Generative, High-Fidelity Network Traces
PROCEEDINGS OF THE 22ND ACM WORKSHOP ON HOT TOPICS IN NETWORKS, HOTNETS 2023(2023)
摘要
Recently, much attention has been devoted to the development of generative network traces and their potential use in supplementing real-world data for a variety of data-driven networking tasks. Yet, the utility of existing synthetic traffic approaches are limited by their low fidelity: low feature granularity, insufficient adherence to task constraints, and subpar class coverage. As effective network tasks are increasingly reliant on raw packet captures, we advocate for a paradigm shift from coarse-grained to fine-grained traffic generation compliant to constraints. We explore this path employing controllable diffusion-based methods. Our preliminary results suggest its effectiveness in generating realistic and fine-grained network traces that mirror the complexity and variety of real network traffic required for accurate service recognition. We further outline the challenges and opportunities of this approach, and discuss a research agenda towards text-to-traffic synthesis.
更多查看译文
关键词
Network traffic,synthesis,diffusion model
AI 理解论文
溯源树
样例
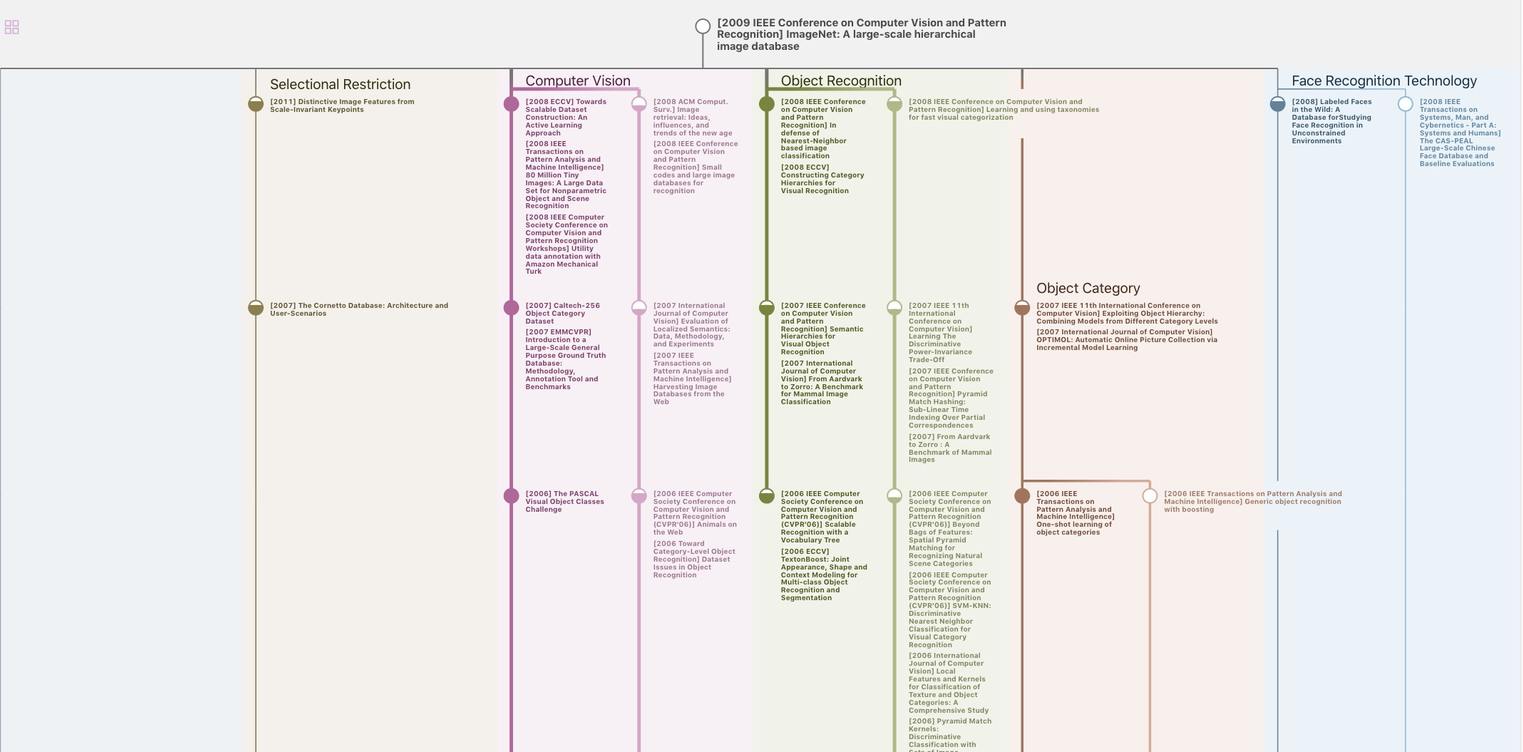
生成溯源树,研究论文发展脉络
Chat Paper
正在生成论文摘要