One General Teacher for Multi-Data Multi-Task: A New Knowledge Distillation Framework for Discourse Relation Analysis
IEEE-ACM TRANSACTIONS ON AUDIO SPEECH AND LANGUAGE PROCESSING(2024)
摘要
Automatically identifying the discourse relations can help many downstream NLP tasks such as reading comprehension and machine translation. It can be categorized into explicit and implicit discourse relation recognition (EDRR and IDRR). Due to the lack of connectives, IDRR remains to be a big challenge. A good number of methods have been developed to combine explicit data with implicit ones under the multi-task learning framework. However, the difference in linguistic property and class distribution makes it hard to directly optimize EDRR and IDRR with multi-task learning. In this paper, we take the first step to exploit the knowledge distillation (KD) technique for discourse relation analysis. Our target is to train a focused single-data single-task student with the help of a general multi-data multi-task teacher. Specifically, we first train one teacher for both the top and second level relation classification tasks with explicit and implicit data. We then transfer the feature embeddings and soft labels from the teacher network to the student network. Moreover, we develop an adaptive knowledge distillation module to reduce the number of hyper-parameters and also to stimulate the potential of the student on autonomous learning. Extensive experimental results on the popular PDTB dataset proves that our model achieves a new state-of-the-art performance. We also show the effectiveness of our proposed KD architecture through detailed analysis.
更多查看译文
关键词
Discourse relation analysis,knowledge distillation
AI 理解论文
溯源树
样例
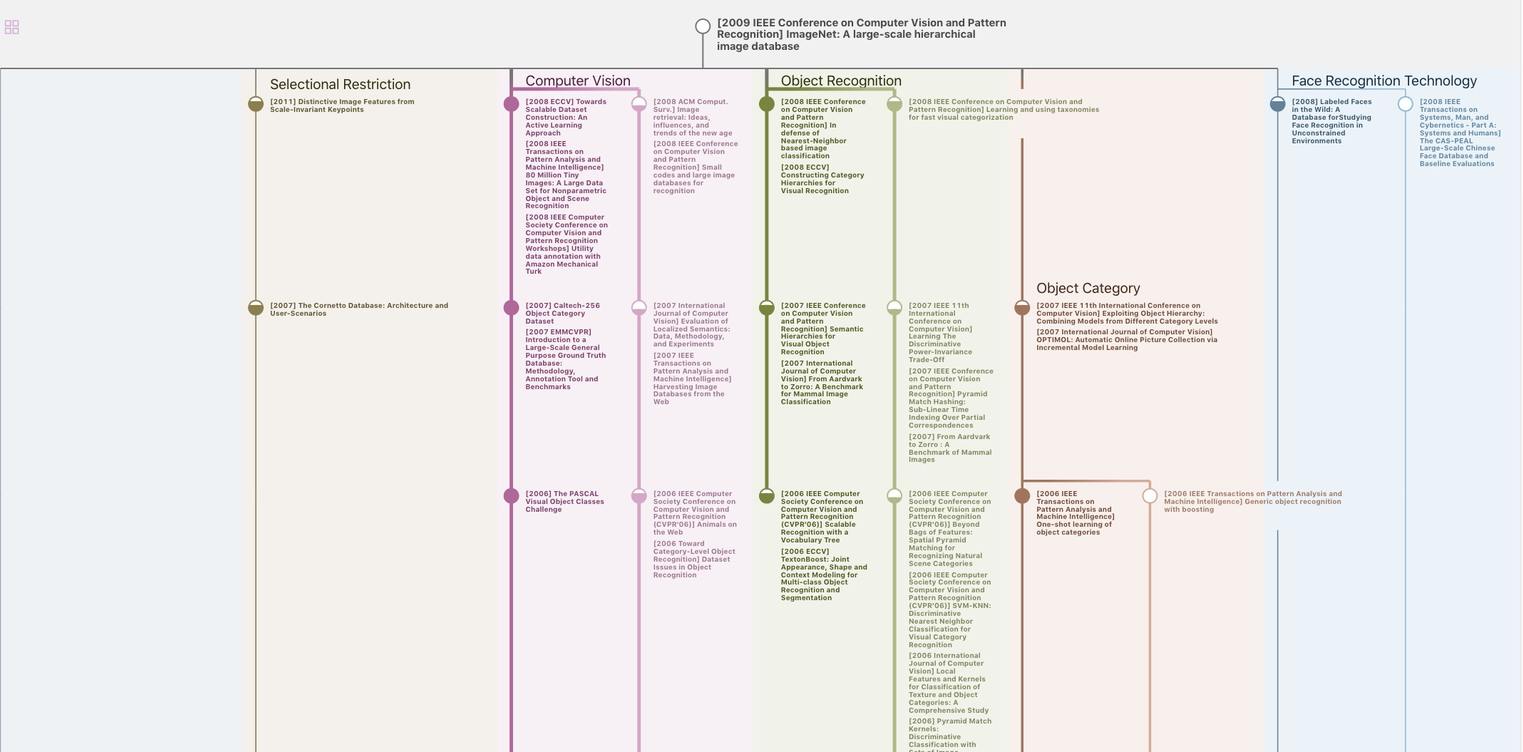
生成溯源树,研究论文发展脉络
Chat Paper
正在生成论文摘要