Adversarial Example Detection and Restoration Defensive Framework for Signal Intelligent Recognition Networks
Applied Sciences(2023)
摘要
Deep learning-based automatic modulation recognition networks are susceptible to adversarial attacks, posing significant performance vulnerabilities. In response, we introduce a defense framework enriched by tailored autoencoder (AE) techniques. Our design features a detection AE that harnesses reconstruction errors and convolutional neural networks to discern deep features, employing thresholds from reconstruction error and Kullback-Leibler divergence to identify adversarial samples and their origin mechanisms. Additionally, a restoration AE with a multi-layered structure effectively restores adversarial samples generated via optimization methods, ensuring accurate classification. Tested rigorously on the RML2016.10a dataset, our framework proves robust against adversarial threats, presenting a versatile defense solution compatible with various deep learning models.
更多查看译文
关键词
adversarial examples,sample detection,sample restoration,autoencoder
AI 理解论文
溯源树
样例
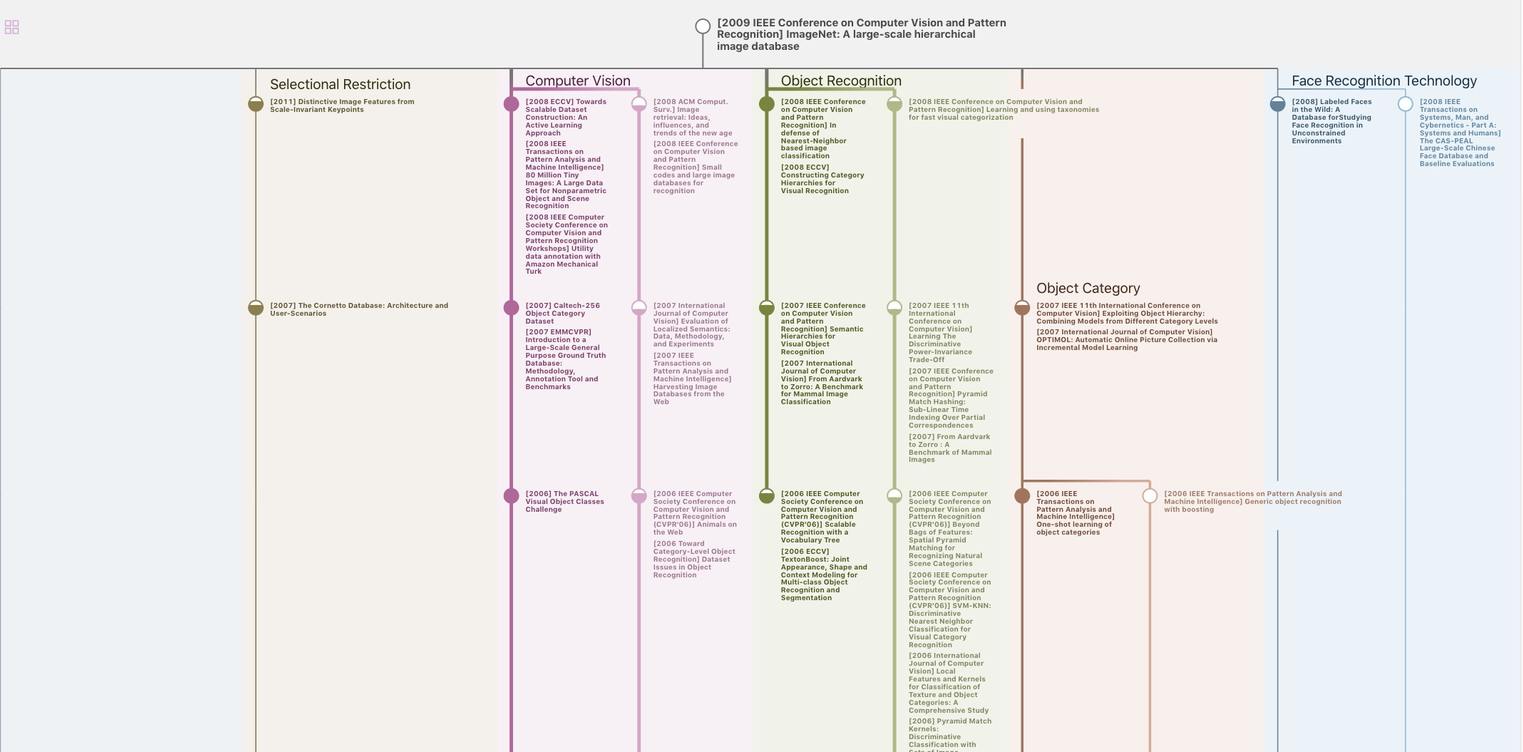
生成溯源树,研究论文发展脉络
Chat Paper
正在生成论文摘要