Exploring Fine-tuning ChatGPT for News Recommendation.
CoRR(2023)
摘要
News recommendation systems (RS) play a pivotal role in the current digital age, shaping how individuals access and engage with information. The fusion of natural language processing (NLP) and RS, spurred by the rise of large language models such as the GPT and T5 series, blurs the boundaries between these domains, making a tendency to treat RS as a language task. ChatGPT, renowned for its user-friendly interface and increasing popularity, has become a prominent choice for a wide range of NLP tasks. While previous studies have explored ChatGPT on recommendation tasks, this study breaks new ground by investigating its fine-tuning capability, particularly within the news domain. In this study, we design two distinct prompts: one designed to treat news RS as the ranking task and another tailored for the rating task. We evaluate ChatGPT's performance in news recommendation by eliciting direct responses through the formulation of these two tasks. More importantly, we unravel the pivotal role of fine-tuning data quality in enhancing ChatGPT's personalized recommendation capabilities, and illustrates its potential in addressing the longstanding challenge of the "cold item" problem in RS. Our experiments, conducted using the Microsoft News dataset (MIND), reveal significant improvements achieved by ChatGPT after fine-tuning, especially in scenarios where a user's topic interests remain consistent, treating news RS as a ranking task. This study illuminates the transformative potential of fine-tuning ChatGPT as a means to advance news RS, offering more effective news consumption experiences.
更多查看译文
关键词
chatgpt,news recommendation,fine-tuning
AI 理解论文
溯源树
样例
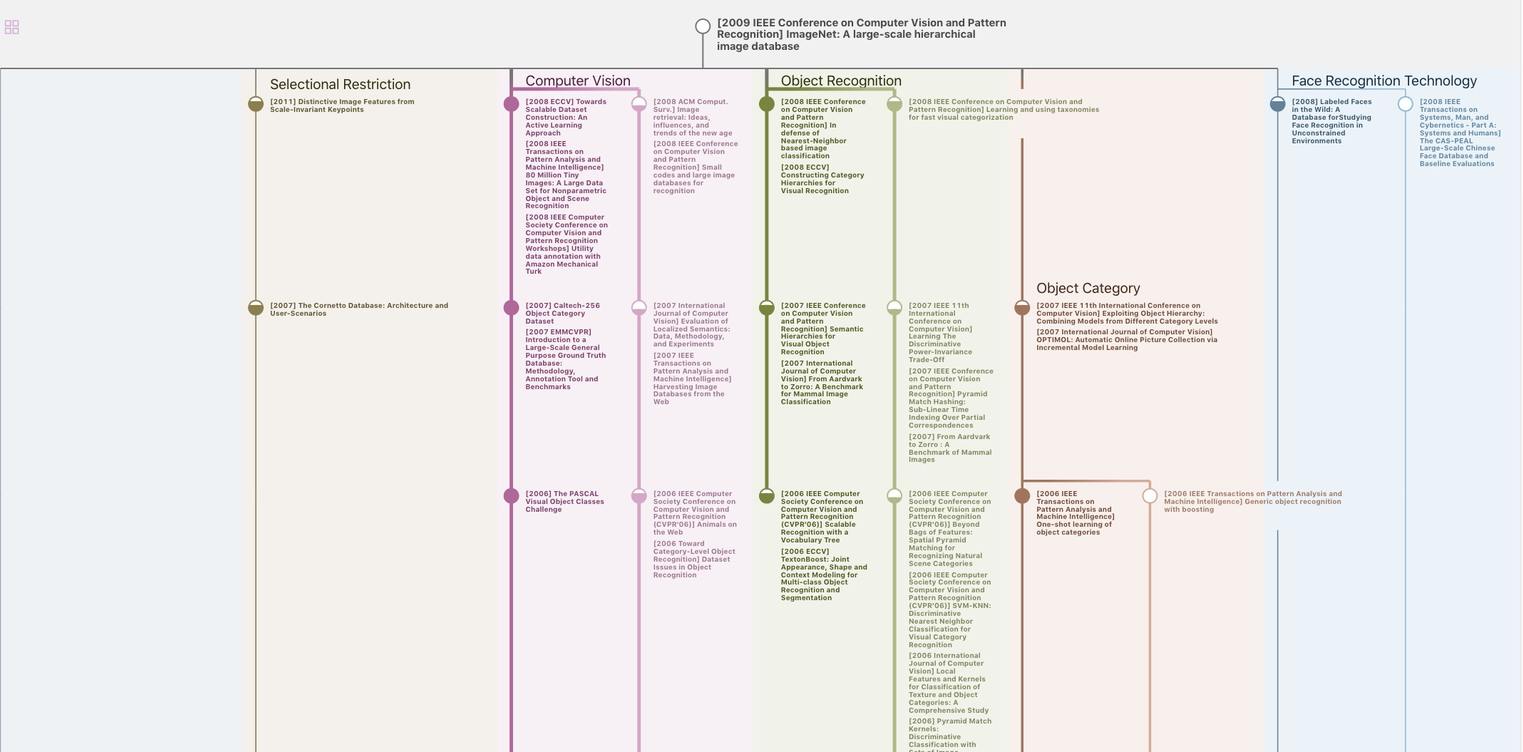
生成溯源树,研究论文发展脉络
Chat Paper
正在生成论文摘要