Purpose in the Machine: Do Traffic Simulators Produce Distributionally Equivalent Outcomes for Reinforcement Learning Applications?
2023 Winter Simulation Conference (WSC)(2023)
摘要
Traffic simulators are used to generate data for learning in intelligent transportation systems (ITSs). A key question is to what extent their modelling assumptions affect the capabilities of ITSs to adapt to various scenarios when deployed in the real world. This work focuses on two simulators commonly used to train reinforcement learning (RL) agents for traffic applications, CityFlow and SUMO. A controlled virtual experiment varying driver behavior and simulation scale finds evidence against distributional equivalence in RL-relevant measures from these simulators, with the root mean squared error and KL divergence being significantly greater than 0 for all assessed measures. While granular real-world validation generally remains infeasible, these findings suggest that traffic simulators are not a deus ex machina for RL training: understanding the impacts of inter-simulator differences is necessary to train and deploy RL-based ITSs.
更多查看译文
关键词
Traffic Simulation,Divergence,Root Mean Square Error,Mean Square Error,Learning Data,Intelligent Transportation Systems,Driver Behavior,Travel Time,Real-world Data,Light Signal,Road Network,Pairwise Interactions,Real-world Studies,Traffic Control,Reinforcement Learning Algorithm,Maximum Acceleration,Veridical,Cellular Automata,Observation Space,Lane Change,Lead Vehicle,Minor Roads,Traffic Demand,Queue Length,Experiments In This Work,Minimum Gap,Maximum Deceleration,Divergence Measure,Discrete Time Steps,Traffic Flow
AI 理解论文
溯源树
样例
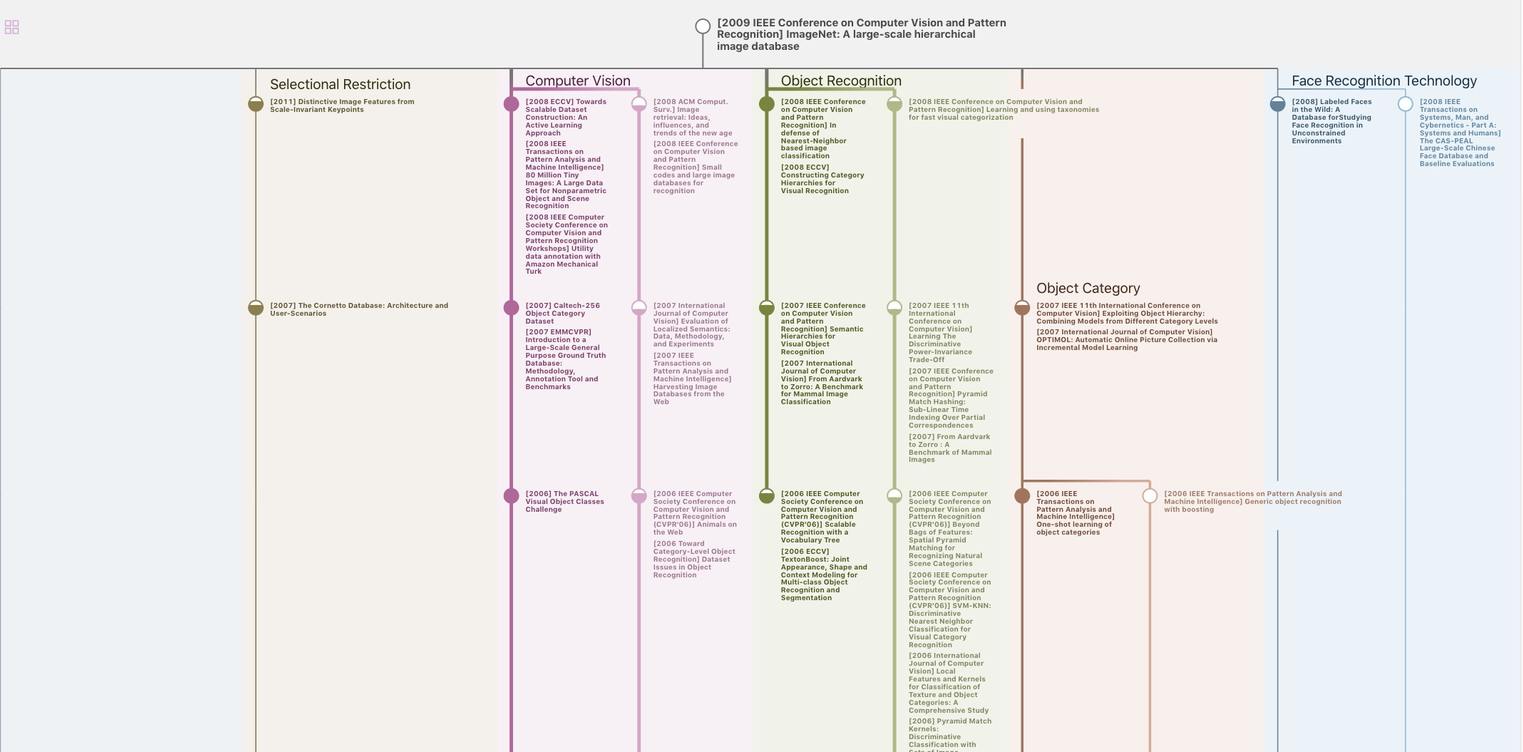
生成溯源树,研究论文发展脉络
Chat Paper
正在生成论文摘要