Secure Sampling for Approximate Multi-party Query Processing.
Proceedings of the ACM on Management of Data(2023)
摘要
We study the problem of random sampling in the secure multi-party computation (MPC) model. In MPC, taking a sample securely must have a cost Ω(n) irrespective to the sample size s. This is in stark contrast with the plaintext setting, where a sample can be taken in O(s) time trivially. Thus, the goal of approximate query processing (AQP) with sublinear costs seems unachievable under MPC. To get around this inherent barrier, in this paper we take a two-stage approach: In the offline stage, we generate a batch of n/s samples with (n) total cost, which can then be consumed to answer queries as they arrive online. Such an approach allows us to achieve an Õ(s) amortized cost per query, similar to the plaintext setting. Based on our secure batch sampling algorithms, we build MASQUE, an MPC-AQP system that achieves sublinear online query costs by running an MPC protocol to evaluate the queries on pre-generated samples. MASQUE achieves the strong security guarantee of the MPC model, i.e., nothing is revealed beyond the query result, which itself can be further protected by (amplified) differential privacy
更多查看译文
关键词
secure sampling,approximate,multi-party
AI 理解论文
溯源树
样例
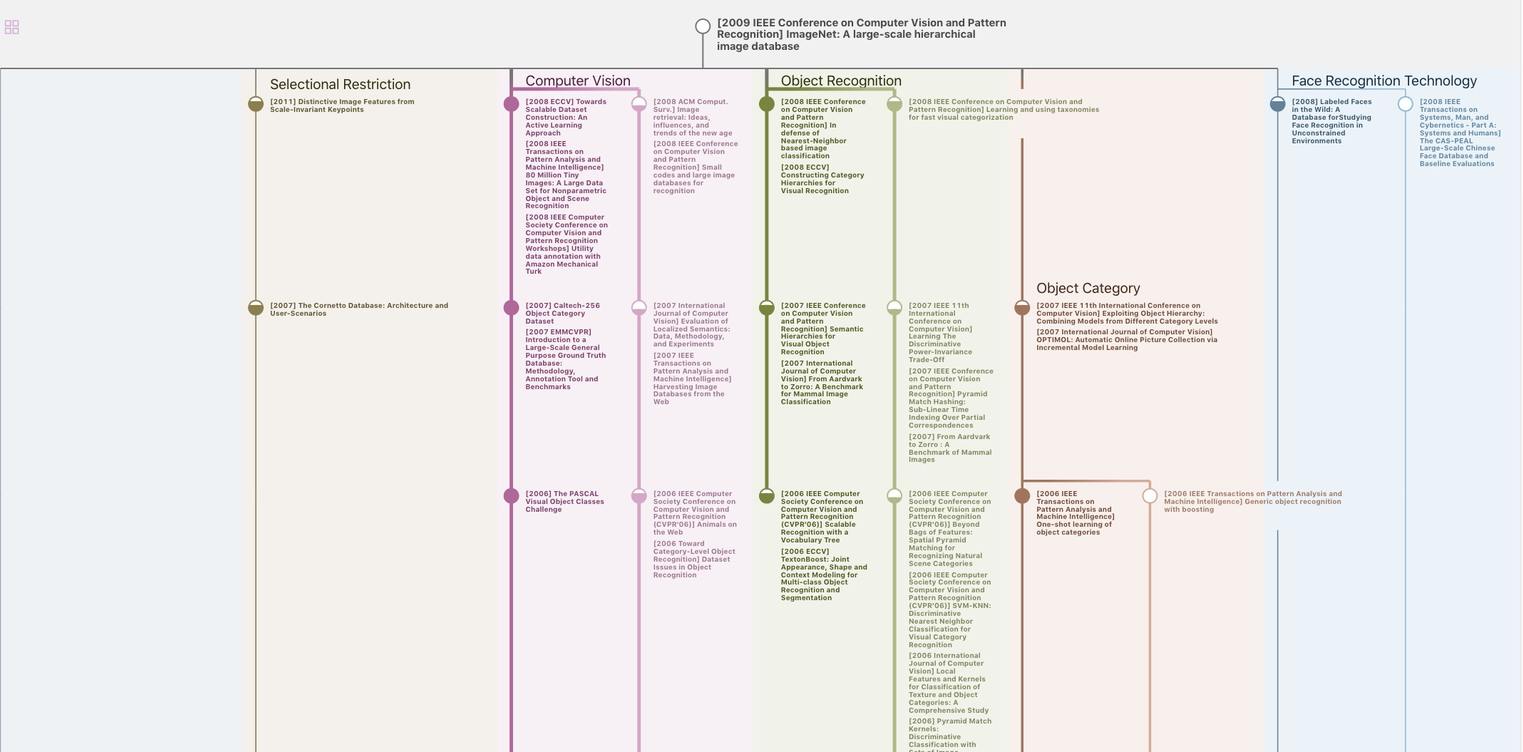
生成溯源树,研究论文发展脉络
Chat Paper
正在生成论文摘要