A Multisource Data Fusion-based Heterogeneous Graph Attention Network for Competitor Prediction
ACM TRANSACTIONS ON KNOWLEDGE DISCOVERY FROM DATA(2024)
摘要
Competitor identification is an essential component of corporate strategy. With the rapid development of artificial intelligence, various data-mining methodologies and frameworks have emerged to identify competitors. In general, the competitiveness among companies is determined by both market commonality and resource similarity. However, because resource information is more difficult to obtain than market information, existing studies primarily identify competitors via market commonality. To address this limitation, we introduce multisource company descriptions as well as heterogeneous business relationships, and we propose a novel method for simultaneously mining themarket commonality and resource similarity. First, we use multisource company descriptions to represent companies and transform the heterogeneous business relationships into a heterogeneous business network. Then, we propose a novel multisource data fusion-based heterogeneous graph attention network (MHGAT) to learn the pairwise competitive relationships between companies. Specifically, a graph neural network-based model is proposed to learn the embeddings of companies by preserving their competition, and a multilevel attention framework is designed to integrate the embeddings from neighboring company level, heterogeneous relationship level, and multisource description level. Finally, experiments on a real-world dataset verify the effectiveness of our proposed MHGAT and demonstrate the usefulness of company descriptions and business relationships in competitor identification.
更多查看译文
关键词
Competitor identification,business intelligence,business data,graph neural network
AI 理解论文
溯源树
样例
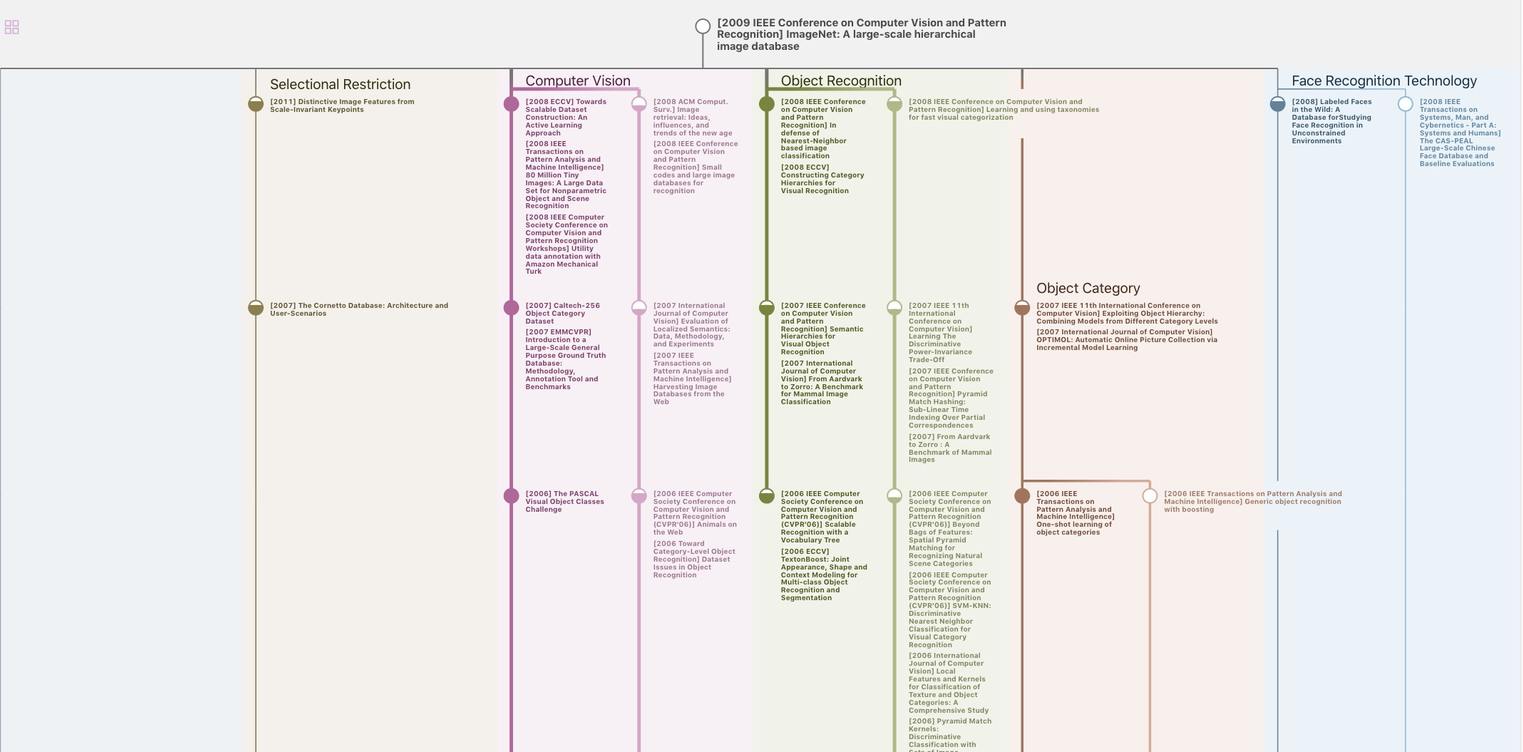
生成溯源树,研究论文发展脉络
Chat Paper
正在生成论文摘要