Joint Embedding of Food Photographs and Blood Glucose for Improved Calorie Estimation
2023 IEEE EMBS International Conference on Biomedical and Health Informatics (BHI)(2023)
摘要
Type 2 diabetes has a significant impact on individuals' health and well-being, and diet monitoring is an important tool in treating individuals. Accurate estimation of meal intake is essential for promoting diet and behavior interventions. While continuous glucose models (CGMs) have demonstrated the ability to estimate carbohydrate quantities in meals, CGMs-alone have been insufficient in capturing other meal nutritional information due to the different types of food and people's health conditions. Therefore, we propose a multi-modality model for augmenting CGM-based inverse metabolic models by using both CGM-captured interstitial glucose data and food image data. A late fusion approach is used to aggregate the extracted glucose information from the attention-based Transformer and Gaussian area under the curve (gAUC) features, and image information from the vision transformer. We test our model on a dataset collecting Freestyle Libre Pro CGM data and meal photographs of breakfasts and lunches on 27 participants, with meals with known fixed caloric content. Our joint embedded approach to learning calorie estimations from both CGM data and image data achieved an average Normalized Root Mean Squared Error (NRMSE) of 0.34 for calorie prediction, with a correlation of 0.52, a 15.0% improvement over CGM-only models and 17.1% over image-only models.
更多查看译文
关键词
Machine learning,Continuous Glucose Monitors,Diabetes,Diet monitoring,Nutrition
AI 理解论文
溯源树
样例
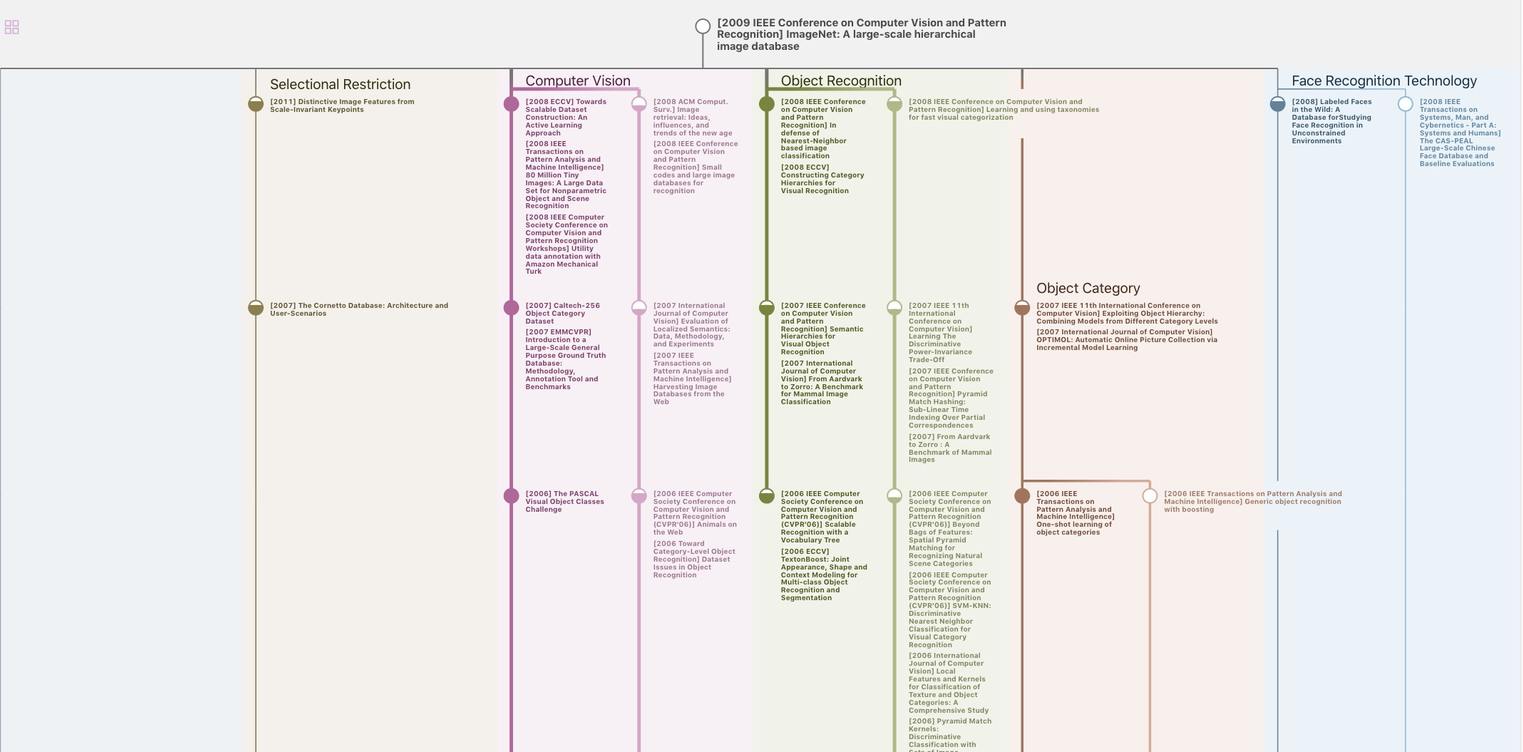
生成溯源树,研究论文发展脉络
Chat Paper
正在生成论文摘要