Differential Privacy Under Membership Inference Attacks.
Future Data and Security Engineering. Big Data, Security and Privacy, Smart City and Industry 4.0 Applications Communications in Computer and Information Science(2023)
摘要
Membership inference attacks are used as an audit tool to quantify training data leaks in machine learning models. Protection can be provided by anonymizing the training data or using training functions with differential privacy. Depending on the context, such as building data collection services for central machine learning models or responding to queries from end users, data scientists can choose between local and global differential privacy parameters. Different types of differential privacy have different epsilon values that reflect different mechanisms, making it difficult for data scientists to select appropriate differential privacy parameters and avoid inaccurate conclusions. The experiments in this paper show the relative privacy-accuracy trade-off of local and global differential privacy mechanisms under a white-box membership inference attack. While membership inference only reflects the lower bound for inference risk, and differential privacy formulates the upper bound, the experiments in this study with some datasets show that the trade-off between accuracy and privacy is similar for both types of mechanisms, although there is a large difference in their upper bounds. This suggests that the upper bound is far from the practical susceptibility to membership inference. Therefore, a small epsilon value in global differential privacy and a large epsilon value in local differential privacy lead to the same risk of membership inference. In addition, the risks from membership inference attacks are not uniform across all classes, especially when the training dataset in machine learning models is skewed.
更多查看译文
AI 理解论文
溯源树
样例
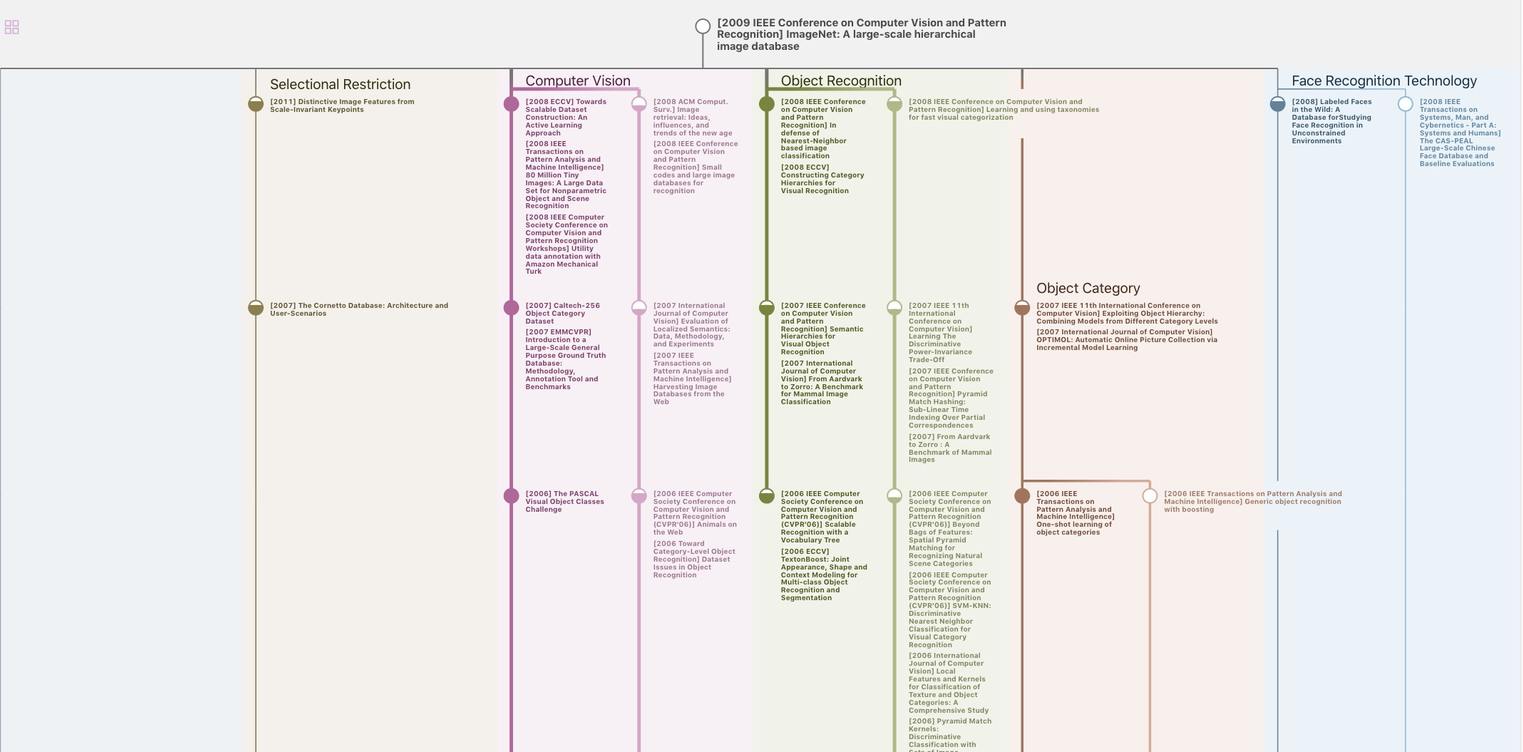
生成溯源树,研究论文发展脉络
Chat Paper
正在生成论文摘要