DeMuX: Data-efficient Multilingual Learning.
CoRR(2023)
摘要
We consider the task of optimally fine-tuning pre-trained multilingual models, given small amounts of unlabelled target data and an annotation budget. In this paper, we introduce DEMUX, a framework that prescribes the exact data-points to label from vast amounts of unlabelled multilingual data, having unknown degrees of overlap with the target set. Unlike most prior works, our end-to-end framework is language-agnostic, accounts for model representations, and supports multilingual target configurations. Our active learning strategies rely upon distance and uncertainty measures to select task-specific neighbors that are most informative to label, given a model. DeMuX outperforms strong baselines in 84% of the test cases, in the zero-shot setting of disjoint source and target language sets (including multilingual target pools), across three models and four tasks. Notably, in low-budget settings (5-100 examples), we observe gains of up to 8-11 F1 points for token-level tasks, and 2-5 F1 for complex tasks. Our code is released here: https://github.com/simran-khanuja/demux.
更多查看译文
AI 理解论文
溯源树
样例
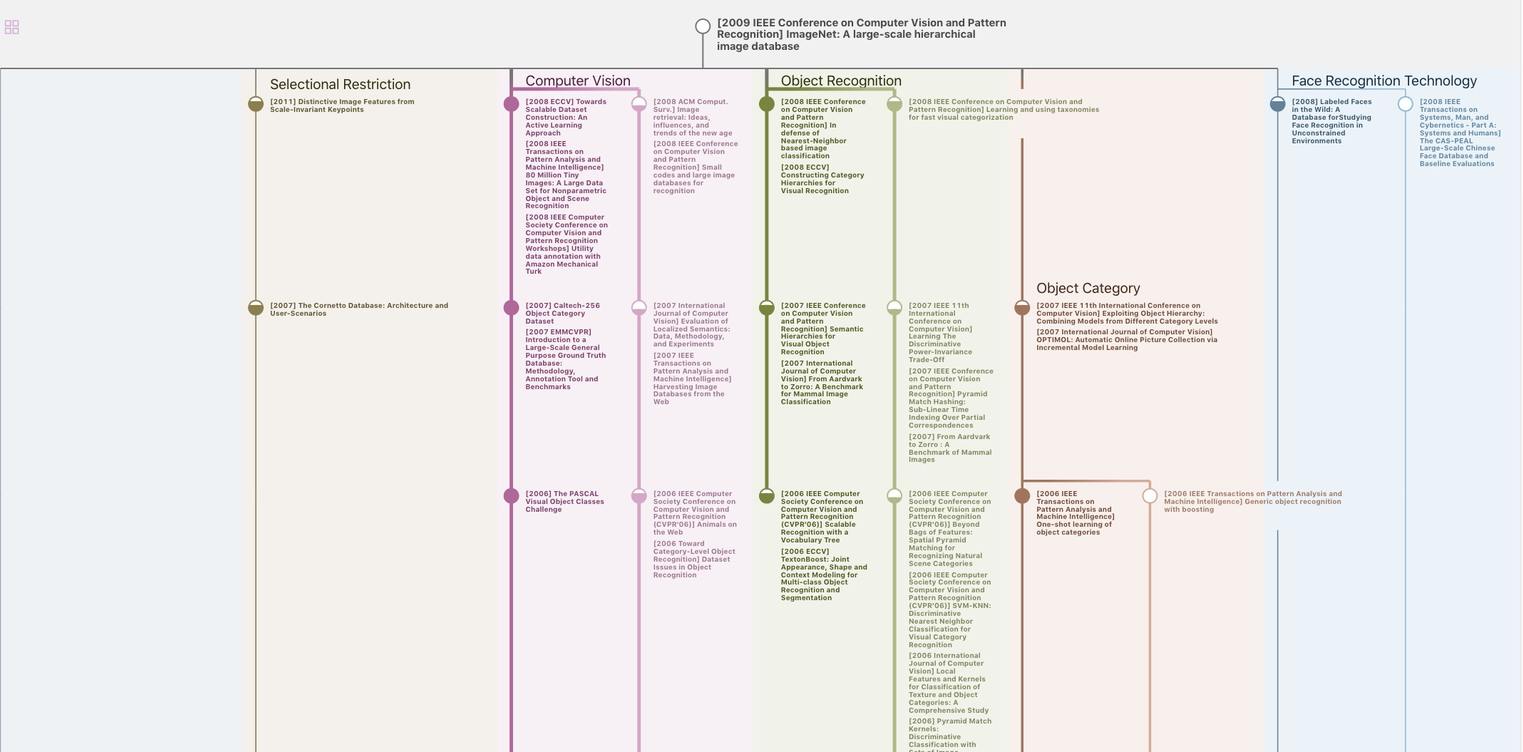
生成溯源树,研究论文发展脉络
Chat Paper
正在生成论文摘要