Multi-objective Quantum Annealing approach for solving flexible job shop scheduling in manufacturing
JOURNAL OF MANUFACTURING SYSTEMS(2024)
摘要
Flexible Job Shop Scheduling (FJSSP) is a challenging optimization problem with multiple conflicting objectives used to model and compute real-world process scheduling tasks. In order for a manufacturing system to remain competitive, it is necessary to compute such optimization problems quickly and efficiently. The limitations of conventional optimization methods frequently stem from a delicate balance between solution quality and computation time. Consequently, a pressing need for solution algorithms arises that can effectively transcend these limitations. This paper presents a novel Quantum Annealing-based solving algorithm (QASA) for computing FJSSP, leveraging the power of Quantum Annealing combined with classical techniques. The proposed approach aims to optimize a multi-criterial FJSSP considering makespan, total workload, and job priority simultaneously. QASA employs a Hamiltonian formulation with Lagrange parameters to integrate the problem's constraints and objectives. By assigning appropriate weights to the objectives, the method allows the prioritization of certain objectives over others. To handle the computational complexity of large FJSSP instances, the problem is decomposed into smaller subproblems, and a decision logic based on bottleneck factors is employed to select critical jobs for computation combined with variable pruning techniques. To evaluate the effectiveness of the proposed approach, experiments are conducted on benchmark problems, considering makespan, total workload, and priority objectives. Therefore, QASA combining tabu search, simulated annealing, and Quantum Annealing is used for efficient computation and is compared with a classical solving algorithm (CSA) combining tabu search and simulated annealing. The results demonstrate that QASA outperforms CSA in terms of solution quality, as measured by set coverage and hypervolume ratio metrics. Furthermore, computational efficiency analysis reveals that QASA achieves superior Pareto solutions compared to the classical approach, with a reasonable increase in computation time.
更多查看译文
关键词
Process scheduling,Job shop scheduling,Multi -objective optimization,Binary quadratic model (BQM),Quantum Annealing
AI 理解论文
溯源树
样例
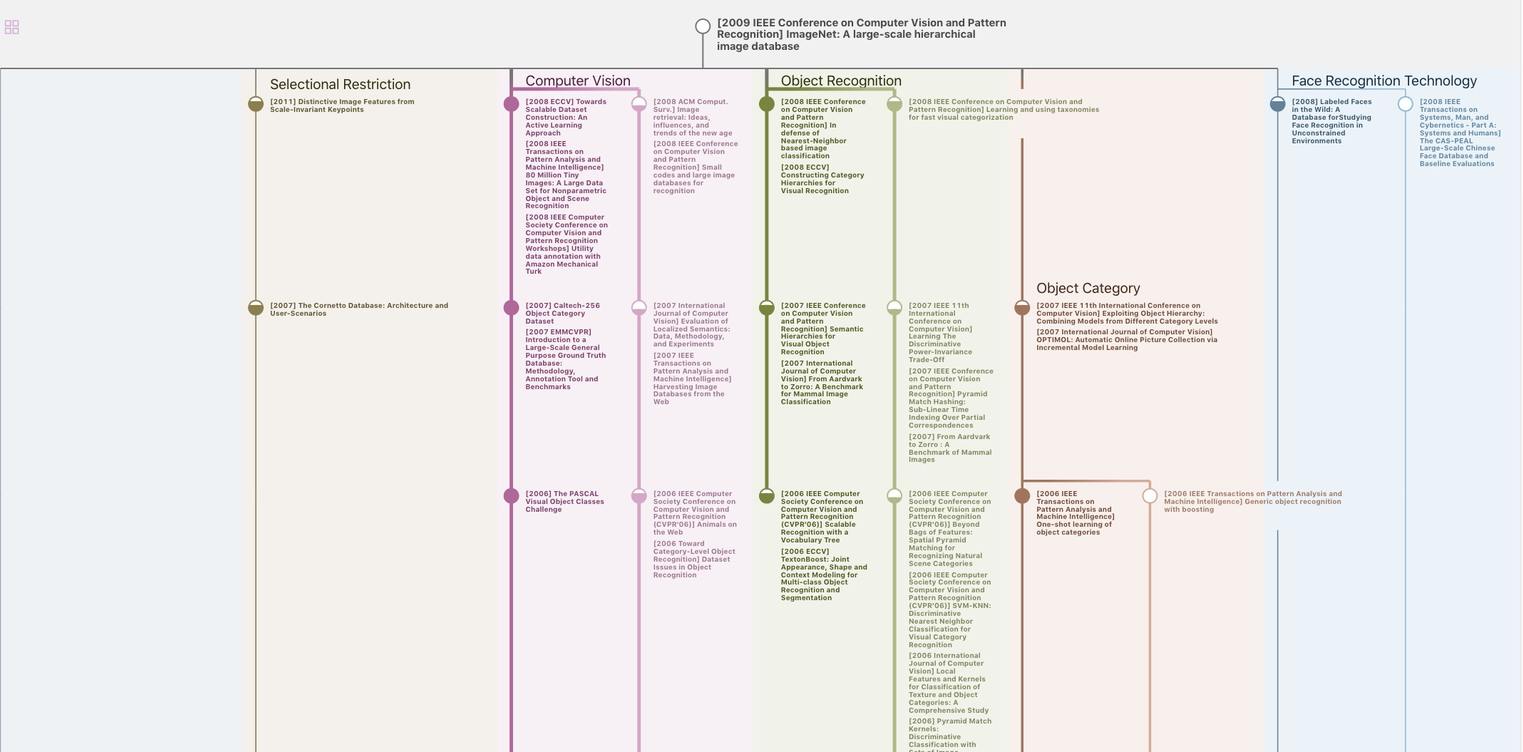
生成溯源树,研究论文发展脉络
Chat Paper
正在生成论文摘要