A Large Deviations Perspective on Policy Gradient Algorithms
arXiv (Cornell University)(2023)
摘要
We derive the first large deviation rate function for the stochastic iterates generated by policy gradient methods with a softmax parametrization and an entropy regularized objective. Leveraging the contraction principle from large deviations theory, we also develop a general recipe for deriving exponential convergence rates for a wide spectrum of other policy parametrizations. This approach unifies several results from the literature and simplifies existing proof techniques.
更多查看译文
AI 理解论文
溯源树
样例
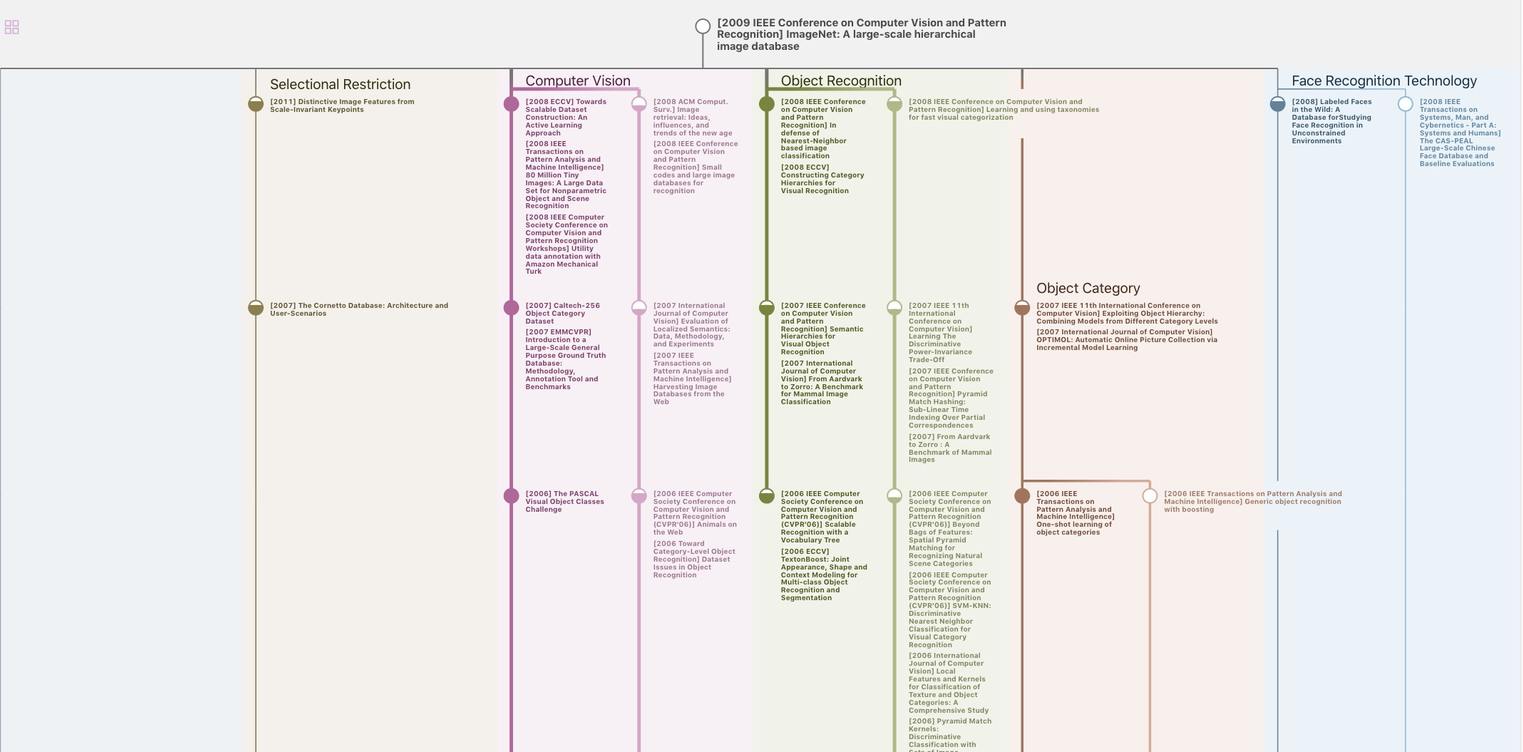
生成溯源树,研究论文发展脉络
Chat Paper
正在生成论文摘要