A Long Time Span-Specific Emitter Identification Method Based on Unsupervised Domain Adaptation
Remote Sensing(2023)
摘要
Specific emitter identification (SEI) is a professional technology to recognize different emitters by measuring the unique features of received signals. It has been widely used in both civilian and military fields. Recently, many SEI methods based on deep learning have been proposed, most of which assume that the training set and testing set have the same data distribution. However, in reality, the testing set is generally used later than the training set and lacks labels. The long time span may change the signal transmission environment and fingerprint features. These changes result in considerable differences in data distribution between the training and testing sets, thereby affecting the recognition and prediction abilities of the model. Therefore, the existing works cannot achieve satisfactory results for a long time span SEI. To address this challenge and obtain stable fingerprints, we transform the long time span SEI problem into a domain adaptive problem and propose an unsupervised domain adaptive method called LTS-SEI. Noteworthily, LTS-SEI uses a multilayer convolutional feature extractor to learn feature knowledge and confronts a domain discriminator to generate domain-invariant shallow fingerprints. The classifier of LTS-SEI applies feature matching to source domain samples and target domain samples to achieve the domain alignment of deep fingerprints. The classifier further reduces the intraclass diversity of deep features to alleviate the misclassification problem of edge samples in the target domain. To confirm the effectiveness and reliability of LTS-SEI, we collect multiple sets of real satellite navigation signals using two antennas with 13 m- and 40 m-large apertures, respectively, and construct two available datasets. Numerous experiments demonstrate that LTS-SEI can considerably increase the recognition accuracy of the long time span SEI and is superior to the other existing methods.
更多查看译文
关键词
specific emitter identification,long time span,unsupervised domain adaptation,source domain,target domain,satellite navigation signal
AI 理解论文
溯源树
样例
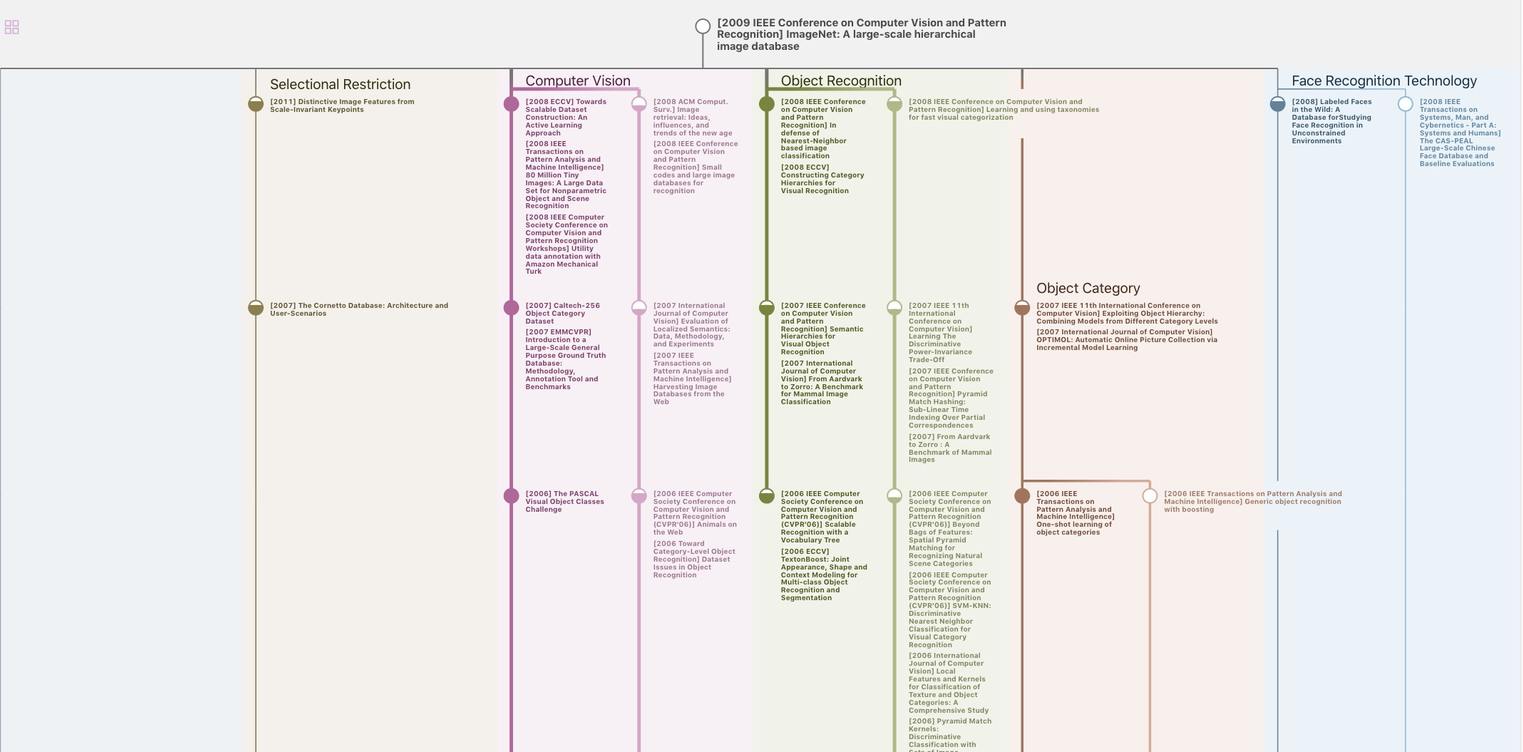
生成溯源树,研究论文发展脉络
Chat Paper
正在生成论文摘要