GBRS: A Unified Granular-Ball Learning Model of Pawlak Rough Set and Neighborhood Rough Set
IEEE TRANSACTIONS ON NEURAL NETWORKS AND LEARNING SYSTEMS(2023)
摘要
Pawlak rough set (PRS) and neighborhood rough set (NRS) are the two most common rough set theoretical models. Although the PRS can use equivalence classes to represent knowledge, it is unable to process continuous data. On the other hand, NRSs, which can process continuous data, rather lose the ability of using equivalence classes to represent knowledge. To remedy this deficit, this article presents a granular-ball rough set (GBRS) based on the granular-ball computing combining the robustness and the adaptability of the granular-ball computing. The GBRS can simultaneously represent both the PRS and the NRS, enabling it not only to be able to deal with continuous data and to use equivalence classes for knowledge representation as well. In addition, we propose an implementation algorithm of the GBRS by introducing the positive region of GBRS into the PRS framework. The experimental results on benchmark datasets demonstrate that the learning accuracy of the GBRS has been significantly improved compared with the PRS and the traditional NRS. The GBRS also outperforms nine popular or the state-of-the-art feature selection methods. We have open-sourced all the source codes of this article at http://www.cquptshuyinxia.com/GBRS.html, https://github.com/syxiaa/GBRS.
更多查看译文
关键词
Attribute reduction,feature selection,granular ball,granular computing,Neighborhood rough set (NRS)
AI 理解论文
溯源树
样例
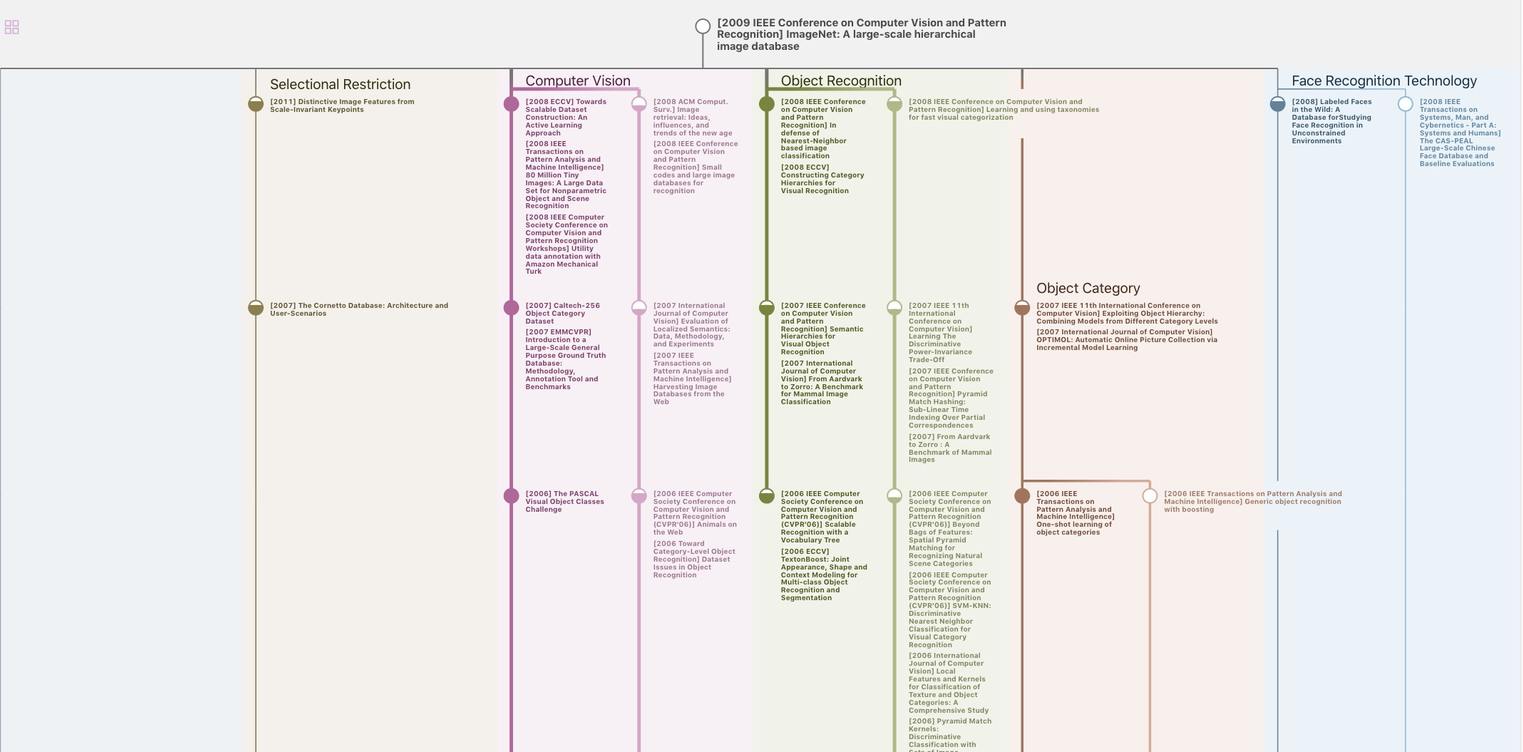
生成溯源树,研究论文发展脉络
Chat Paper
正在生成论文摘要