Leveraging Citizen Science for Flood Extent Detection using Machine Learning Benchmark Dataset.
CoRR(2023)
摘要
Accurate detection of inundated water extents during flooding events is crucial in emergency response decisions and aids in recovery efforts. Satellite Remote Sensing data provides a global framework for detecting flooding extents. Specifically, Sentinel-1 C-Band Synthetic Aperture Radar (SAR) imagery has proven to be useful in detecting water bodies due to low backscatter of water features in both co-polarized and cross-polarized SAR imagery. However, increased backscatter can be observed in certain flooded regions such as presence of infrastructure and trees - rendering simple methods such as pixel intensity thresholding and time-series differencing inadequate. Machine Learning techniques has been leveraged to precisely capture flood extents in flooded areas with bumps in backscatter but needs high amounts of labelled data to work desirably. Hence, we created a labeled known water body extent and flooded area extents during known flooding events covering about 36,000 sq. kilometers of regions within mainland U.S and Bangladesh. Further, We also leveraged citizen science by open-sourcing the dataset and hosting an open competition based on the dataset to rapidly prototype flood extent detection using community generated models. In this paper we present the information about the dataset, the data processing pipeline, a baseline model and the details about the competition, along with discussion on winning approaches. We believe the dataset adds to already existing datasets based on Sentinel-1C SAR data and leads to more robust modeling of flood extents. We also hope the results from the competition pushes the research in flood extent detection further.
更多查看译文
AI 理解论文
溯源树
样例
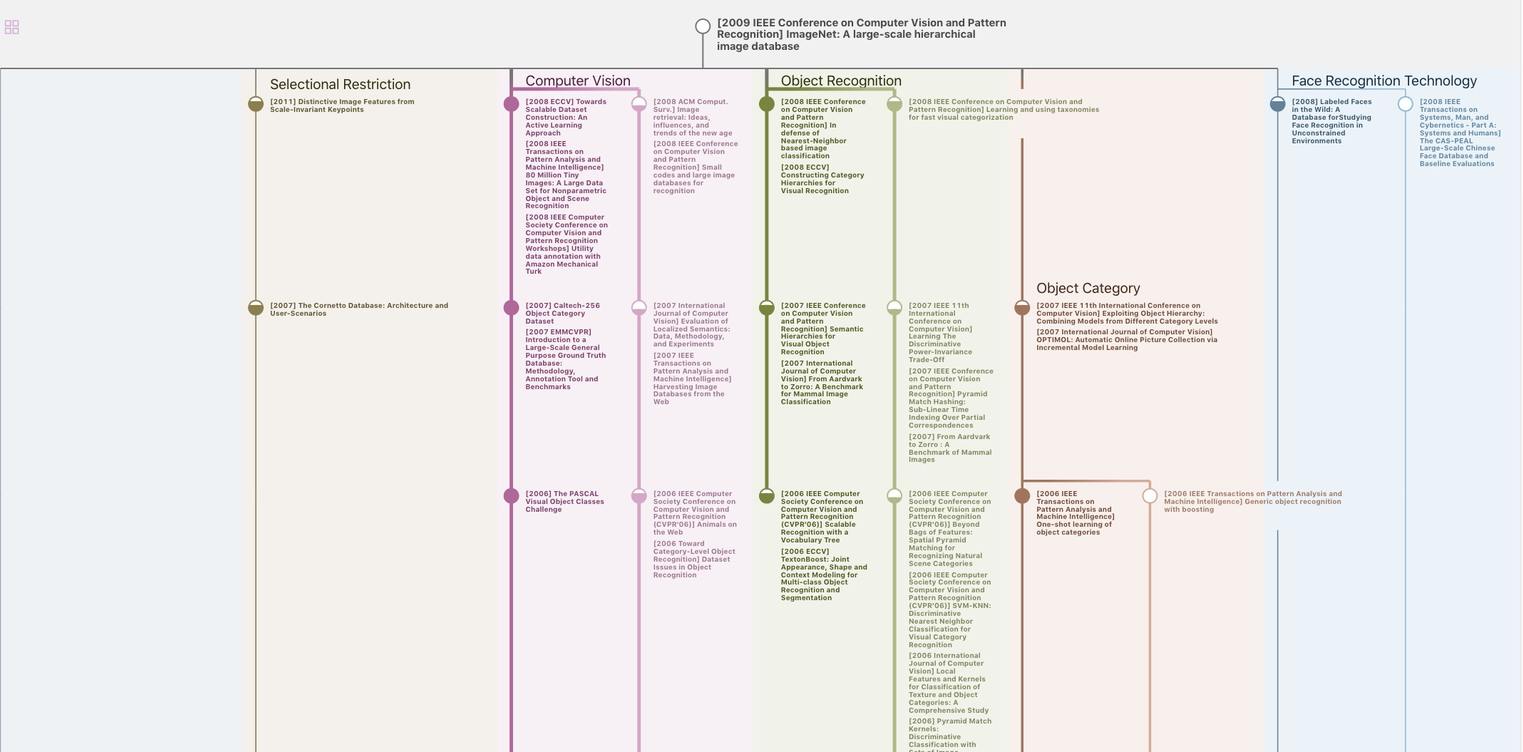
生成溯源树,研究论文发展脉络
Chat Paper
正在生成论文摘要