Data Convection: A GPU-Driven Case Study for Thermal-Aware Data Placement in 3D DRAMs
ACM SIGMETRICS Performance Evaluation Review(2022)
摘要
Stacked DRAMs have been studied and productized in the last decade. The large available bandwidth they offer makes them an attractive choice, particularly, in high-performance computing (HPC) environments. Consequently, many prior research efforts have studied and evaluated 3D stacked DRAM-based designs. Despite offering high bandwidth, stacked DRAMs are severely constrained by the overall memory capacity offered. In this paper, we study and evaluate integrating stacked DRAM on top of a GPU in a 3D manner which in tandem with the 2.5D stacked DRAM boosts the capacity and the bandwidth without increasing the package size. It also helps meet the capacity needs of emergent workloads like deep learning. However, the bandwidth given by these 3D stacked DRAMs is significantly constrained by the GPU's heat production. Our investigations on a cycle-level simulator show that the 3D stacked DRAM portions closest to the GPU have shorter retention times than the layers further away. Depending on the retention period, certain regions of 3D stacked DRAM are refreshed more frequently than others, leading to thermally-induced NUMA paradigms. Our proposed approach attempts to place the most frequently requested data in a thermally conscious manner, taking into consideration both bank-level parallelism and channel-level parallelism. The results collected with a cycle-level GPU simulator indicate that the three implementations of our proposed approach lead to 1.8%, 11.7%, and 14.4% performance improvements, over a baseline that already includes 3D+2.5D stacked DRAMs.
更多查看译文
AI 理解论文
溯源树
样例
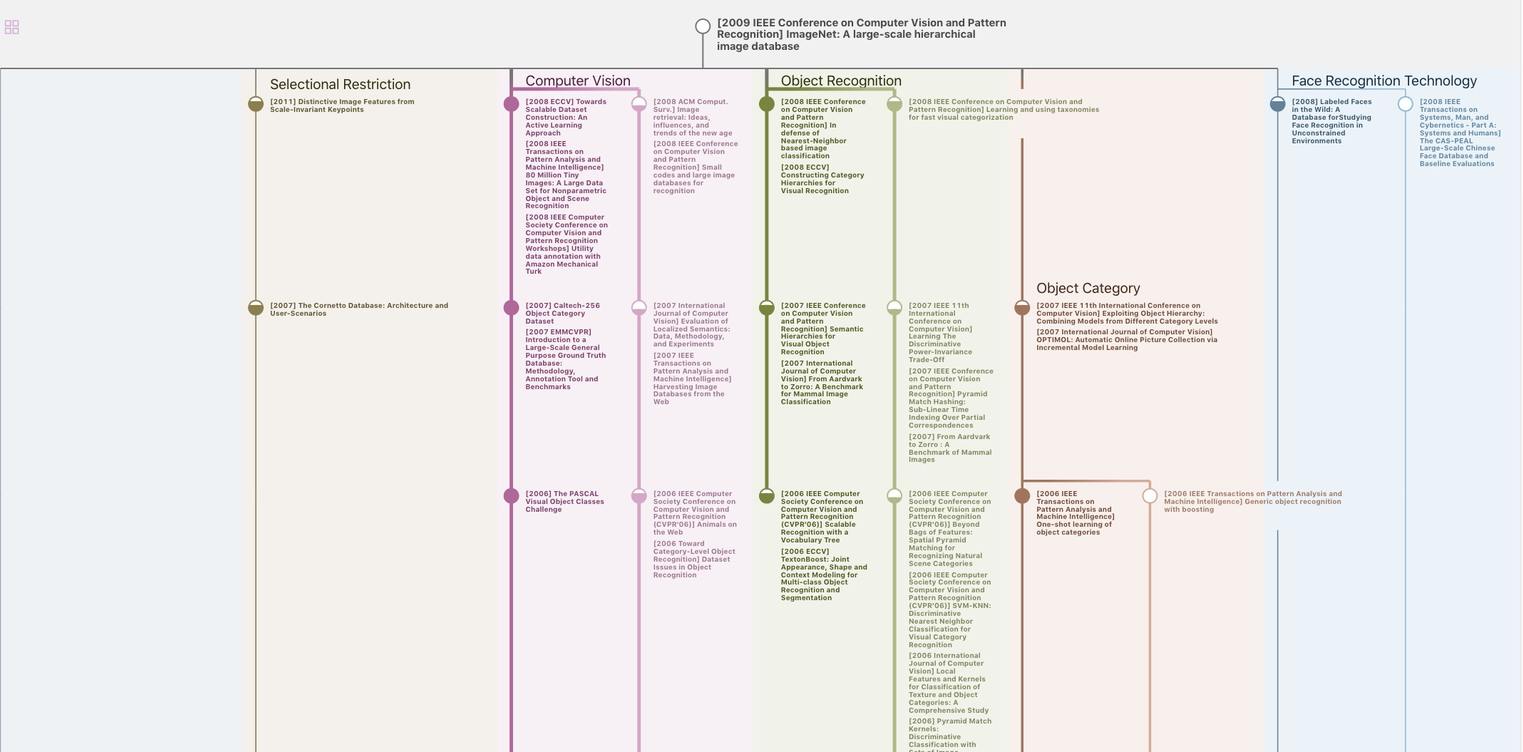
生成溯源树,研究论文发展脉络
Chat Paper
正在生成论文摘要