities associated with radio galaxy classification. This research contributes to advancing the field by offering a robust solution that transcends the orientation challenges intrinsic to radio galaxy observations. Our method is versatile in that it is applicable to various image classification approaches.
更多查看译文
AI 理解论文
溯源树
样例
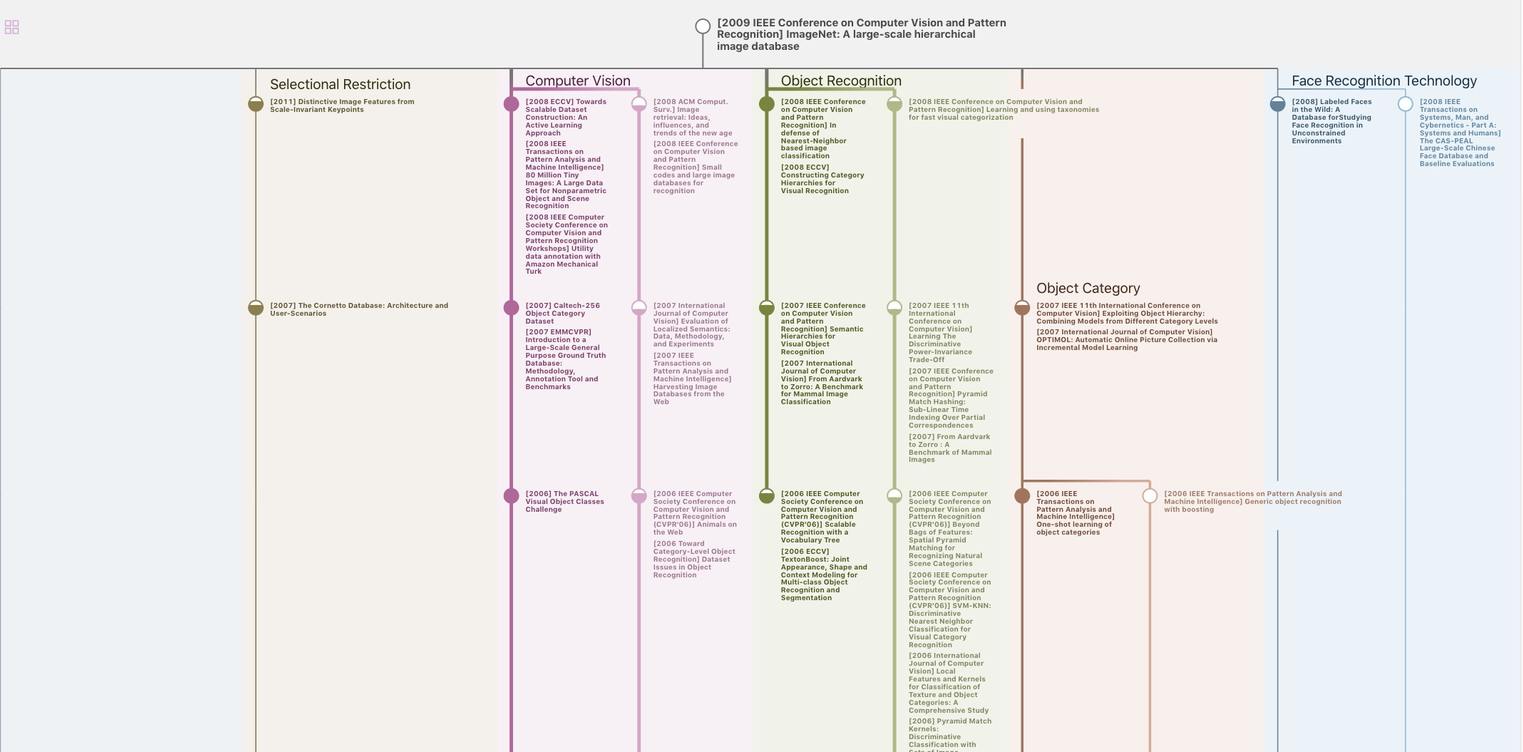
生成溯源树,研究论文发展脉络
Chat Paper
正在生成论文摘要