Labeled Interactive Topic Models.
CoRR(2023)
摘要
Topic models help users understand large document collections; however, topic
models do not always find the ``right'' topics. While classical probabilistic
and anchor-based topic models have interactive variants to guide models toward
better topics, such interactions are not available for neural topic models such
as the embedded topic model (\abr{etm}). We correct this lacuna by adding an
intuitive interaction to neural topic models: users can label a topic with a
word, and topics are updated so that the topic words are close to the label.
This allows a user to refine topics based on their information need. While,
interactivity is intuitive for \abr{etm}, we extend this framework to work with
other neural topic models as well. We develop an interactive interface which
allows users to interact and relabel topic models as they see fit. We evaluate
our method through a human study, where users can relabel topics to find
relevant documents. Using our method, user labeling improves document rank
scores, helping to find more relevant documents to a given query when compared
to no user labeling.
更多查看译文
AI 理解论文
溯源树
样例
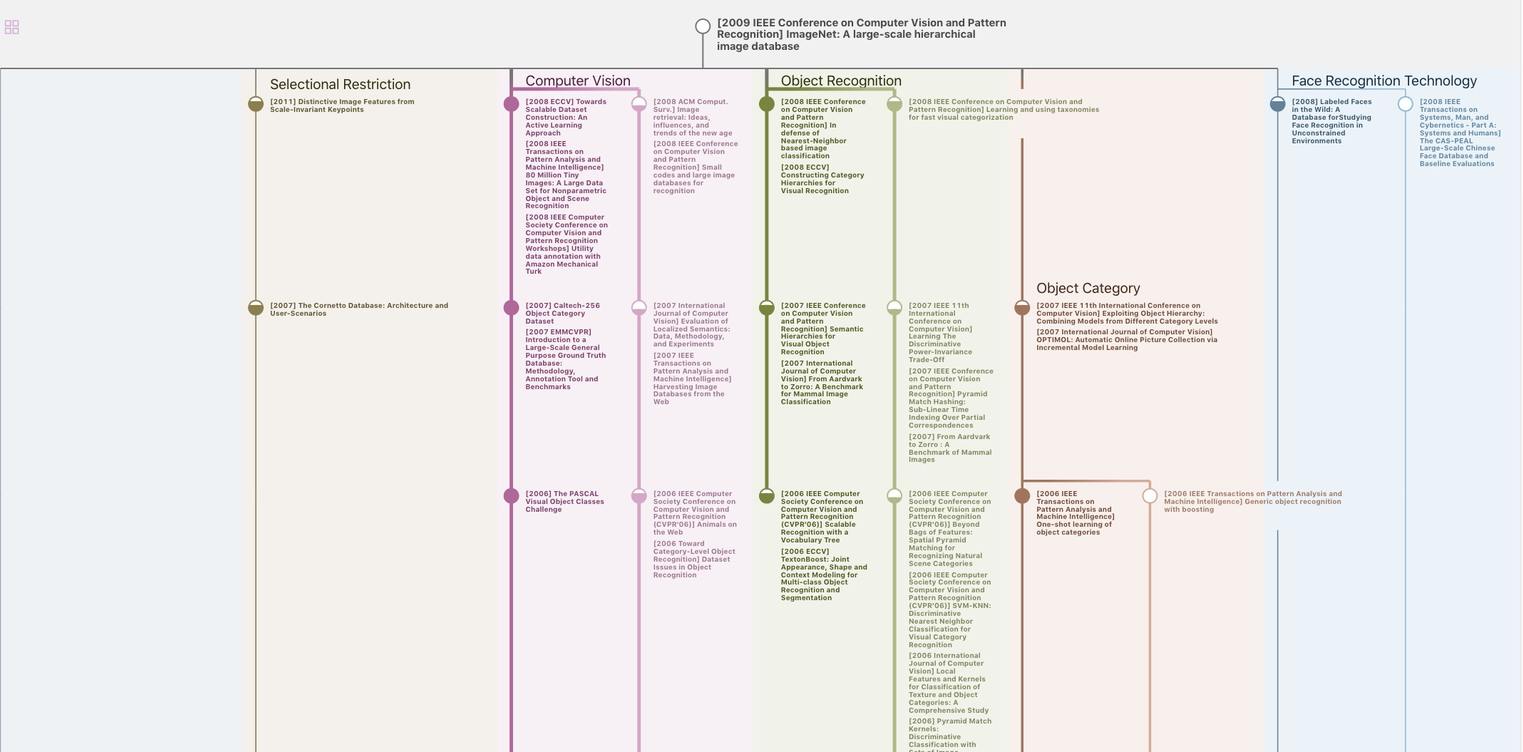
生成溯源树,研究论文发展脉络
Chat Paper
正在生成论文摘要