Enhanced Hybrid Hierarchical Federated Edge Learning Over Heterogeneous Networks
IEEE TRANSACTIONS ON VEHICULAR TECHNOLOGY(2023)
摘要
In this work, a Hybrid Hierarchical Federated Edge Learning (HHFEL) architecture that consists of a device layer, an edge layer, and a cloud layer over heterogeneous networks, is investigated for large-scale model training. In such systems, learning efficiency is severely degraded by limited communication resources and device heterogeneity in terms of local data distribution and computation capability, especially for synchronous FL mechanisms where the training of each round should wait for the slowest device. To tackle this issue, asynchronous FL is proposed, which allows the devices with powerful computation and communication capabilities exchanging information with the server more frequently. However, this asynchronous FL framework faces a new challenge of low accuracy caused by the imbalanced local model updating. To overcome the shortage of both synchronous and asynchronous FLs, we propose an enhanced online semi-asynchronous FL mechanism between the edge-device layers, where each device trains its local model with the newly generated data and each edge server aggregates a number of local models based on their arrival order in each round. Particularly, devices with faster training speeds would fully utilize the idle time by training their local models repetitively. Meanwhile, synchronous FL with an edge elastic update strategy is adopted to the cloud-edge layers for personalized information exchange. Considering the continuous data generation feature, we formulate the objective problem as an online Markov Decision Process (MDP) to realize efficient communication-and-computing HHFEL via joint device selection and resource allocation. Due to the non-convex and combinatorial problem structure, we develop a hybrid Deep Q-Network (DQN) and Deep Deterministic Policy Gradient (DDPG) approach with low computational complexity to adapt the device selection and resource allocation strategies. Numerical results show the effectiveness of the proposed mechanism compared with existing benchmarks.
更多查看译文
关键词
Training,Servers,Data models,Computational modeling,Resource management,Heterogeneous networks,Computer architecture,Edge computing,federated edge learning,hierarchical systems,semi-asynchronous
AI 理解论文
溯源树
样例
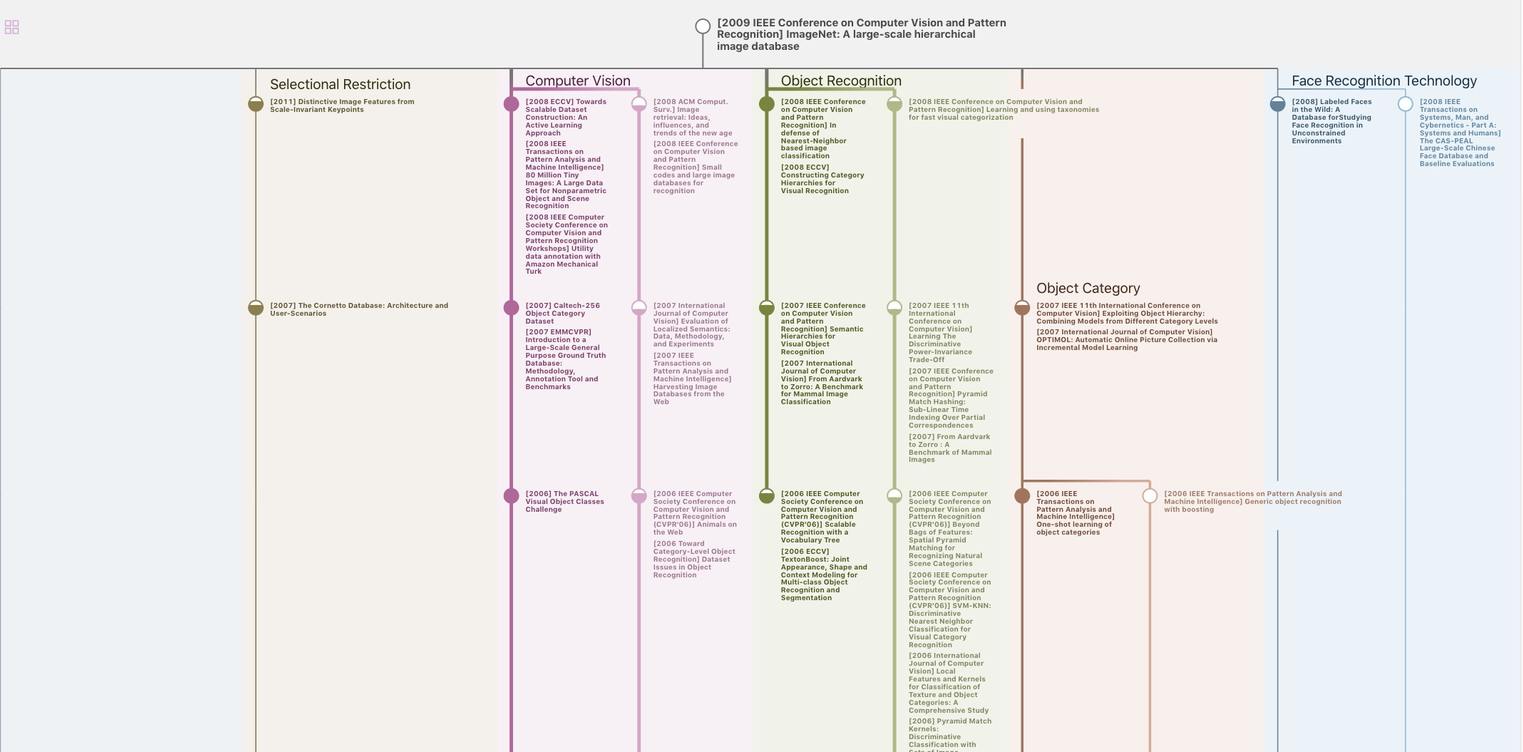
生成溯源树,研究论文发展脉络
Chat Paper
正在生成论文摘要