Gen-Z: Generative Zero-Shot Text Classification with Contextualized\n Label Descriptions
ICLR 2024(2023)
摘要
Language model (LM) prompting--a popular paradigm for solving NLP tasks--has been shown to be susceptible to miscalibration and brittleness to slight prompt variations, caused by its discriminative prompting approach, i.e., predicting the label given the input. To address these issues, we propose Gen-Z--a generative prompting framework for zero-shot text classification. GEN-Z is generative, as it measures the LM likelihood of input text, conditioned on natural language descriptions of labels. The framework is multivariate, as label descriptions allow us to seamlessly integrate additional contextual information about the labels to improve task performance. On various standard classification benchmarks, with six open-source LM families, we show that zero-shot classification with simple contextualization of the data source of the evaluation set consistently outperforms both zero-shot and few-shot baselines while improving robustness to prompt variations. Further, our approach enables personalizing classification in a zero-shot manner by incorporating author, subject, or reader information in the label descriptions.
更多查看译文
关键词
zero-shot classification,prompting,generative classification,label descriptions
AI 理解论文
溯源树
样例
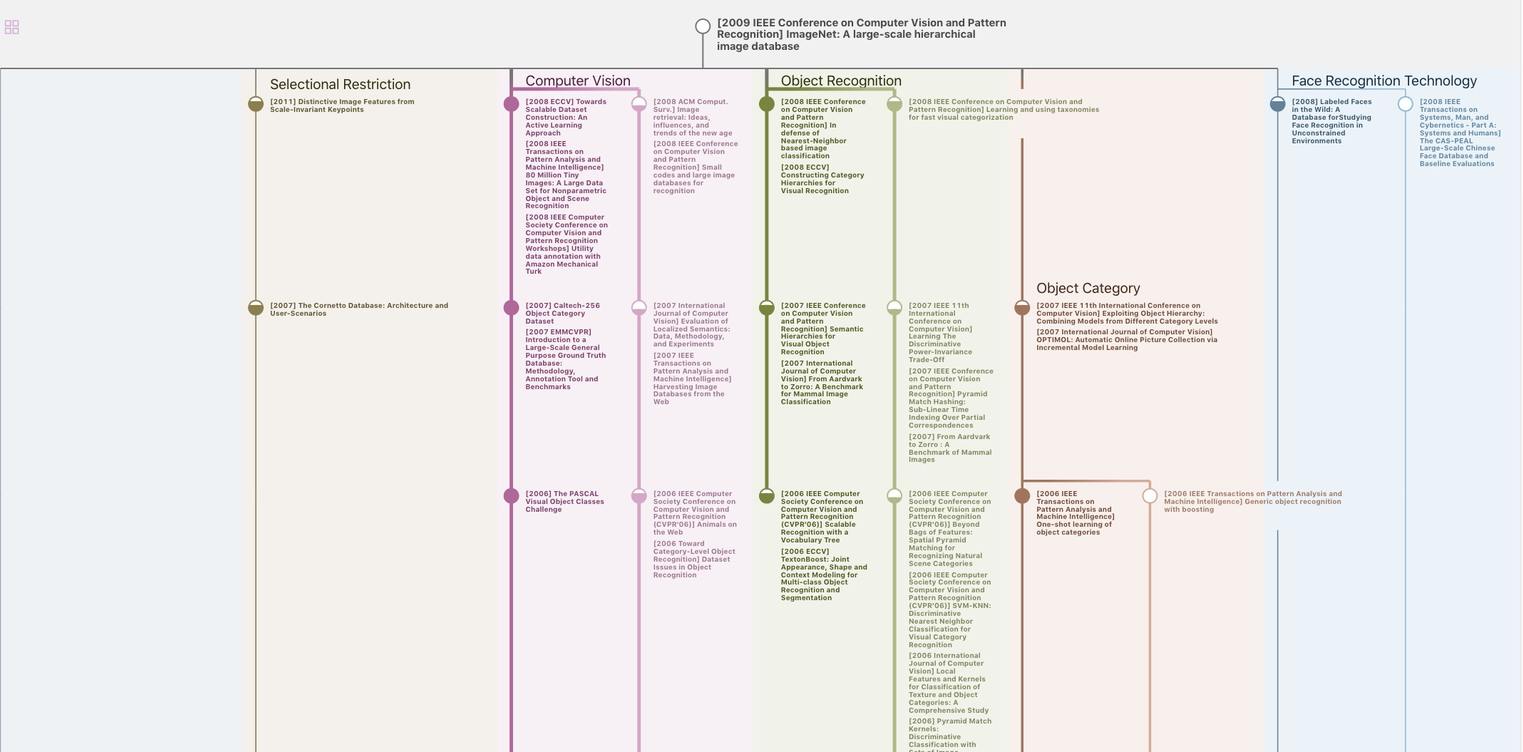
生成溯源树,研究论文发展脉络
Chat Paper
正在生成论文摘要