Accuracy-Constrained Efficiency Optimization and GPU Profiling of CNN Inference for Detecting Drainage Crossing Locations.
SC-W '23: Proceedings of the SC '23 Workshops of The International Conference on High Performance Computing, Network, Storage, and Analysis(2023)
摘要
The accurate and efficient determination of hydrologic connectivity has garnered significant attention from both academic and industrial sectors due to its critical implications for environmental management. While recent studies have leveraged the spatial characteristics of hydrologic features, the use of elevation models for identifying drainage paths can be influenced by flow barriers. To address these challenges, our focus in this study is on detecting drainage crossings through the application of advanced convolutional neural networks (CNNs). In pursuit of this goal, we use neural architecture search to automatically explore CNN models for identifying drainage crossings. Our approach not only attains high accuracy (over 97% for average precision) in object detection but also excels in efficiently inferring correct drainage crossings within a remarkably short time frame (0.268 ms). Furthermore, we perform a detailed profiling of our approach on GPU systems to analyze performance bottlenecks.
更多查看译文
AI 理解论文
溯源树
样例
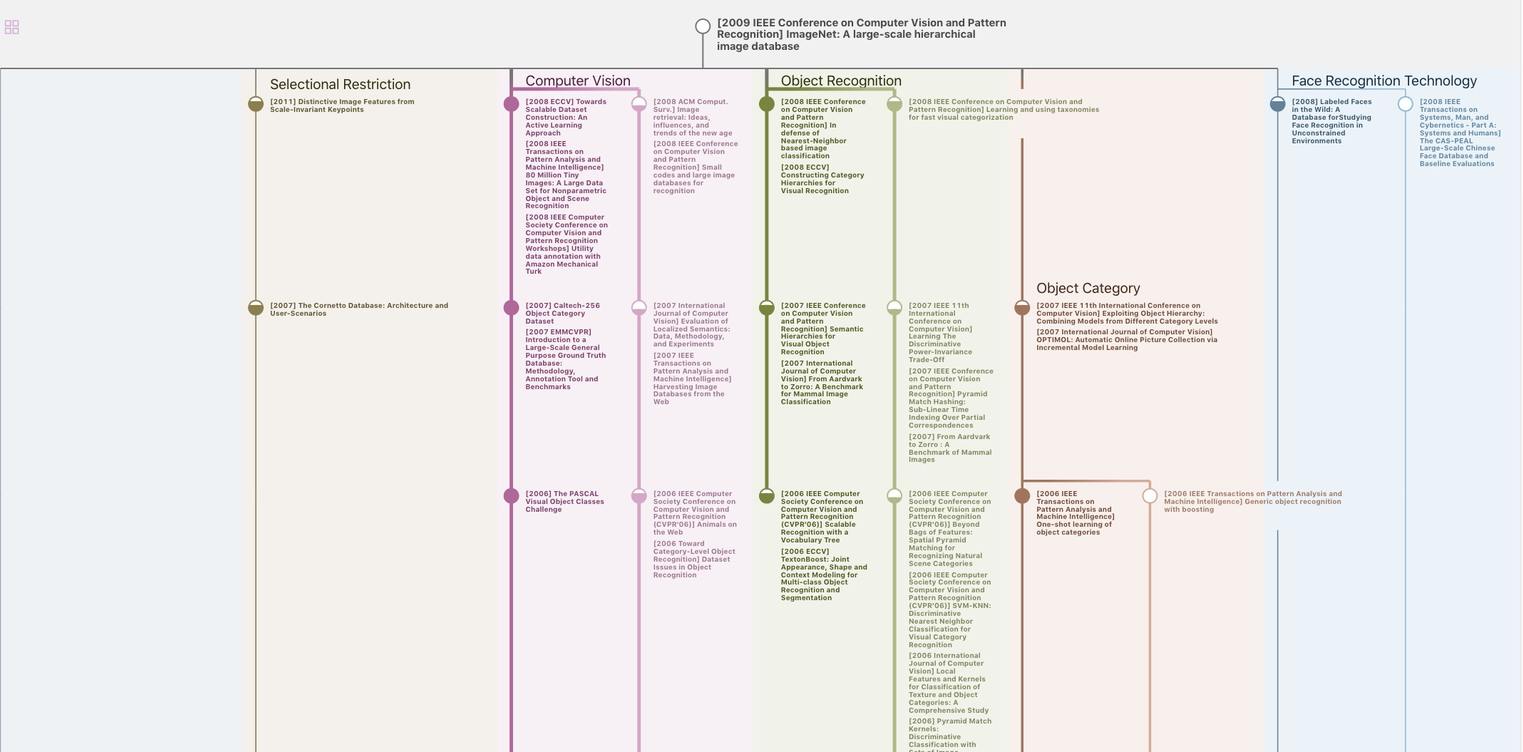
生成溯源树,研究论文发展脉络
Chat Paper
正在生成论文摘要